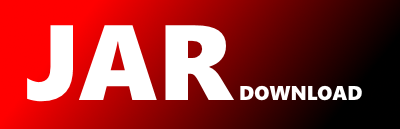
mnist.MNISTSequenceTest.kt Maven / Gradle / Ivy
Go to download
Show more of this group Show more artifacts with this name
Show all versions of simplednn Show documentation
Show all versions of simplednn Show documentation
SimpleDNN is a machine learning lightweight open-source library written in Kotlin whose purpose is to
support the development of feed-forward and recurrent Artificial Neural Networks.
/* Copyright 2016-present The KotlinNLP Authors. All Rights Reserved.
*
* This Source Code Form is subject to the terms of the Mozilla Public
* License, v. 2.0. If a copy of the MPL was not distributed with this
* file, you can obtain one at http://mozilla.org/MPL/2.0/.
* ------------------------------------------------------------------*/
package mnist
import com.kotlinnlp.simplednn.core.functionalities.activations.Softmax
import com.kotlinnlp.simplednn.core.functionalities.activations.Tanh
import com.kotlinnlp.simplednn.core.neuralnetwork.preset.GRUNeuralNetwork
import com.kotlinnlp.simplednn.core.neuralprocessor.recurrent.RecurrentNeuralProcessor
import com.kotlinnlp.simplednn.core.optimizer.ParamsOptimizer
import com.kotlinnlp.simplednn.core.functionalities.updatemethods.adam.ADAMMethod
import com.kotlinnlp.simplednn.dataset.*
import com.kotlinnlp.simplednn.core.functionalities.outputevaluation.ClassificationEvaluation
import com.kotlinnlp.simplednn.helpers.training.SequenceWithFinalOutputTrainingHelper
import com.kotlinnlp.simplednn.helpers.validation.SequenceWithFinalOutputValidationHelper
import com.kotlinnlp.simplednn.core.functionalities.losses.SoftmaxCrossEntropyCalculator
import com.kotlinnlp.simplednn.simplemath.ndarray.dense.DenseNDArray
import mnist.helpers.MNISTSequenceExampleExtractor
import utils.CorpusReader
fun main(args: Array) {
println("Start 'MNIST Sequence Test'")
val dataset = CorpusReader>().read(
corpusPath = Configuration.loadFromFile().mnist_sequence.datasets_paths, // same for validation and test
exampleExtractor = MNISTSequenceExampleExtractor(outputSize = 10),
perLine = true)
MNISTSequenceTest(dataset).start()
println("End.")
}
/**
*
*/
class MNISTSequenceTest(val dataset: Corpus>) {
/**
*
*/
val neuralNetwork = GRUNeuralNetwork(
inputSize = 2,
hiddenSize = 200,
hiddenActivation = Tanh(),
outputSize = 10,
outputActivation = Softmax())
/**
*
*/
fun start() {
this.train()
}
/**
*
*/
fun train() {
println("\n-- TRAINING")
val optimizer = ParamsOptimizer(params = this.neuralNetwork.model, updateMethod = ADAMMethod(stepSize = 0.001))
val neuralProcessor = RecurrentNeuralProcessor(this.neuralNetwork)
val trainingHelper = SequenceWithFinalOutputTrainingHelper(
neuralProcessor = neuralProcessor,
optimizer = optimizer,
lossCalculator = SoftmaxCrossEntropyCalculator(),
verbose = true)
val validationHelper = SequenceWithFinalOutputValidationHelper(
neuralProcessor = neuralProcessor,
outputEvaluationFunction = ClassificationEvaluation())
trainingHelper.train(
trainingExamples = this.dataset.training,
validationExamples = this.dataset.validation,
epochs = 3,
batchSize = 1,
shuffler = Shuffler(enablePseudoRandom = true, seed = 1),
validationHelper = validationHelper)
}
}
© 2015 - 2025 Weber Informatics LLC | Privacy Policy