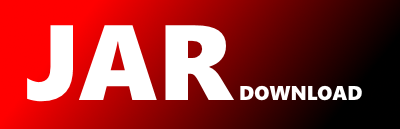
Lib.robot.utils.recommendations.py Maven / Gradle / Ivy
Go to download
Show more of this group Show more artifacts with this name
Show all versions of sikulixapi Show documentation
Show all versions of sikulixapi Show documentation
... for visual testing and automation
# Copyright 2008-2015 Nokia Solutions and Networks
#
# Licensed under the Apache License, Version 2.0 (the "License");
# you may not use this file except in compliance with the License.
# You may obtain a copy of the License at
#
# http://www.apache.org/licenses/LICENSE-2.0
#
# Unless required by applicable law or agreed to in writing, software
# distributed under the License is distributed on an "AS IS" BASIS,
# WITHOUT WARRANTIES OR CONDITIONS OF ANY KIND, either express or implied.
# See the License for the specific language governing permissions and
# limitations under the License.
import difflib
class RecommendationFinder(object):
def __init__(self, normalizer=None):
self.normalizer = normalizer or (lambda x: x)
def find_recommendations(self, name, candidates, max_matches=10):
"""Return a list of close matches to `name` from `candidates`."""
if not name or not candidates:
return []
norm_name = self.normalizer(name)
norm_candidates = self._get_normalized_candidates(candidates)
cutoff = self._calculate_cutoff(norm_name)
norm_matches = difflib.get_close_matches(norm_name,
norm_candidates,
n=max_matches,
cutoff=cutoff)
return self._get_original_candidates(norm_candidates, norm_matches)
@staticmethod
def format_recommendations(msg, recommendations):
"""Add recommendations to the given message.
The recommendation string looks like:
Did you mean:
"""
if recommendations:
msg += " Did you mean:"
for rec in recommendations:
msg += "\n %s" % rec
return msg
def _get_normalized_candidates(self, candidates):
norm_candidates = {}
# sort before normalization for consistent Python/Jython ordering
for cand in sorted(candidates):
norm = self.normalizer(cand)
norm_candidates.setdefault(norm, []).append(cand)
return norm_candidates
def _get_original_candidates(self, norm_candidates, norm_matches):
candidates = []
for norm_match in norm_matches:
candidates.extend(norm_candidates[norm_match])
return candidates
def _calculate_cutoff(self, string, min_cutoff=.5, max_cutoff=.85,
step=.03):
"""Calculate a cutoff depending on string length.
Default values determined by manual tuning until the results
"look right".
"""
cutoff = min_cutoff + len(string) * step
return min(cutoff, max_cutoff)
© 2015 - 2025 Weber Informatics LLC | Privacy Policy