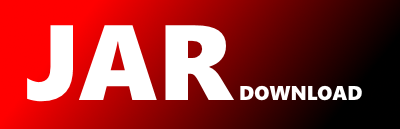
de.julielab.geneexpbase.classification.SVMClassifier Maven / Gradle / Ivy
package de.julielab.geneexpbase.classification;
import cc.mallet.classify.Classification;
import cc.mallet.classify.Classifier;
import cc.mallet.types.Instance;
import cc.mallet.types.InstanceList;
import cc.mallet.types.LabelVector;
import de.julielab.geneexpbase.classification.svm.SVM;
import de.julielab.geneexpbase.classification.svm.SVMModel;
import de.julielab.geneexpbase.classification.svm.SVMTrainOptions;
import libsvm.svm_model;
/**
* This is a MALLET compatible wrapper around LibSVM.
*/
public class SVMClassifier extends Classifier {
private SVMModel model;
@Override
public Classification classify(Instance instance) {
svm_model svmModel = model.svmModel;
if (!model.trainOptions.probability && svmModel.nr_class != 2)
throw new IllegalArgumentException("This method can only be used for two-class problems or when the SVM was trained with probability estimates.");
double[] scores = SVM.predict(instance, model);
if (scores.length == 1) {
// no probabilities; the sole value is "class 1 vs class 2" as hyperplane distance
double[] fullScores = new double[2];
fullScores[0] = scores[0];
// for the other class, the perspective is reversed
fullScores[1] = -1 * scores[0];
scores = fullScores;
}
// Reorder the SVM scores to match the Label indices.
// The SVM labels are just the indices of the MALLET labels.
// Thus we just need to put the values to the position that the SVM label points to.
double[] orderedScores = new double[scores.length];
for (int i = 0; i < scores.length; i++) {
int labelIndex = svmModel.label[i];
orderedScores[labelIndex] = scores[i];
}
return new Classification (instance, this,
new LabelVector(getLabelAlphabet(),
scores));
}
public void train(InstanceList instances, SVMTrainOptions options) {
model = SVM.train(instances, options);
instancePipe = instances.getPipe();
}
}
© 2015 - 2025 Weber Informatics LLC | Privacy Policy