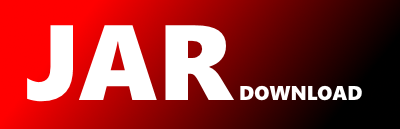
streams.weka.WekaModel Maven / Gradle / Ivy
/**
*
*/
package streams.weka;
import java.io.ByteArrayInputStream;
import java.io.ByteArrayOutputStream;
import java.io.InputStream;
import java.io.ObjectInputStream;
import java.io.ObjectOutputStream;
import java.io.OutputStream;
import java.io.Serializable;
import java.lang.reflect.Method;
import java.text.DecimalFormat;
import java.text.DecimalFormatSymbols;
import java.text.SimpleDateFormat;
import java.util.ArrayList;
import java.util.Collections;
import java.util.Date;
import java.util.LinkedHashMap;
import java.util.List;
import java.util.Map;
import java.util.zip.GZIPInputStream;
import java.util.zip.GZIPOutputStream;
import org.apache.commons.codec.binary.Base64;
import org.slf4j.Logger;
import org.slf4j.LoggerFactory;
import stream.Data;
import stream.runtime.setup.ParameterInjection;
import stream.util.MultiSet;
import stream.util.StringUtils;
import weka.classifiers.Classifier;
import weka.core.Attribute;
import weka.core.DenseInstance;
import weka.core.Instance;
import weka.core.Instances;
/**
* A simple utility class to serialize/deserialize Weka classifiers.
*
* @author Christian Bockermann
*/
public class WekaModel implements Serializable {
/** The unique class ID */
private static final long serialVersionUID = 3777389958517403566L;
static Logger log = LoggerFactory.getLogger(WekaModel.class);
LinkedHashMap meta = new LinkedHashMap();
LinkedHashMap types = new LinkedHashMap();
String label = "@label";
ArrayList classes = new ArrayList();
LinkedHashMap> mapping = new LinkedHashMap>();
transient ArrayList attributes = new ArrayList();
transient Attribute labelAttribute;
transient Instances dataset;
Classifier classifier;
public WekaModel(Classifier classifier) {
this.classifier = classifier;
meta.put("training.date", "");
meta.put("training.user", "");
meta.put("training.instances", "");
meta.put("training.time", "");
meta.put("training.dataset.#attributes", "");
meta.put("training.dataset.attributes", "");
meta.put("training.dataset.label", "");
meta.put("training.dataset.classes", "");
meta.put("training.error", "");
meta.put("training.error.time", "");
}
public void addNominalValue(String key, String value) {
ArrayList values = this.mapping.get(key);
if (values == null) {
log.info("Creating new nominal mapping for '{}'", key);
values = new ArrayList();
mapping.put(key, values);
}
int idx = values.indexOf(value);
if (idx < 0) {
values.add(value);
idx = values.indexOf(value);
log.info("Adding nominal value {} => '{}' for feature '" + key
+ "'", idx, value);
} else {
log.info("Value '{}' already known for feature '{}'", value, key);
}
}
public void setNominalValues(String key, List values) {
ArrayList vals = new ArrayList(values);
log.info("Setting nominal mapping for '{}' to: {}", key, vals);
mapping.put(key, vals);
}
protected boolean isNominal(String key) {
return mapping.containsKey(key);
}
public Classifier classifier() {
return classifier;
}
public Map types() {
return Collections.unmodifiableMap(types);
}
public void train(Instances instances) throws Exception {
MultiSet classDist = new MultiSet();
types = new LinkedHashMap();
for (int i = 0; i < instances.numAttributes(); i++) {
Attribute a = instances.attribute(i);
if (a.isString() || a.isNominal()) {
types.put(a.name(), "java.lang.String");
// mapping.remove(a.name());
for (int v = 0; v < a.numValues(); v++) {
String val = a.value(v);
addNominalValue(a.name(), val);
}
} else {
types.put(a.name(), "java.lang.Double");
}
}
log.info("Built instance type header: {}", types);
labelAttribute = instances.classAttribute();
label = labelAttribute.name();
for (int i = 0; i < labelAttribute.numValues(); i++) {
log.info("Label attribute value: {} => '{}'", i,
labelAttribute.value(i));
List vals = this.mapping.get(label);
log.info(" internal mapping: {} => '{}'", i, vals.get(i));
}
for (int i = 0; i < instances.size(); i++) {
Instance inst = instances.instance(i);
String label = inst.stringValue(labelAttribute);
classDist.add(label);
}
long start = System.currentTimeMillis();
classifier.buildClassifier(instances);
long end = System.currentTimeMillis();
DecimalFormatSymbols dfs = new DecimalFormatSymbols();
dfs.setDecimalSeparator('.');
DecimalFormat df = new DecimalFormat("0.000", dfs);
SimpleDateFormat fmt = new SimpleDateFormat("yyyy-MM-dd HH:mm:ss");
meta.put("training.date", fmt.format(new Date()));
meta.put("training.user", System.getProperty("user.name") + "");
for (String cls : classDist) {
meta.put("training.dataset.class[" + cls + "]",
classDist.count(cls) + "");
}
meta.put("training.time", (end - start) + "ms");
meta.put("training.dataset.#attributes", "" + types.size());
meta.put("training.dataset.attributes",
StringUtils.join(types.keySet(), ","));
meta.put("training.dataset.label", label);
meta.put("training.dataset.classes", "" + mapping.get(label));
start = System.currentTimeMillis();
Double err = 0.0;
Double correct = 0.0;
for (int i = 0; i < instances.size(); i++) {
Instance ex = instances.instance(i);
double cls = ex.classValue();
double pred = classifier.classifyInstance(ex);
if (cls == pred) {
correct += 1.0;
} else {
err += 1.0;
}
}
end = System.currentTimeMillis();
meta.put("training.error", df.format(err / (err + correct)));
meta.put("training.error.time", (end - start) + "ms");
}
public Map info() {
Map info = new LinkedHashMap();
info.put("classifier.class", classifier.getClass().getCanonicalName());
for (Method m : classifier.getClass().getMethods()) {
try {
String name = m.getName();
if (name.startsWith("get") && m.getParameterTypes().length == 0) {
Object prop = m.invoke(classifier, new Object[0]);
name = name.replaceFirst("get", "");
name = name.substring(0, 1).toLowerCase()
+ name.substring(1);
if (prop != null && prop.getClass().isArray()) {
continue;
}
if (!ParameterInjection.isTypeSupported(prop.getClass())) {
continue;
}
info.put("classifier." + name, prop + "");
}
} catch (Exception e) {
}
}
info.putAll(meta);
return Collections.unmodifiableMap(info);
}
public void prepare() throws Exception {
if (attributes != null && !attributes.isEmpty()) {
log.info("Model already preapred.");
return;
}
log.info("Initializing attribute list from training types.");
attributes = new ArrayList();
for (String key : types.keySet()) {
Attribute attribute = null;
if (isNominal(key)) {
List values = mapping.get(key);
attribute = new Attribute(key, values);
log.info(
"Adding nominal attribute '{}' to list of attributes.",
attribute);
} else {
attribute = new Attribute(key);
}
attributes.add(attribute);
if (attribute != null) {
if (attribute.name().equals(label)) {
log.info("Found label attribute '{}'", attribute);
labelAttribute = attribute;
}
}
}
// prepare the dataset and set the class attribute ('label')
//
dataset = new Instances("On-the-Fly", attributes, 1);
dataset.setClass(labelAttribute);
log.info("Model prepared, using {} attributes.", types.size());
}
public Data apply(Data input) throws Exception {
if (attributes == null || attributes.isEmpty()) {
prepare();
}
Instance example = new DenseInstance(attributes.size());
example.setDataset(dataset);
int i = 0;
for (Attribute a : attributes) {
if (a.name().startsWith("@")) {
continue;
}
Serializable value = input.get(a.name());
if (value == null) {
log.warn("Missing value for attribute '{}'", a.name());
continue;
}
if (a.isNumeric()) {
example.setValue(i, new Double(value.toString()));
} else {
log.warn(
"Attribute '{}' will be skipped - String attributes are not supported!",
a.name());
// example.setValue(i, value.toString());
List vals = mapping.get(a.name());
if (vals == null) {
log.warn("No nominal mapping found for attribute '{}'!",
a.name());
} else {
Integer idx = vals.indexOf(value.toString());
example.setValue(a, idx.doubleValue());
}
}
i++;
}
try {
final int pred;
Double conf = null;
// labelAttribute = dataset.classAttribute();
// label = labelAttribute.name();
// for (int j = 0; j < labelAttribute.numValues(); j++) {
// log.info("Label attribute value: {} => '{}'", j,
// labelAttribute.value(j));
// List vals = this.mapping.get(label);
// log.info(" internal mapping: {} => '{}'", j, vals.get(j));
// }
double[] dist = classifier.distributionForInstance(example);
if (dist != null) {
int maxIdx = 0;
for (int c = 0; c < labelAttribute.numValues(); c++) {
String cl = labelAttribute.value(c);
input.put("@prob:" + cl, dist[c]);
if (dist[c] > dist[maxIdx]) {
maxIdx = c;
}
}
pred = maxIdx;
conf = dist[maxIdx];
} else {
pred = (int) classifier.classifyInstance(example);
}
String prediction = labelAttribute.value((int) pred);
log.debug("Prediction is: {}", prediction);
input.put("@prediction", prediction);
if (conf != null) {
input.put("@confidence", conf);
}
} catch (Exception e) {
log.error("Failed to predict example: {}", e.getMessage());
e.printStackTrace();
}
return input;
}
public String toInfoString() {
StringBuffer s = new StringBuffer();
s.append("+--------------------- WekaModel ------------------------\n");
Map info = info();
for (String key : info.keySet()) {
s.append("| " + key + " : " + info.get(key) + "\n");
}
s.append("+--------------------------------------------------------\n");
return s.toString();
}
public static void write(WekaModel c, OutputStream out) throws Exception {
ObjectOutputStream oos = new ObjectOutputStream(out);
oos.writeObject(c);
oos.close();
}
public static WekaModel read(InputStream in) throws Exception {
ObjectInputStream ois = new ObjectInputStream(in);
WekaModel classifier = (WekaModel) ois.readObject();
ois.close();
return classifier;
}
public static String encode(Object o) throws Exception {
ByteArrayOutputStream baos = new ByteArrayOutputStream();
ObjectOutputStream oos = new ObjectOutputStream(new GZIPOutputStream(
baos));
oos.writeObject(o);
oos.close();
return Base64.encodeBase64String(baos.toByteArray());
}
public static Object decode(String str) throws Exception {
byte[] bytes = Base64.decodeBase64(str);
ByteArrayInputStream bais = new ByteArrayInputStream(bytes);
ObjectInputStream ois = new ObjectInputStream(new GZIPInputStream(bais));
Object obj = ois.readObject();
ois.close();
return obj;
}
}
© 2015 - 2025 Weber Informatics LLC | Privacy Policy