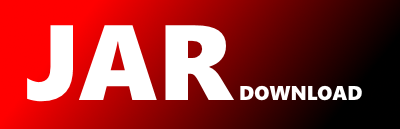
edu.berkeley.nlp.PCFGLA.FeaturizedLexicon Maven / Gradle / Ivy
Go to download
Show more of this group Show more artifacts with this name
Show all versions of berkeleyparser Show documentation
Show all versions of berkeleyparser Show documentation
The Berkeley parser analyzes the grammatical structure of natural language using probabilistic context-free grammars (PCFGs).
The newest version!
package edu.berkeley.nlp.PCFGLA;
import edu.berkeley.nlp.PCFGLA.SimpleLexicon.IntegerIndexer;
import edu.berkeley.nlp.PCFGLA.smoothing.Smoother;
import edu.berkeley.nlp.math.CachingDifferentiableFunction;
import edu.berkeley.nlp.math.DifferentiableFunction;
import edu.berkeley.nlp.math.DoubleArrays;
import edu.berkeley.nlp.math.LBFGSMinimizer;
import edu.berkeley.nlp.math.SloppyMath;
import edu.berkeley.nlp.syntax.StateSet;
import edu.berkeley.nlp.syntax.Tree;
import edu.berkeley.nlp.util.ArrayUtil;
import edu.berkeley.nlp.util.Counter;
import edu.berkeley.nlp.util.Indexer;
import edu.berkeley.nlp.util.Numberer;
import edu.berkeley.nlp.util.Pair;
import edu.berkeley.nlp.util.ScalingTools;
import java.io.Serializable;
import java.util.*;
/**
*
* @author dlwh
*/
public class FeaturizedLexicon implements Lexicon, Serializable {
private double[][][] expectedCounts; // indexed by tag, substate, word
private double[][][] scores; // indexed by tag, substate, word
private double[][] normalizers; // indexed by tag, substate
public int[] wordCounter; // how many times each word occured (global indexed)
private int[][] tagWordCounts; //indexed by tag, word (global indexed)
private int[][] tagWordsWithFeatures; // indexed by tag, index: which tag/word pairs have
// any features at all. (i.e., which ones are allowed?)
/** A trick to allow loading of saved Lexicons even if the version has changed. */
private static final long serialVersionUID = 3L;
/** The number of substates for each state */
public short[] numSubStates;
int numStates;
int nWords;
double threshold;
boolean isLogarithmMode;
boolean useVarDP = false;
private Indexer wordIndexer = new Indexer();
public int[][][][] indexedFeatures; // tag, substate, word, substate list of features
Smoother smoother;
private Featurizer featurizer;
private Indexer featureIndex = new Indexer();
private double[] featureWeights;
private double regularizationConstant = 1.0;
/** Create a blank Lexicon object. Fill it by
* calling tallyStateSetTree for each training tree, then
* calling optimize().
*
* @param numSubStates
*/
@SuppressWarnings("unchecked")
public FeaturizedLexicon(short[] numSubStates, Featurizer featurizer, StateSetTreeList trainTrees) {
this(numSubStates, featurizer);
;
init(trainTrees);
}
public FeaturizedLexicon(short[] numSubStates, Featurizer featurizer) {
this.numSubStates = numSubStates;
this.wordIndexer = new Indexer();
this.numStates = numSubStates.length;
this.isLogarithmMode = false;
this.featurizer = featurizer;
minimizer.setMaxIterations(20);
}
transient private LBFGSMinimizer minimizer = new LBFGSMinimizer();
public LBFGSMinimizer getMinimizer() {
if (minimizer == null) {
minimizer = new LBFGSMinimizer();
}
return minimizer;
}
private double[][][] projectWeightsToScores(double[] weights) {
final double[][][] thetas = new double[numStates][][];
for (int tag = 0; tag < numStates; tag++) {
thetas[tag] = new double[numSubStates[tag]][];
normalizers[tag] = new double[numSubStates[tag]];
final int expLength = expectedCounts[tag].length;
for (int substate = 0; substate < expLength; ++substate) {
thetas[tag][substate] = new double[wordIndexer.size()];
double[] importantThetas = new double[tagWordsWithFeatures[tag].length];
int j = 0;
for (int word: tagWordsWithFeatures[tag]) {
double score = 0.0;
if (indexedFeatures[tag][substate][word].length == 0) {
throw new RuntimeException("Shouldn't be here!");
} else {
for (int f : indexedFeatures[tag][substate][word]) {
score += weights[f];
}
}
thetas[tag][substate][word] = score;
importantThetas[j++] = score;
}
// TODO: updating normalizers here is ugly ugly ugly, but safe enough.
normalizers[tag][substate] = SloppyMath.logAdd(importantThetas);
// the rest are pre-inited to 0.0
for(int word: tagWordsWithFeatures[tag]) {
thetas[tag][substate][word] = Math.exp(thetas[tag][substate][word] - normalizers[tag][substate]);
}
}
}
isLogarithmMode = false;
return thetas;
}
// the m-step objective
private DifferentiableFunction objective(final double[][][] expectedCounts) { // tag, substate
final double[][] eTotals = new double[expectedCounts.length][];
for (int tag = 0; tag < numStates; tag++) {
eTotals[tag] = new double[numSubStates[tag]];
for (int substate = 0; substate < numSubStates[tag]; ++substate) {
for (int word: tagWordsWithFeatures[tag]) {
eTotals[tag][substate] += expectedCounts[tag][substate][word];
}
eTotals[tag][substate] = Math.log(eTotals[tag][substate]);
}
}
return new CachingDifferentiableFunction() {
public int dimension() {
return featureWeights.length;
}
@Override
public double valueAt(double[] x) {
if(isCached(x)) return super.valueAt(x);
double[][][] thetas = projectWeightsToScores(x);
double logProb = 0.0;
for (int tag = 0; tag < numStates; tag++) {
final int expLength = expectedCounts[tag].length;
for (int substate = 0; substate < expLength; ++substate) {
for (int word: tagWordsWithFeatures[tag]) {
if(expectedCounts[tag][substate][word] > 0)
logProb += expectedCounts[tag][substate][word] * Math.log(thetas[tag][substate][word]);
}
}
}
return -logProb + regularizationValue(x);
}
@Override
protected Pair calculate(double[] x) {
double[] gradient = new double[x.length];
double[][][] thetas = projectWeightsToScores(x);
double logProb = 0.0;
for (int tag = 0; tag < numStates; tag++) {
final int expLength = expectedCounts[tag].length;
for (int substate = 0; substate < expLength; ++substate) {
double logTotal = eTotals[tag][substate];
for (int word: tagWordsWithFeatures[tag]) {
double e = expectedCounts[tag][substate][word];
double lT = Math.log(thetas[tag][substate][word]);
double margin = e - Math.exp(logTotal + lT);
if(e > 0)
logProb += expectedCounts[tag][substate][word] * Math.log(thetas[tag][substate][word]);
for (int f : indexedFeatures[tag][substate][word]) {
// we're doing negative gradient because we're maximizing.
gradient[f] -= margin;
}
}
}
}
double[] finalGrad = DoubleArrays.add(gradient, regularizationGradient(x));
double finalLP = -logProb + regularizationValue(x);
return Pair.makePair(finalLP, finalGrad);
}
};
}
private static final double PRIOR_MEAN = -3.0;
private double[] regularizationGradient(double[] x) {
double[] centered = DoubleArrays.add(x, -PRIOR_MEAN);
return DoubleArrays.multiply(centered, regularizationConstant);
}
private double regularizationValue(double[] weights) {
double[] centered = DoubleArrays.add(weights, -PRIOR_MEAN);
return DoubleArrays.innerProduct(centered, centered) * 0.5 * regularizationConstant;
}
// Should be called whenever the number of features or substates changes.
private void refeaturize() {
indexedFeatures = new int[numStates][][][];
featureIndex = new Indexer();
tagWordsWithFeatures = new int[numStates][];
for (int tag = 0; tag < numStates; tag++) {
IntegerIndexer tagIndexer = new IntegerIndexer(wordIndexer.size());
indexedFeatures[tag] = new int[numSubStates[tag]][wordIndexer.size()][];
// index all the features for each word seen with this tag.
for (int globalWordIndex = 0; globalWordIndex < wordIndexer.size(); ++globalWordIndex) {
String word = wordIndexer.getObject(globalWordIndex);
List[] features = featurizer.featurize(word, tag, numSubStates[tag], wordCounter[globalWordIndex], tagWordCounts[tag][globalWordIndex]);
for (int state = 0; state < numSubStates[tag]; ++state) {
int[] indices = new int[features[state].size()];
for (int i = 0; i < indices.length; ++i) {
indices[i] = featureIndex.getIndex(features[state].get(i));
}
indexedFeatures[tag][state][globalWordIndex] = indices;
if(features[state].size() > 0) tagIndexer.add(globalWordIndex);
}
}
tagWordsWithFeatures[tag] = new int[tagIndexer.size()];
for(int j = 0; j < tagIndexer.size(); ++j) {
tagWordsWithFeatures[tag][j] = tagIndexer.get(j);
}
}
if (featureWeights == null || featureWeights.length != featureIndex.size()) {
featureWeights = new double[featureIndex.size()];
}
}
public void optimize() {
refeaturize();
LBFGSMinimizer minimizer = getMinimizer();
DifferentiableFunction objective = objective(expectedCounts);
minimizer.dumpHistory();
//System.out.println("pre norm:" + DoubleArrays.innerProduct(featureWeights, featureWeights));
featureWeights = minimizer.minimize(objective, featureWeights, 1E-5, true);
//System.out.println("post norm1:" + DoubleArrays.innerProduct(featureWeights, featureWeights));
scores = projectWeightsToScores(featureWeights);
}
public double[] score(String word, short tag, int pos, boolean noSmoothing, boolean isSignature) {
StateSet stateSet = new StateSet(tag, (short) 1, word, (short) pos, (short) (pos + 1));
stateSet.wordIndex = -2;
stateSet.sigIndex = -2;
return score(stateSet, tag, noSmoothing, isSignature);
}
public double[] score(StateSet stateSet, short tag, boolean noSmoothing, boolean isSignature) {
double[] res = new double[numSubStates[tag]];
int globalWordIndex = stateSet.wordIndex;
if (globalWordIndex < 1) {
globalWordIndex = stateSet.wordIndex = wordIndexer.indexOf(stateSet.getWord());
}
if (globalWordIndex < 0) { // not rare, so it can't be this tag.
List[] features = featurizer.featurize(stateSet.getWord(), tag, numSubStates[tag], 0, 0);
for (int state = 0; state < numSubStates[tag]; ++state) {
double score = 0.0;
for (String feature : features[state]) {
int index = featureIndex.indexOf(feature);
if (index >= 0) {
score += featureWeights[index];
} else {
score += 100 * PRIOR_MEAN;
}
}
if (isLogarithmMode()) {
res[state] = score - normalizers[tag][state];
} else {
res[state] = Math.exp(score - normalizers[tag][state]);
}
}
} else { // we've scored this word:
for (int i = 0; i < numSubStates[tag]; i++) {
res[i] = scores[tag][i][globalWordIndex];
}
}
return res;
}
// no signatures
public String getSignature(String word, int sentencePosition) {
return word;
}
public boolean isLogarithmMode() {
return isLogarithmMode;
}
public void logarithmMode() {
if (isLogarithmMode) {
return;
}
for (int tag = 0; tag < scores.length; tag++) {
for (int word = 0; word < scores[tag].length; word++) {
for (int substate = 0; substate < scores[tag][word].length; substate++) {
scores[tag][word][substate] = Math.log(scores[tag][word][substate]);
}
}
}
isLogarithmMode = true;
}
/*
* assume that rare words have been replaced by their signature
*/
public void init(StateSetTreeList trainTrees) {
for (Tree tree : trainTrees) {
List words = tree.getYield();
for (StateSet word : words) {
String sig = word.getWord();
wordIndexer.add(sig);
}
}
wordCounter = new int[wordIndexer.size()];
tagWordCounts = new int[numStates][wordIndexer.size()];
for (Tree tree : trainTrees) {
List tags = tree.getPreTerminalYield();
List words = tree.getYield();
int ind = 0;
for (StateSet word : words) {
String sig = word.getWord();
wordCounter[wordIndexer.indexOf(sig)]++;
tagWordCounts[tags.get(ind).getState()][wordIndexer.indexOf(sig)]++;
ind++;
}
}
resetCounts();
nWords = wordIndexer.size();
labelTrees(trainTrees);
}
public void resetCounts() {
expectedCounts = new double[numStates][][];
scores = new double[numStates][][];
normalizers = new double[numStates][];
for (int tag = 0; tag < numStates; tag++) {
expectedCounts[tag] = new double[numSubStates[tag]][wordIndexer.size()];
normalizers[tag] = new double[numSubStates[tag]];
scores[tag] = new double[numSubStates[tag]][wordIndexer.size()];
}
}
public void labelTrees(StateSetTreeList trainTrees) {
for (Tree tree : trainTrees) {
List words = tree.getYield();
for (StateSet word : words) {
word.wordIndex = wordIndexer.indexOf(word.getWord());
word.sigIndex = -1;
}
}
}
public double[] scoreWord(StateSet stateSet, int tag) {
throw new UnsupportedOperationException("Not supported yet.");
}
public double[] scoreSignature(StateSet stateSet, int tag) {
throw new UnsupportedOperationException("Not supported yet.");
}
public void trainTree(Tree trainTree, double randomness, Lexicon oldLexicon, boolean secondHalf, boolean noSmoothing, int unkThreshold) {
// scan data
//for all substates that the word's preterminal tag has
double sentenceScore = 0;
if (randomness == -1) {
sentenceScore = trainTree.getLabel().getIScore(0);
if (sentenceScore == 0) {
System.out.println("Something is wrong with this tree. I will skip it.");
return;
}
}
int sentenceScale = trainTree.getLabel().getIScale();
List words = trainTree.getYield();
List tags = trainTree.getPreTerminalYield();
//for all words in sentence
for (int position = 0; position < words.size(); position++) {
int nSubStates = tags.get(position).numSubStates();
short tag = tags.get(position).getState();
String word = words.get(position).getWord();
int globalWordIndex = wordIndexer.indexOf(word);
double[] oldLexiconScores = null;
if (randomness == -1) {
oldLexiconScores = oldLexicon.score(word, tag, position, noSmoothing, false);
}
StateSet currentState = tags.get(position);
double scale = ScalingTools.calcScaleFactor(currentState.getOScale() - sentenceScale) / sentenceScore;
for (short substate = 0; substate < nSubStates; substate++) {
double weight = 1;
if (randomness == -1) {
//weight by the probability of seeing the tag and word together, given the sentence
if (!Double.isInfinite(scale)) {
weight = currentState.getOScore(substate) * oldLexiconScores[substate] * scale;
} else {
weight = Math.exp(Math.log(ScalingTools.SCALE)
* (currentState.getOScale() - sentenceScale)
- Math.log(sentenceScore)
+ Math.log(currentState.getOScore(substate))
+ Math.log(oldLexiconScores[substate]));
}
} else if (randomness == 0) {
// for the baseline
weight = 1;
} else {
//add a bit of randomness
weight = GrammarTrainer.RANDOM.nextDouble() * randomness / 100.0 + 1.0;
}
if (weight == 0) {
continue;
}
//tally in the tag with the given weight
expectedCounts[tag][substate][globalWordIndex] += weight;
}
}
}
public void setSmoother(Smoother smoother) {
this.smoother = smoother;
}
public FeaturizedLexicon splitAllStates(int[] counts, boolean moreSubstatesThanCounts, int mode) {
FeaturizedLexicon splitLex = this.copyLexicon();
short[] newNumSubStates = new short[numSubStates.length];
newNumSubStates[0] = 1; // never split ROOT
for (short i = 1; i < numSubStates.length; i++) {
newNumSubStates[i] = (short) (numSubStates[i] * 2);
}
newNumSubStates[0] = 1; // never split ROOT
Random random = GrammarTrainer.RANDOM;
splitLex.numSubStates = newNumSubStates;
double[][][] newScores = new double[scores.length][][];
double[][][] newExpCounts = new double[scores.length][][];
for (int tag = 1; tag < expectedCounts.length; tag++) {
newScores[tag] = new double[newNumSubStates[tag]][wordIndexer.size()];
newExpCounts[tag] = new double[newNumSubStates[tag]][wordIndexer.size()];
for (int substate = 0; substate < numSubStates[tag]; substate++) {
for (int word = 0; word < scores[tag][substate].length; word++) {
newScores[tag][2 * substate][word] = newScores[tag][2 * substate + 1][word] = scores[tag][substate][word];
if (mode == 2) {
newScores[tag][2 * substate][word] = newScores[tag][2 * substate + 1][word] = 1.0 + random.nextDouble() / 100.0;
}
}
}
}
splitLex.scores = newScores;
splitLex.expectedCounts = newExpCounts;
return splitLex;
}
/**
* @param mergeThesePairs
* @param mergeWeights
*/
public void mergeStates(boolean[][][] mergeThesePairs, double[][] mergeWeights) {
short[] newNumSubStates = new short[numSubStates.length];
short[][] mapping = new short[numSubStates.length][];
//invariant: if partners[state][substate][0] == substate, it's the 1st one
short[][][] partners = new short[numSubStates.length][][];
Grammar.calculateMergeArrays(mergeThesePairs,newNumSubStates,mapping,partners,numSubStates);
double[][][] newScores = new double[scores.length][][];
for (int tag=1; tag getWordCounter() {
throw new UnsupportedOperationException("Not supported yet.");
}
public void explicitlyComputeScores(int finalLevel) {
throw new UnsupportedOperationException("Not supported yet.");
}
}
© 2015 - 2025 Weber Informatics LLC | Privacy Policy