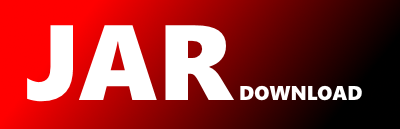
edu.berkeley.nlp.math.OldStochasticObjectiveOptimizer Maven / Gradle / Ivy
Go to download
Show more of this group Show more artifacts with this name
Show all versions of berkeleyparser Show documentation
Show all versions of berkeleyparser Show documentation
The Berkeley parser analyzes the grammatical structure of natural language using probabilistic context-free grammars (PCFGs).
The newest version!
package edu.berkeley.nlp.math;
import edu.berkeley.nlp.mapper.AsynchronousMapper;
import edu.berkeley.nlp.mapper.SimpleMapper;
import edu.berkeley.nlp.util.Logger;
import edu.berkeley.nlp.util.CallbackFunction;
import java.util.Collection;
import java.util.List;
import java.util.ArrayList;
/**
* User: aria42
* Date: Mar 10, 2009
*/
public class OldStochasticObjectiveOptimizer {
Collection items;
List extends ObjectiveItemDifferentiableFunction> itemFns;
Regularizer regularizer;
double initAlpha = 0.5;
double upAlphaMult = 1.1;
double downAlphaMult = 0.5;
Object weightLock = new Object();
double[] weights;
double alpha ;
CallbackFunction iterDoneCallback;
public OldStochasticObjectiveOptimizer(double initAlpha, double upAlphaMult, double downAlphaMult)
{
this.initAlpha = initAlpha;
this.upAlphaMult = upAlphaMult;
this.downAlphaMult = downAlphaMult;
}
public void setIterationCallback(CallbackFunction iterDoneCallback) {
this.iterDoneCallback = iterDoneCallback;
}
class Mapper implements SimpleMapper {
double val = 0.0;
ObjectiveItemDifferentiableFunction itemFn;
Mapper(ObjectiveItemDifferentiableFunction itemFn) {
this.itemFn = itemFn;
}
public void map(I elem) {
double[] localWeights;
synchronized (weightLock) {
localWeights = DoubleArrays.clone(weights);
}
double[] localGrad = new double[dimension()];
itemFn.setWeights(localWeights);
val += itemFn.update(elem,localGrad);
val += regularizer.update(localWeights,localGrad,1.0/items.size());
synchronized (weightLock) {
DoubleArrays.addInPlace(weights,localGrad, -alpha);
}
}
}
private double doIter() {
List mappers = new ArrayList();
for (ObjectiveItemDifferentiableFunction itemFn : itemFns) {
mappers.add(new Mapper(itemFn));
}
AsynchronousMapper.doMapping(items,mappers);
double val = 0.0;
for (Mapper mapper : mappers) {
val += mapper.val;
}
return val;
}
public double[] minimize(double[] initWeights,
int numIters,
Collection items,
List extends ObjectiveItemDifferentiableFunction> itemFns,
Regularizer regularizer)
{
this.items = items;
this.itemFns = itemFns;
this.regularizer = regularizer;
alpha = initAlpha;
weights = DoubleArrays.clone(initWeights);
double lastVal = Double.POSITIVE_INFINITY;
for (int iter = 0; iter < numIters; iter++) {
double val = doIter();
alpha *= (val < lastVal ? upAlphaMult : downAlphaMult);
lastVal = val;
Logger.logs("[StochasticObjectiveOptimizer] Ended Iteration %d with value %.5f",iter+1,val);
if (iterDoneCallback != null) {
iterDoneCallback.callback(iter,weights,val,alpha);
}
}
return weights;
}
public int dimension() {
return itemFns.get(0).dimension();
}
}
© 2015 - 2025 Weber Informatics LLC | Privacy Policy