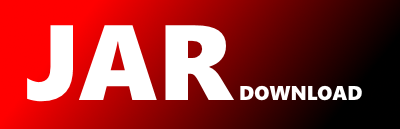
edu.berkeley.nlp.util.NumUtils Maven / Gradle / Ivy
Go to download
Show more of this group Show more artifacts with this name
Show all versions of berkeleyparser Show documentation
Show all versions of berkeleyparser Show documentation
The Berkeley parser analyzes the grammatical structure of natural language using probabilistic context-free grammars (PCFGs).
The newest version!
package edu.berkeley.nlp.util;
import java.util.*;
public class NumUtils {
// This random stuff should be deprecated. DON'T USE IT!
@Deprecated
private static Random random = new Random();
@Deprecated
public static Random getRandom() { return random; }
@Deprecated
public static void setRandom(long seed) { setRandom(new Random(seed)); }
@Deprecated
public static void setRandom(Random random) { NumUtils.random = random; }
@Deprecated
public static double randDouble() { return random.nextDouble(); }
@Deprecated
public static int randInt(int n) { return random.nextInt(n); }
@Deprecated
public static boolean randBernoulli(double p) { return random.nextDouble() < p; }
@Deprecated
public static int randMultinomial(double[] probs, Random random) {
double v = random.nextDouble();
double sum = 0;
for(int i = 0; i < probs.length; i++) {
sum += probs[i];
if(v < sum) return i;
}
throw new RuntimeException(sum + " < " + v);
}
@Deprecated
public static int randMultinomial(double[] probs) {
return randMultinomial(probs, random);
}
public static boolean isFinite(double x) { return !Double.isNaN(x) && !Double.isInfinite(x); }
public static void assertIsFinite(double x) { assert isFinite(x): "Not finite: " + x; }
public static void assertIsFinite(double[] xs) { for(double x : xs) assert isFinite(x): "Not finite: " + Fmt.D(xs); }
public static void assertIsFinite(double[][] xss) { for(double[] xs : xss) for(double x : xs) assert isFinite(x): "Not finite: " + Fmt.D(xss); }
public static boolean isProb(double x) { return x >= 0 && x <= 1 && !Double.isNaN(x); }
public static void assertIsProb(double x) { assert isProb(x): "Not a probability [0, 1]: " + x; }
public static void assertEquals(double x, double y) { assertEquals(x, y, 1e-10); }
public static void assertEquals(double x, double y, double tol) { assert Math.abs(x-y) 0) ListUtils.multMut(x, 1.0/norm);
return x;
}
// If sum is 0, set to uniform
// Return false if we had to set to uniform
public static boolean normalizeForce(double[] data) {
double sum = 0;
for(double x : data) sum += x;
if(sum == 0) {
for(int i = 0; i < data.length; i++) data[i] = 1.0/data.length;
return false;
}
else {
for(int i = 0; i < data.length; i++) data[i] /= sum;
return true;
}
}
public static double[][] transpose(double[][] mat) {
int m = mat.length, n = mat[0].length;
double[][] newMat = new double[n][m];
for(int r = 0; r < m; r++)
for(int c = 0; c < n; c++)
newMat[c][r] = mat[r][c];
return newMat;
}
public static double[][] elementWiseMult(double[][] mat1, double[][] mat2) {
int m = mat1.length, n = mat1[0].length;
double[][] newMat = new double[m][n];
for(int r = 0; r < m; r++)
for(int c = 0; c < n; c++)
newMat[r][c] = mat1[r][c] * mat2[r][c];
return newMat;
}
public static void scalarMult(double[][] mat, double x) {
int m = mat.length, n = mat[0].length;
for(int r = 0; r < m; r++)
for(int c = 0; c < n; c++)
mat[r][c] *= x;
}
public static double[][] copy(double[][] mat) {
int m = mat.length;
double[][] newMat = new double[m][];
for (int r = 0; r < m; r++) {
int n = mat[r].length;
newMat[r] = new double[n];
for (int c = 0; c < n; c++)
newMat[r][c] = mat[r][c];
}
return newMat;
}
public static boolean equals(double x, double y) {
return Math.abs(x-y) < 1e-10;
}
public static boolean equals(double x, double y, double tol) {
return Math.abs(x-y) < tol;
}
public static double round(double x, int numPlaces) {
double scale = Math.pow(10, numPlaces);
return Math.round(x * scale)/scale;
}
public static double[] round(double[] vec, int numPlaces) {
double[] newVec = new double[vec.length];
double scale = Math.pow(10, numPlaces);
for(int i = 0; i < vec.length; i++)
newVec[i] = Math.round(vec[i] * scale)/scale;
return newVec;
}
public static double bound(double x, double lower, double upper) {
if(x < lower) return lower;
if(x > upper) return upper;
return x;
}
public static int bound(int x, int lower, int upper) {
if(x < lower) return lower;
if(x > upper) return upper;
return x;
}
// }
public static double entropy(double[] probs) {
double e = 0;
for(double p : probs) {
if(p > 0) e += -p * Math.log(p);
}
return e;
}
// Suppose we have a joint probability distribution p(X, Y)
// and we want to calculate the conditional entropy
// H(Y | X) = -\sum_x \sum_y p(x, y) \log p(y | x)
// For a fixed x, we input y -> p(X=x, Y=y)
// and get out the contribution to H(Y|X).
// If we sum over all values of X, we get the desired result.
public static double condEntropy(double[] probs) {
double sum = ListUtils.sum(probs); // This is p(X) = \sum_y P(X, Y=y)
double e = 0;
for(double p : probs) {
if(p > 0) e += -p * Math.log(p/sum);
}
assertIsFinite(sum);
return e;
}
public static double condEntropy(double[][] probs) {
double sum = 0;
for(int i = 0; i < probs.length; i++)
sum += condEntropy(probs[i]);
return sum;
}
// Return log(gamma(xx))
private static double[] logGammaCoeff =
{
76.18009172947146, -86.50532032941677,
24.01409824083091, -1.231739572450155,
0.1208650973866179e-2, -0.5395239384953e-5
};
public static double logGamma(double xx)
{
double x, y, tmp, ser;
y = x = xx;
tmp = x + 5.5;
tmp -= (x+0.5) * Math.log(tmp);
ser = 1.000000000190015;
for(int j = 0; j <= 5; j++) ser += logGammaCoeff[j]/++y;
return -tmp + Math.log(2.5066282746310005*ser/x);
}
// Return log factorial(n) = logGamma(n+1)
// Cache small values
private static double[] cachedLogFactorial = null;
private static int numCachedLogFactorial = 1024;
public static double logFactorial(int n) {
if(n < numCachedLogFactorial) {
if(cachedLogFactorial == null) {
cachedLogFactorial = new double[numCachedLogFactorial];
for(int i = 1; i < numCachedLogFactorial; i++) {
cachedLogFactorial[i] = cachedLogFactorial[i-1] + Math.log(i);
}
}
return cachedLogFactorial[n];
}
return logGamma(n+1);
}
public static double logChoose(int n, int k) {
return logFactorial(n) - logFactorial(k) - logFactorial(n-k);
}
/**
* Stolen from Radford Neal's fbm package.
* digamma(x) is defined as (d/dx) log Gamma(x). It is computed here
* using an asymptotic expansion when x>5. For x<=5, the recurrence
* relation digamma(x) = digamma(x+1) - 1/x is used repeatedly. See
* Venables & Ripley, Modern Applied Statistics with S-Plus, pp. 151-152.
* COMPUTE THE DIGAMMA FUNCTION. Returns -inf if the argument is an integer
* less than or equal to zero.
*/
public static double digamma(double x) {
assert x > 0 : x;
double r, f, t;
r = 0;
while (x<=5) {
r -= 1/x;
x += 1;
}
f = 1/(x*x);
t = f*(-1/12.0 + f*(1/120.0 + f*(-1/252.0 + f*(1/240.0 + f*(-1/132.0
+ f*(691/32760.0 + f*(-1/12.0 + f*3617/8160.0)))))));
return r + Math.log(x) - 0.5/x + t;
}
// Return log(exp(a)+exp(b))
private static double logMaxValue = Math.log(Double.MAX_VALUE);
public static double logAdd(double a, double b)
{
if(a > b) {
if(Double.isInfinite(b) || a-b > logMaxValue) return a;
return b+Math.log(1+Math.exp(a-b));
}
else {
if(Double.isInfinite(a) || b-a > logMaxValue) return b;
return a+Math.log(1+Math.exp(b-a));
}
}
// Fast exponential
// http://martin.ankerl.com/2007/10/04/optimized-pow-approximation-for-java-and-c-c/
public static double fastExp(double val) {
final long tmp = (long) (1512775 * val + (1072693248 - 60801));
return Double.longBitsToDouble(tmp << 32);
}
public static double fastLog(double val) {
final double x = (Double.doubleToLongBits(val) >> 32);
return (x - 1072632447) / 1512775;
}
}
© 2015 - 2025 Weber Informatics LLC | Privacy Policy