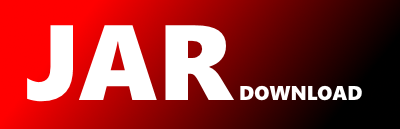
burlap.behavior.singleagent.learning.modellearning.models.TabularModel Maven / Gradle / Ivy
Go to download
Show more of this group Show more artifacts with this name
Show all versions of burlap Show documentation
Show all versions of burlap Show documentation
The Brown-UMBC Reinforcement Learning and Planning (BURLAP) Java code library is for the use and
development of single or multi-agent planning and learning algorithms and domains to accompany them. The library
uses a highly flexible state/observation representation where you define states with your own Java classes, enabling
support for domains that discrete, continuous, relational, or anything else. Planning and learning algorithms range from classic forward search
planning to value-function-based stochastic planning and learning algorithms.
The newest version!
Please wait ...
© 2015 - 2025 Weber Informatics LLC | Privacy Policy