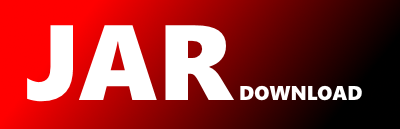
burlap.behavior.stochasticgames.madynamicprogramming.MADynamicProgramming Maven / Gradle / Ivy
Show all versions of burlap Show documentation
package burlap.behavior.stochasticgames.madynamicprogramming;
import burlap.behavior.stochasticgames.madynamicprogramming.AgentQSourceMap.HashMapAgentQSourceMap;
import burlap.behavior.valuefunction.ValueFunction;
import burlap.mdp.core.StateTransitionProb;
import burlap.mdp.core.TerminalFunction;
import burlap.mdp.core.state.State;
import burlap.mdp.stochasticgames.JointAction;
import burlap.mdp.stochasticgames.SGDomain;
import burlap.mdp.stochasticgames.agent.SGAgentType;
import burlap.mdp.stochasticgames.model.FullJointModel;
import burlap.mdp.stochasticgames.model.JointModel;
import burlap.mdp.stochasticgames.model.JointRewardFunction;
import burlap.statehashing.HashableState;
import burlap.statehashing.HashableStateFactory;
import java.util.ArrayList;
import java.util.HashMap;
import java.util.List;
import java.util.Map;
/**
* An abstract value function based planning algorithm base for sequential stochastic games that require the computation of Q-values for each agent for each joint action. Value function
* updates are performed using a Bellman-like backup operator; however, planning for different solution concepts is achieved by providing different backup operators via the
* {@link SGBackupOperator} object.
*
* Note that the agent definitions can only be changed up until planning begins. Once planning has begun, the agent definitions must remain fixed
* for consistency of planning results. If the the client tries to change the agent definitions after planning has already begun, then a runtime exception will
* be thrown.
*
* Since value function planning algorithms compute multi-agent Q-values, this object implements the {@link MultiAgentQSourceProvider} interface.
*
* @author James MacGlashan
*
*/
public abstract class MADynamicProgramming implements MultiAgentQSourceProvider{
/**
* The domain in which planning is to be performed
*/
protected SGDomain domain;
/**
* The agent types
*/
protected List agentDefinitions;
/**
* The joint action model to use in planning.
*/
protected JointModel jointModel;
/**
* The joint reward function
*/
protected JointRewardFunction jointRewardFunction;
/**
* The state terminal function.
*/
protected TerminalFunction terminalFunction;
/**
* The discount factor in [0, 1]
*/
protected double discount;
/**
* The state hashing factory used to query the value function for individual states
*/
protected HashableStateFactory hashingFactory;
/**
* The Q-value initialization function to use.
*/
protected ValueFunction vInit;
/**
* The backup operating defining the solution concept to use.
*/
protected SGBackupOperator backupOperator;
/**
* The Hash map backed multi-agent Q-source in which to store Q-values.
*/
protected HashMapAgentQSourceMap qSources;
/**
* Whether planning has begun or not.
*/
protected boolean planningStarted = false;
/**
* Initializes all the main datstructres of the value function valueFunction
* @param domain the domain in which to perform planning
* @param agentDefinitions the definitions of the agents involved in the planning problem.
* @param jointRewardFunction the joint reward function
* @param terminalFunction the terminal state function
* @param discount the discount factor
* @param hashingFactory the state hashing factorying to use to lookup Q-values for individual states
* @param vInit the value function initialization function to use
* @param backupOperator the solution concept backup operator to use.
*/
public void initMAVF(SGDomain domain, List agentDefinitions, JointRewardFunction jointRewardFunction, TerminalFunction terminalFunction,
double discount, HashableStateFactory hashingFactory, ValueFunction vInit, SGBackupOperator backupOperator){
this.domain = domain;
this.jointModel = domain.getJointActionModel();
this.jointRewardFunction = jointRewardFunction;
this.terminalFunction = terminalFunction;
this.discount = discount;
this.hashingFactory = hashingFactory;
this.vInit = vInit;
this.backupOperator = backupOperator;
this.setAgentDefinitions(agentDefinitions);
}
/**
* Indicates whether planning has begun or not. Once planning has begun, the agent defintions cannot be changed or a runtime exception will be thrown.
* @return true is planning has started; false if it has not.
*/
public boolean hasStartedPlanning(){
return this.planningStarted;
}
/**
* Sets/changes the agent definitions to use in planning. This can only be change up until planning begins, after which a runtime exception will
* be thrown. To check if the planning has already begun, use the {@link #hasStartedPlanning()} method.
* @param agentDefinitions the definitions of agents involve in the planning problem.
*/
public void setAgentDefinitions(List agentDefinitions){
if(this.planningStarted){
throw new RuntimeException("Cannot reset the agent definitions after planning has already started.");
}
if(agentDefinitions == null){
return;
}
if(this.agentDefinitions == agentDefinitions){
return ;
}
this.agentDefinitions = agentDefinitions;
Map hQSources = new HashMap();
for(int i = 0; i < this.agentDefinitions.size(); i++){
QSourceForSingleAgent qs = new BackupBasedQSource(i);
hQSources.put(i, qs);
}
this.qSources = new AgentQSourceMap.HashMapAgentQSourceMap(hQSources);
}
/**
* Calling this method causes planning to be performed from State s.
* @param s the state from which planning is to be performed.
*/
public abstract void planFromState(State s);
@Override
public AgentQSourceMap getQSources(){
return qSources;
}
/**
* Backups the state value function for all agent's value functions in state s.
* @param s the state in which the value functions should be backed up.
* @return the maximum value-function change from this backup.
*/
public double backupAllValueFunctions(State s){
HashableState sh = this.hashingFactory.hashState(s);
double maxChange = Double.NEGATIVE_INFINITY;
for(int i = 0; i < this.agentDefinitions.size(); i++){
BackupBasedQSource qsource = (BackupBasedQSource)this.qSources.agentQSource(i);
double oldVal = qsource.getValue(sh);
double newVal = this.backupOperator.performBackup(s, i, this.agentDefinitions, this.qSources);
maxChange = Math.max(maxChange, Math.abs(newVal-oldVal));
qsource.setValue(sh, newVal);
}
return maxChange;
}
/**
* A class for holding all of the transition dynamic information for a given joint action in a given state. This includes
* state transitions as well as joint rewards. Information is stored as a triple consisting of the {@link JointAction},
* the list of state transtitions ({@link StateTransitionProb} objects), and a list of joint rewards (A map from agent names
* to rewards received).
* @author James MacGlashan
*
*/
public class JointActionTransitions{
public JointAction ja;
public List tps;
public List jrs;
/**
* Generates the transition information for the given state and joint aciton
* @param s the state in which the joint action is applied
* @param ja the joint action applied to the given state
*/
public JointActionTransitions(State s, JointAction ja){
FullJointModel model = (FullJointModel)MADynamicProgramming.this.jointModel;
this.ja = ja;
this.tps = model.stateTransitions(s, ja);
this.jrs = new ArrayList(this.tps.size());
for(StateTransitionProb tp : this.tps){
double[] jr = MADynamicProgramming.this.jointRewardFunction.reward(s, ja, tp.s);
this.jrs.add(jr);
}
}
}
/**
* A {@link QSourceForSingleAgent} implementation which stores a value function for an agent and produces Joint action Q-values
* by marginalizing over the transition dynamics the reward and discounted next state value.
* @author James MacGlashan
*
*/
protected class BackupBasedQSource implements QSourceForSingleAgent{
/**
* The agent for which this value function is assigned.
*/
protected int agentNum;
/**
* The tabular value function
*/
protected Map valueFunction = new HashMap();
/**
* Initializes a value function for the agent of the given name.
* @param agentNum the name of the agent for which the value function corresponds.
*/
public BackupBasedQSource(int agentNum){
this.agentNum = agentNum;
}
@Override
public JAQValue getQValueFor(State s, JointAction ja) {
JointActionTransitions jat = new JointActionTransitions(s, ja);
double sumQ = 0.;
if(!MADynamicProgramming.this.terminalFunction.isTerminal(s)){
for(int i = 0; i < jat.tps.size(); i++){
StateTransitionProb tp = jat.tps.get(i);
double p = tp.p;
HashableState sh = MADynamicProgramming.this.hashingFactory.hashState(tp.s);
double r = jat.jrs.get(i)[this.agentNum];
double vprime = this.getValue(sh);
double contribution = r + MADynamicProgramming.this.discount*vprime;
double weightedContribution = p*contribution;
sumQ += weightedContribution;
}
}
JAQValue q = new JAQValue(s, ja, sumQ);
return q;
}
/**
* Returns the stored state value for hashed state sh, or creates an entry with an initial value corresponding to the {@link MADynamicProgramming}
* instance's value function initialization object and returns that value if the the quried state has never previously been indexed.
* @param sh the state for which the value is to be returned.
* @return the value of the state.
*/
public double getValue(HashableState sh){
Double stored = this.valueFunction.get(sh);
if(stored != null){
return stored;
}
double v = 0.;
if(!MADynamicProgramming.this.terminalFunction.isTerminal(sh.s())){
v = MADynamicProgramming.this.vInit.value(sh.s());
}
this.valueFunction.put(sh, v);
return v;
}
/**
* Sets the value of the state in this objects value function map.
* @param sh the hashed state for which the value is to be set
* @param v the value to set the state to.
*/
public void setValue(HashableState sh, double v){
this.valueFunction.put(sh, v);
}
}
}