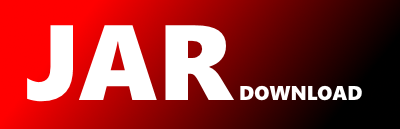
main.java.burlap.behavior.functionapproximation.dense.DenseStateActionLinearVFA Maven / Gradle / Ivy
Go to download
Show more of this group Show more artifacts with this name
Show all versions of burlap Show documentation
Show all versions of burlap Show documentation
The Brown-UMBC Reinforcement Learning and Planning (BURLAP) Java code library is for the use and
development of single or multi-agent planning and learning algorithms and domains to accompany them. The library
uses a highly flexible state/observation representation where you define states with your own Java classes, enabling
support for domains that discrete, continuous, relational, or anything else. Planning and learning algorithms range from classic forward search
planning to value-function-based stochastic planning and learning algorithms.
The newest version!
package burlap.behavior.functionapproximation.dense;
import burlap.behavior.functionapproximation.DifferentiableStateActionValue;
import burlap.behavior.functionapproximation.FunctionGradient;
import burlap.mdp.core.action.Action;
import burlap.mdp.core.state.State;
/**
* @author James MacGlashan.
*/
public class DenseStateActionLinearVFA implements DifferentiableStateActionValue {
protected DenseStateActionFeatures features;
/**
* The function weights when performing Q-value function approximation.
*/
protected double[] stateActionWeights;
/**
* A default weight value for the functions weights.
*/
protected double defaultWeight = 0.0;
protected double[] currentFeatures;
protected double currentValue;
protected FunctionGradient currentGradient = null;
protected State lastState;
public DenseStateActionLinearVFA(DenseStateActionFeatures features, double defaultWeight) {
this.features = features;
this.defaultWeight = defaultWeight;
}
public DenseStateActionLinearVFA(DenseStateActionFeatures features, double[] stateActionWeights, double defaultWeight) {
this.features = features;
this.stateActionWeights = stateActionWeights;
this.defaultWeight = defaultWeight;
}
@Override
public FunctionGradient gradient(State s, Action a) {
double [] features;
if(this.lastState == s){
if(this.currentGradient != null){
return this.currentGradient;
}
features = this.currentFeatures;
}
else{
features = this.features.features(s, a);
}
FunctionGradient gradient = new FunctionGradient.SparseGradient(features.length);
for(int i = 0; i < features.length; i++){
gradient.put(i, features[i]);
}
this.currentGradient = gradient;
this.currentFeatures = features;
this.lastState = s;
return gradient;
}
@Override
public double evaluate(State s, Action a) {
this.currentFeatures = this.features.features(s, a);
if(this.stateActionWeights == null){
this.stateActionWeights = new double[this.currentFeatures.length];
for(int i = 0; i < this.stateActionWeights.length; i++){
this.stateActionWeights[i] = defaultWeight;
}
}
double val = 0;
for(int i = 0; i < this.currentFeatures.length; i++){
val += this.currentFeatures[i] * this.stateActionWeights[i];
}
this.currentValue = val;
this.currentGradient = null;
this.lastState = s;
return this.currentValue;
}
@Override
public int numParameters() {
if(this.stateActionWeights != null){
return this.stateActionWeights.length;
}
return 0;
}
@Override
public double getParameter(int i) {
return this.stateActionWeights[i];
}
@Override
public void setParameter(int i, double p) {
this.stateActionWeights[i] = p;
}
@Override
public void resetParameters() {
this.stateActionWeights = null;
}
@Override
public DenseStateActionLinearVFA copy() {
return new DenseStateActionLinearVFA(features, this.stateActionWeights.clone(), this.defaultWeight);
}
}
© 2015 - 2025 Weber Informatics LLC | Privacy Policy