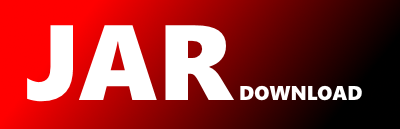
main.java.burlap.behavior.singleagent.learnfromdemo.IRLRequest Maven / Gradle / Ivy
Go to download
Show more of this group Show more artifacts with this name
Show all versions of burlap Show documentation
Show all versions of burlap Show documentation
The Brown-UMBC Reinforcement Learning and Planning (BURLAP) Java code library is for the use and
development of single or multi-agent planning and learning algorithms and domains to accompany them. The library
uses a highly flexible state/observation representation where you define states with your own Java classes, enabling
support for domains that discrete, continuous, relational, or anything else. Planning and learning algorithms range from classic forward search
planning to value-function-based stochastic planning and learning algorithms.
The newest version!
package burlap.behavior.singleagent.learnfromdemo;
import burlap.behavior.singleagent.Episode;
import burlap.behavior.singleagent.planning.Planner;
import burlap.mdp.singleagent.SADomain;
import java.util.ArrayList;
import java.util.List;
/**
* A data structure for setting the common parameters necessary for an IRL algorithm.
*
* @author Stephen Brawner and Mark Ho
*/
public class IRLRequest {
/**
* The domain in which IRL is to be performed
*/
protected SADomain domain;
/**
* The planning algorithm used to compute the policy for a given reward function
*/
protected Planner planner;
/**
* The input trajectories/episodes that are to be modeled.
*/
protected List expertEpisodes;
/**
* The discount factor of the problem
*/
protected double gamma = 0.99;
/**
* Values will not be initialized. You must use the setters for the domain, valueFunction, and expert episodes.
*/
public IRLRequest(){
}
/**
* Initializes. Discount factor will be defaulted to 0.99, which can optionally be changed with a setter.
* @param domain the domain in which IRL is to be performed
* @param planner the planning algorithm the IRL algorithm will invoke.
* @param expertEpisodes the example expert trajectories/episodes.
*/
public IRLRequest(SADomain domain, Planner planner, List expertEpisodes){
this.setDomain(domain);
this.setPlanner(planner);
this.setExpertEpisodes(expertEpisodes);
}
/**
* Returns true if this request object has valid data members set; false otherwise.
* @return true if this request object has valid data members set; false otherwise.
*/
public boolean isValid() {
if(this.domain == null){
return false;
}
if (this.planner == null) {
return false;
}
if (this.expertEpisodes == null || this.expertEpisodes.isEmpty()) {
return false;
}
if (this.gamma > 1 || this.gamma < 0 || Double.isNaN(this.gamma)) {
return false;
}
return true;
}
public void setDomain(SADomain d) {
this.domain = d;
}
public void setPlanner(Planner p) {
this.planner = p;
}
public void setExpertEpisodes(List episodeList) {
this.expertEpisodes = new ArrayList(episodeList);
}
public void setGamma(double gamma) { this.gamma = gamma;}
public SADomain getDomain() {return this.domain;}
public Planner getPlanner() {return this.planner;}
public double getGamma() {
return gamma;
}
public List getExpertEpisodes() {
return expertEpisodes;
}
}
© 2015 - 2024 Weber Informatics LLC | Privacy Policy