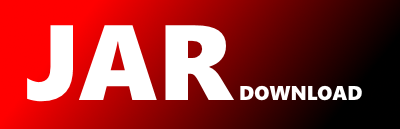
ngmf.util.cosu.BranchAndBound Maven / Gradle / Ivy
Go to download
Show more of this group Show more artifacts with this name
Show all versions of oms Show documentation
Show all versions of oms Show documentation
Object Modeling System (OMS) is a pure Java object-oriented framework.
OMS v3.+ is a highly interoperable and lightweight modeling framework for component-based model and simulation development on multiple platforms.
/*
* $Id: BranchAndBound.java 50798ee5e25c 2013-01-09 [email protected] $
*
* This file is part of the Object Modeling System (OMS),
* 2007-2012, Olaf David and others, Colorado State University.
*
* OMS is free software: you can redistribute it and/or modify
* it under the terms of the GNU Lesser General Public License as published by
* the Free Software Foundation, version 2.1.
*
* OMS is distributed in the hope that it will be useful,
* but WITHOUT ANY WARRANTY; without even the implied warranty of
* MERCHANTABILITY or FITNESS FOR A PARTICULAR PURPOSE. See the
* GNU Lesser General Public License for more details.
*
* You should have received a copy of the GNU Lesser General Public License
* along with OMS. If not, see .
*/
package ngmf.util.cosu;
import java.util.ArrayList;
import java.util.Random;
import java.util.Stack;
import java.util.Vector;
import oms3.util.Statistics;
/**
*
* @author od
*/
public class BranchAndBound {
class HyperCube {
protected Sample a;
protected Sample b;
protected Sample midPoint;
protected double L;
protected ArrayList InCubeSamples;
public double goodOneFactor;
protected HyperCube parent;
protected double highestLowBound;
HyperCube(Sample a, Sample b, Sample midPoint, HyperCube parent) {
this.a = a;
this.b = b;
this.midPoint = midPoint;
this.parent = parent;
this.InCubeSamples = new ArrayList();
InCubeSamples.add(a);
InCubeSamples.add(b);
InCubeSamples.add(midPoint);
this.L = ApproxL(a, b, this);
if (parent != null) {
highestLowBound = Math.max(Math.max(-1000000000000.0,
Math.max(a.fx, b.fx) - VectorNorm2(VectorMul(VectorSub(b.x, a.x), L))),
midPoint.fx - VectorNorm2(VectorMul(VectorSub(b.x, a.x), L)) / 2.0);
}
double minimum = Math.min(Math.min(a.fx, b.fx), midPoint.fx);
if (highestLowBound > minimum) {
highestLowBound = minimum;
}
goodOneFactor = ((midPoint.fx - a.fx) + (midPoint.fx - b.fx));
}
public void addCubeSample(Sample x) {
this.InCubeSamples.add(x);
}
double CalculateLForTarget(double target) {
//look which L value can realize highestLowBound
double L_theo1 = (Math.max(a.fx, b.fx) - target) / VectorNorm(VectorSub(b.x, a.x));
double L_theo2 = 2.0 * (midPoint.fx - target) / VectorNorm(VectorSub(b.x, a.x));
return Math.min(L_theo1, L_theo2);
}
double bound() {
return highestLowBound;
}
public String compactDescriptionString() {
String s = "";
s += a.x[0] + "\t";
s += a.x[1] + "\t";
s += highestLowBound + "\n";
s += b.x[0] + "\t";
s += a.x[1] + "\t";
s += highestLowBound + "\n";
s += b.x[0] + "\t";
s += b.x[1] + "\t";
s += highestLowBound + "\n";
s += a.x[0] + "\t";
s += b.x[1] + "\t";
s += highestLowBound + "\n";
return s;
}
public String toString() {
return "a:" + a.toString() + "\nb:" + b.toString() + "\nmidPoint:" + midPoint.toString() + "\nbound:" + highestLowBound;
}
}
double[] VectorAdd(double[] a, double[] b) {
double sum[] = new double[a.length];
for (int i = 0; i < a.length; i++) {
sum[i] = a[i] + b[i];
}
return sum;
}
double[] VectorAdd(double[] a, double b) {
double sum[] = new double[a.length];
for (int i = 0; i < a.length; i++) {
sum[i] = a[i] + b;
}
return sum;
}
double[] VectorSub(double[] a, double[] b) {
double sum[] = new double[a.length];
for (int i = 0; i < a.length; i++) {
sum[i] = a[i] - b[i];
}
return sum;
}
double[] VectorMul(double[] a, double mul) {
double result[] = new double[a.length];
for (int i = 0; i < a.length; i++) {
result[i] = a[i] * mul;
}
return result;
}
double[] VectorMul(double[] a, double[] mul) {
double result[] = new double[a.length];
for (int i = 0; i < a.length; i++) {
result[i] = a[i] * mul[i];
}
return result;
}
boolean VectorLessEq(double[] x, double[] y) {
for (int i = 0; i < x.length; i++) {
if (x[i] > y[i] - 0.000001) {
return false;
}
}
return true;
}
double VectorNorm(double[] v) {
double sum = 0;
for (int i = 0; i < v.length; i++) {
sum += Math.abs(v[i]);//*v[i];
}
return sum;//Math.sqrt(sum);
}
double VectorNorm2(double[] v) {
double sum = 0;
for (int i = 0; i < v.length; i++) {
sum += (v[i] * v[i]);//*v[i];
}
return Math.sqrt(sum);//Math.sqrt(sum);
}
double VectorMaxNorm(double[] v) {
double sum = 0;
for (int i = 0; i < v.length; i++) {
sum = Math.max(Math.abs(v[i]), sum);//*v[i];
}
return sum;//Math.sqrt(sum);
}
double VectorMin(double[] v) {
double sum = 0;
for (int i = 0; i < v.length; i++) {
sum = Math.min(v[i], sum);//*v[i];
}
return sum;//Math.sqrt(sum);
}
int getMin(Vector Q) {
double min = Double.MAX_VALUE;
int index = 0;
for (int i = 0; i < Q.size(); i++) {
if (Q.get(i).fx < min) {
min = Q.get(i).fx;
index = i;
}
}
return index;
}
double test = 0;
double[] lowBound;
double[] upBound;
int mode;
int currentSampleCount;
double effValue;
int maxn;
int n;
double[] parameters;
Vector sampleList = new Vector();
Random generator = new Random();
Sample getSample(double[] x) {
Sample s = new Sample(x, funct(x));
sampleList.add(s);
return s;
}
double funct(double x[]) {
//RefreshDataHandles();
for (int j = 0; j < parameters.length; j++) {
try {
parameters[j] = x[j];
} catch (Exception e) {
throw new RuntimeException("Error! Parameter No. " + j + " wasn^t found" + e.toString());
}
}
// singleRun();
double value = effValue;
//sometimes its a bad idea to calculate with NaN or Infty
double bigNumber = 10000000;
effValue = Math.max(effValue, -bigNumber);
effValue = Math.min(effValue, bigNumber);
if (Double.isNaN(effValue)) {
effValue = -bigNumber;
}
currentSampleCount++;
switch (mode) {
case Statistics.MINIMIZATION:
return value;
case Statistics.MAXIMIZATION:
return -value;
case Statistics.ABSMINIMIZATION:
return Math.abs(value);
case Statistics.ABSMAXIMIZATION:
return -Math.abs(value);
default:
return 0.0;
}
}
double[] randomSample() {
double[] sample = new double[n];
for (int i = 0; i < n; i++) {
sample[i] = (lowBound[i] + generator.nextDouble() * (upBound[i] - lowBound[i]));
}
return sample;
}
double ApproxL(Sample a, Sample b, HyperCube myCube) {
lowBound = a.x;
upBound = b.x;
ArrayList list = myCube.InCubeSamples;
if (myCube.parent != null) {
for (int i = 0; i < myCube.parent.InCubeSamples.size(); i++) {
Sample x = myCube.parent.InCubeSamples.get(i);
if (VectorLessEq(a.x, x.x) && VectorLessEq(x.x, b.x) && !list.contains(x)) {
boolean contains = false;
for (int j = 0; j < list.size(); j++) {
if (VectorNorm(VectorSub(list.get(j).x, x.x)) < 0.0001) {
contains = true;
break;
}
}
if (!contains) {
myCube.addCubeSample(x);
}
}
}
}
while (list.size() < 3 * n + 1) {
Sample rnd_point = getSample(randomSample());
if (rnd_point.fx < a.fx && rnd_point.fx < b.fx) {
test = a.fx - rnd_point.fx + b.fx - rnd_point.fx;
}
myCube.addCubeSample(rnd_point);
}
//single value L
double variance = 0;
double mean = 0;
//Stack tmp = new Stack();
double sL = 0;
double size = (list.size() - 1) * (list.size()) / 2.0;
for (int i = 0; i < list.size(); i++) {
for (int j = i + 1; j < list.size(); j++) {
double d = VectorNorm2(VectorSub(list.get(i).x, list.get(j).x));
sL = Math.max(Math.abs((list.get(i).fx - list.get(j).fx) / d), sL);
mean += sL;
//tmp.push(new Double(sL));
}
}
mean /= size;
return sL;
}
public void run() {
Vector Q = new Vector();
Vector cubes = new Vector();
int xCount = 0;
final double epsilon1 = 0.1; //app-error
double gamma, myR, my;
int k = 1;
System.out.println("***************************");
System.out.println("****start optimization ****");
System.out.println("***************************");
Sample a = getSample(lowBound);
Sample b = getSample(upBound);
//add upperleft und lowerright corner of cube
Q.add(a);
Q.add(b);
//midpoint xr
double xR_tmp[] = VectorMul(VectorAdd(lowBound, upBound), 0.5);
Sample xR = getSample(xR_tmp);
Q.add(xR);
//gamma holds minimum of samples
int IndexWithMinimum = getMin(Q);
Sample v = Q.get(IndexWithMinimum);
gamma = v.fx;
//calculate a lower approximation my
HyperCube R = new HyperCube(a, b, xR, null);
cubes.add(R);
double L = R.L;
myR = Math.max(Math.max(a.fx, b.fx) - VectorNorm(VectorMul(VectorSub(a.x, b.x), L)),
xR.fx - (VectorNorm(VectorMul(VectorSub(a.x, b.x), L)) / 2.0));
my = myR;
Stack queue = new Stack();
queue.push(R);
while (true) {
R = queue.pop();
a = R.a;
b = R.b;
my = R.highestLowBound;
//System.out.println("Processing next cube:\nR:" + R.toString() + "\nMinimum:" + gamma + "\nk:" + k + "\nSampleCount:" + currentSampleCount);
//SaveCubes(cubes,"cubedump" + xCount + ".dat");
if (maxn > 0) {
if (sampleList.size() >= maxn) {
break;
}
}
//current minimum and lower approximation are close
if (gamma - my < epsilon1) {
//break;
}
int sel_j = 0;
double max = 0;
for (int i = 0; i < n; i++) {
if (b.x[i] - a.x[i] > max) {
max = b.x[i] - a.x[i];
sel_j = i;
}
}
Sample a1 = a;
double b1_tmp[] = new double[n];
double a2_tmp[] = new double[n];
Sample b2 = b;
for (int i = 0; i < n; i++) {
if (i == sel_j) {
b1_tmp[i] = (a.x[i] + b.x[i]) / 2.0;
a2_tmp[i] = (a.x[i] + b.x[i]) / 2.0;
} else {
b1_tmp[i] = b.x[i];
a2_tmp[i] = a.x[i];
}
}
Sample b1 = getSample(b1_tmp);
Sample a2 = getSample(a2_tmp);
double xR1_tmp[] = VectorMul(VectorAdd(a1.x, b1.x), 0.5);
double xR2_tmp[] = VectorMul(VectorAdd(a2.x, b2.x), 0.5);
Sample xR1 = getSample(xR1_tmp);
Sample xR2 = getSample(xR2_tmp);
//approx L
HyperCube R1 = new HyperCube(a1, b1, xR1, R);
double L1 = R1.L;
double tmp1 = test;
HyperCube R2 = new HyperCube(a2, b2, xR2, R);
double L2 = R2.L;
double tmp2 = test;
Q.clear();
Q.add(v);
Q.add(b1);
Q.add(xR1);
Q.add(a2);
Q.add(xR2);
IndexWithMinimum = getMin(Q);
v = Q.get(IndexWithMinimum);
gamma = v.fx;
if (R1.goodOneFactor < tmp1) {
R1.goodOneFactor = tmp1;
}
if (R2.goodOneFactor < tmp2) {
R2.goodOneFactor = tmp2;
}
cubes.remove(R);
cubes.add(R1);
cubes.add(R2);
my = Double.MAX_VALUE;//gamma;
xCount++;
if (!queue.empty()) {
continue;
}
//double T[] = {0,0.0001,0.001,0.01,0.02,0.03,0.04,0.05,0.06,0.07,0.08,0.09,0.10,0.11,0.12,0.13,0.15,0.20,0.25,0.3,0.4,0.5,0.75,1.0,1.5,2.0,3.0,6.0};
double T[] = {0.0};
//double T[] = {0,0.0001,0.001,0.01,0.05,0.10,0.25,0.5,1.0,2.0,5.0};
int IndexForT[] = new int[T.length];
for (int t = 0; t < T.length; t++) {
int best = -1;
double bestLdiff = 10000000000.0;
for (int i = 0; i < cubes.size(); i++) {
HyperCube c = cubes.get(i);
double Lstar = c.CalculateLForTarget(gamma - T[t]);
//double Ldiff = Lstar - c.L;//VectorSub(Lstar , c.L);
double Ldiff = c.highestLowBound;
if (Ldiff < bestLdiff) {
best = i;
bestLdiff = Ldiff;
}
if (xCount % 20 == 19) {
best = 0;
bestLdiff = Ldiff;
break;
}
}
IndexForT[t] = best;
}
for (int i = 0; i < IndexForT.length; i++) {
if (IndexForT[i] == -1) {
continue;
}
for (int j = i + 1; j < IndexForT.length; j++) {
if (IndexForT[i] == IndexForT[j]) {
IndexForT[j] = -1;
}
}
//System.out.println("add T =" + T[i]);
queue.push(cubes.get(IndexForT[i]));
}
k++;
}
}
}
© 2015 - 2025 Weber Informatics LLC | Privacy Policy