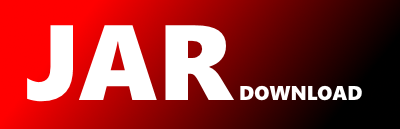
edu.mines.jtk.opt.QuadraticSolver Maven / Gradle / Ivy
Go to download
Show more of this group Show more artifacts with this name
Show all versions of edu-mines-jtk Show documentation
Show all versions of edu-mines-jtk Show documentation
Java packages for science and engineering
The newest version!
/****************************************************************************
Copyright 2003, Landmark Graphics and others.
Licensed under the Apache License, Version 2.0 (the "License");
you may not use this file except in compliance with the License.
You may obtain a copy of the License at
http://www.apache.org/licenses/LICENSE-2.0
Unless required by applicable law or agreed to in writing, software
distributed under the License is distributed on an "AS IS" BASIS,
WITHOUT WARRANTIES OR CONDITIONS OF ANY KIND, either express or implied.
See the License for the specific language governing permissions and
limitations under the License.
****************************************************************************/
package edu.mines.jtk.opt;
import edu.mines.jtk.util.Almost;
import edu.mines.jtk.util.Monitor;
import java.util.logging.Logger;
/**
* Minimize a simple quadratic objective function.
* Finds the x that minimizes the quadratic function 0.5 x'Hx + b'x .
* Solves Hx + b = 0 for x (the Normal Equations of a least-squares problem)
* b is the gradient for x=0, and H is the hessian.
* The algorithm is a conjugate gradient.
* A is an approximate inverse Hessian, making AH more diagonal
* and improving convergence.
* A may also include a model-dependent conditioning to converge
* earlier on eigenfunction of most importance.
* The algorithm assumes that the application of H dominates the cost.
*
* x = p = u = 0;
* beta = 0;
* g = b;
* a = A g;
* q = H a;
* do {
* p = -a + beta p
* u = Hp = -q + beta u
* scalar = -p'g/p'u
* x = x + scalar p
* if (done) return x
* g = H x + b = g + scalar u
* a = A g
* q = H a
* beta = p'H A g / p'H p = p'q/p'u
* }
*
*
* Also contains a solver for a least-squares inverse of a linear
* transform, using QuadraticTransform as a wrapper.
*
* @author W.S. Harlan
*/
public class QuadraticSolver {
private Quadratic _quadratic = null;
private static final Logger LOG = Logger.getLogger("edu.mines.jtk.opt");
/**
* Implement the Quadratic interface and pass to this constructor.
* Pass a vector b to the constructor (a copy is cloned internally).
* This solver calls Vect and Quadratic methods.
* Does not call Vect.multiplyInverseCovariance().
*
* @param quadratic Defines the Hessian quadratic term.
*/
public QuadraticSolver(final Quadratic quadratic) {
_quadratic = quadratic;
}
/**
* Return a new solution after the number of conjugate gradient
* iterations.
*
* @param numberIterations is the number of iterations to perform.
* @param monitor If non-null, then track all progress.
* @return Optimized solution
*/
public Vect solve(final int numberIterations, Monitor monitor) {
if (monitor == null) {
monitor = Monitor.NULL_MONITOR;
}
monitor.report(0.0);
VectConst b = _quadratic.getB(); // instance 1
monitor.report(1.0 / (numberIterations + 2.0));
final double bb = b.dot(b);
checkNaN(bb);
if (Almost.FLOAT.zero(bb)) {
LOG.fine("Gradient of quadratic is negligible. Not solving");
final Vect result = VectUtil.cloneZero(b);
monitor.report(1.0);
return result;
}
final Vect g = (Vect) b;
b = null; // reuse b
final Vect x = VectUtil.cloneZero(g); // instance 2
final Vect p = x.clone(); // instance 3
final Vect u = x.clone(); // instance 4
double pu = 0.0;
final Vect qa = g.clone(); // double use for instance 5
SOLVE:
for (int iter = 0; iter < numberIterations && !monitor.isCanceled(); iter++) {
double beta = 0.0;
{
final Vect q = qa;
VectUtil.copy(q, g);
_quadratic.inverseHessian(q);
q.postCondition(); // a = A g
_quadratic.multiplyHessian(q); // expensive
monitor.report((iter + 2.0) / (numberIterations + 2.0));
if (iter > 0) {
final double pq = p.dot(q);
checkNaN(pq);
beta = Almost.FLOAT.divide(pq, pu, 0);
if (beta < -5.0) {
beta = -5.0;
}
if (beta > 5.0) {
beta = 5.0;
}
}
u.add(beta, -1.0, q);
}
{ // Did not save "a" before calculating beta, to avoid extra instance.
final Vect a = qa;
VectUtil.copy(a, g);
_quadratic.inverseHessian(a);
a.postCondition();
p.add(beta, -1.0, a);
}
final double pg = p.dot(g);
checkNaN(pg);
pu = p.dot(u);
checkNaN(pu);
if (Almost.FLOAT.zero(pg) || Almost.FLOAT.zero(pu)) {
break SOLVE;
}
final double scalar = -pg / pu;
x.add(1.0, scalar, p);
if (iter == numberIterations - 1) {
break SOLVE;
}
g.add(1.0, scalar, u);
}
p.dispose();
u.dispose();
g.dispose();
qa.dispose();
monitor.report(1.0);
return x;
}
/**
* Solve quadratic objective function for linear transform.
* Minimizes
*
* [F(m+x)-data]'N[F(m+x)-data] + (m+x)'M(m+x)
*
* if dampOnlyPerturbation is true and
*
* [F(m+x)-data]'N[F(m+x)-data] + (x)'M(x)
*
* if dampOnlyPerturbation is false.
*
* @param data The data to be fit.
* @param referenceModel Initialize with this model.
* @param linearTransform Describes the linear transform.
* @param dampOnlyPerturbation If true then, only damp perturbations
* to reference model. If false, then damp the reference model plus
* the perturbation.
* @param conjugateGradIterations The specified number of conjugate
* gradient iterations.
* @param monitor Report progress here, if non-null.
* @return Result of optimization
*/
public static Vect solve(final VectConst data,
final VectConst referenceModel,
final LinearTransform linearTransform,
final boolean dampOnlyPerturbation,
final int conjugateGradIterations,
final Monitor monitor) {
final Transform transform = new LinearTransformWrapper(linearTransform);
final VectConst perturbModel = null;
final TransformQuadratic transformQuadratic =
new TransformQuadratic(data, referenceModel, perturbModel, transform,
dampOnlyPerturbation);
final QuadraticSolver quadraticSolver = new QuadraticSolver(transformQuadratic);
final Vect result = quadraticSolver.solve(conjugateGradIterations, monitor);
transformQuadratic.dispose();
result.add(1, 1, referenceModel);
return result;
}
/**
* Abort if NaN's appear.
*
* @param value Abort if this value is a NaN.
*/
private static void checkNaN(final double value) {
if (value * 0 != 0) {
throw new IllegalStateException("Value is a NaN");
}
}
}
© 2015 - 2025 Weber Informatics LLC | Privacy Policy