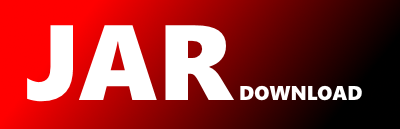
edu.ucla.sspace.clustering.BaseSpectralCut Maven / Gradle / Ivy
Go to download
Show more of this group Show more artifacts with this name
Show all versions of sspace-wordsi Show documentation
Show all versions of sspace-wordsi Show documentation
The S-Space Package is a collection of algorithms for building
Semantic Spaces as well as a highly-scalable library for designing new
distributional semantics algorithms. Distributional algorithms process text
corpora and represent the semantic for words as high dimensional feature
vectors. This package also includes matrices, vectors, and numerous
clustering algorithms. These approaches are known by many names, such as
word spaces, semantic spaces, or distributed semantics and rest upon the
Distributional Hypothesis: words that appear in similar contexts have
similar meanings.
The newest version!
/*
* Copyright 2011 Keith Stevens
*
* This file is part of the S-Space package and is covered under the terms and
* conditions therein.
*
* The S-Space package is free software: you can redistribute it and/or modify
* it under the terms of the GNU General Public License version 2 as published
* by the Free Software Foundation and distributed hereunder to you.
*
* THIS SOFTWARE IS PROVIDED "AS IS" AND NO REPRESENTATIONS OR WARRANTIES,
* EXPRESS OR IMPLIED ARE MADE. BY WAY OF EXAMPLE, BUT NOT LIMITATION, WE MAKE
* NO REPRESENTATIONS OR WARRANTIES OF MERCHANT- ABILITY OR FITNESS FOR ANY
* PARTICULAR PURPOSE OR THAT THE USE OF THE LICENSED SOFTWARE OR DOCUMENTATION
* WILL NOT INFRINGE ANY THIRD PARTY PATENTS, COPYRIGHTS, TRADEMARKS OR OTHER
* RIGHTS.
*
* You should have received a copy of the GNU General Public License
* along with this program. If not, see .
*/
package edu.ucla.sspace.clustering;
import edu.ucla.sspace.matrix.Matrix;
import edu.ucla.sspace.matrix.RowMaskedMatrix;
import edu.ucla.sspace.matrix.SparseRowMaskedMatrix;
import edu.ucla.sspace.matrix.SparseMatrix;
import edu.ucla.sspace.vector.DenseVector;
import edu.ucla.sspace.vector.DoubleVector;
import edu.ucla.sspace.vector.ScaledDoubleVector;
import edu.ucla.sspace.vector.SparseDoubleVector;
import edu.ucla.sspace.vector.Vectors;
import edu.ucla.sspace.vector.VectorIO;
import edu.ucla.sspace.vector.VectorMath;
import java.util.Arrays;
import java.util.logging.Logger;
/**
* An abstract class for computing a spectral cut over a data {@link Matrix}
* that represents a set of data points. The spectral cut attempts to find the
* a separation that minimizes the conductance between the two resulting
* regions. Often, this requires computing a complete affinity matrix from the
* data points, which requires O(n^2) time and space complexity. {@link
* SparseMatrix}s are a special case, Instead of computing the full affinity
* matrix, a centroid for the complete data set, and possible divided regions
* can be computed and used to evaluate the conductance (based on the
* transitivity of the dot product).
*
*
*
* There are several variations on computing the conductance of a matrix. This
* class does nearly all of the heavy computation, except for computing the
* second eigen vector of an affinity matrix. Subclasses need only implement
* {@link #computeSecondEigenVector}, which should return a dense {@link
* DoubleVector} that represents the eigen values of the affinity matrix.
* Implementations are suggested to avoid explicitly computing the full affinity
* matrix.
*
*
*
* This abstract class provides the rest of the needed functionality for {@link
* EigenCut}, such as computing the objective functions, selecting an optimal
* conductance cut accross the affinity matrix, and computing the split
* partitions.
*
* @author Keith Stevens
*/
public abstract class BaseSpectralCut implements EigenCut {
/**
* The logger used to record all output.
*/
private static final Logger LOGGER =
Logger.getLogger(BaseSpectralCut.class.getName());
/**
* The {@link Matrix} containing the data points.
*/
protected Matrix dataMatrix;
/**
* The number of rows in the data matrix. Used as a short hand in
* computations.
*/
protected int numRows;
/**
* The sum similarity values from each data point to all other data points,
* which is equivalent to the simiarltiy between each data point and the
* centroid of the entire data set.
*/
protected DoubleVector rho;
/**
* The centroid of the entire data set.
*/
protected DoubleVector matrixRowSums;
/**
* The summation of the {@code rho} values.
*/
protected double pSum;
/**
* The final ordering of data points in the first created region.
*/
protected int[] leftReordering;
/**
* The final ordering of data points in the first created region.
*/
protected int[] rightReordering;
/**
* The data points in the left region.
*/
protected Matrix leftSplit;
/**
* The data points in the right region.
*/
protected Matrix rightSplit;
/**
* {@inheritDoc}
*/
public double rhoSum() {
return pSum;
}
/**
* {@inheritDoc}
*/
public DoubleVector computeRhoSum(Matrix matrix) {
LOGGER.info("Computing rho and rhoSum");
// Compute the centroid of the entire data set.
int vectorLength = matrix.rows();
matrixRowSums = computeMatrixRowSum(matrix);
dataMatrix = matrix;
// Compute rho, where rho[i] = dataPoint_i DOT matrixRowSums.
rho = new DenseVector(vectorLength);
pSum = 0;
for (int r = 0; r < matrix.rows(); ++r) {
double dot = VectorMath.dotProduct(
matrixRowSums, matrix.getRowVector(r));
pSum += dot;
rho.set(r, dot);
}
return matrixRowSums;
}
/**
* {@inheritDoc}
*/
public void computeCut(Matrix matrix) {
dataMatrix = matrix;
int numRows = matrix.rows();
// Compute the centroid of the entire data set.
int vectorLength = matrix.rows();
DoubleVector matrixRowSums = computeRhoSum(matrix);
LOGGER.info("Computing the second eigen vector");
// Compute the second largest eigenvector of the normalized affinity
// matrix with respect to pi*D^-1, which is similar to it's first eigen
// vector.
DoubleVector v = computeSecondEigenVector(matrix, vectorLength);
// Sort the rows of the original matrix based on the values of the eigen
// vector.
Index[] elementIndices = new Index[v.length()];
for (int i = 0; i < v.length(); ++i)
elementIndices[i] = new Index(v.get(i), i);
Arrays.sort(elementIndices);
// Create a reordering mapping for the indices in the original data
// matrix and of rho. The ith data point and rho value will be ordered
// based on the position of the ith value in the second eigen vector
// after it has been sorted.
DoubleVector sortedRho = new DenseVector(matrix.rows());
int[] reordering = new int[v.length()];
for (int i = 0; i < v.length(); ++i) {
reordering[i] = elementIndices[i].index;
sortedRho.set(i, rho.get(elementIndices[i].index));
}
// Create the sorted matrix based on the reordering. Note that both row
// masked matrices internally handle masking a masked matrix to avoid
// recursive calls to the index lookups.
Matrix sortedMatrix;
if (matrix instanceof SparseMatrix)
sortedMatrix = new SparseRowMaskedMatrix(
(SparseMatrix) matrix, reordering);
else
sortedMatrix = new RowMaskedMatrix(matrix, reordering);
LOGGER.info("Computing the spectral cut");
// Compute the index at which the best cut can be made based on the
// reordered data matrix and rho values.
int cutIndex = computeCut(
sortedMatrix, sortedRho, pSum, matrixRowSums);
leftReordering = Arrays.copyOfRange(reordering, 0, cutIndex);
rightReordering = Arrays.copyOfRange(
reordering, cutIndex, reordering.length);
// Create the split permuted matricies.
if (matrix instanceof SparseMatrix) {
leftSplit = new SparseRowMaskedMatrix((SparseMatrix) matrix,
leftReordering);
rightSplit = new SparseRowMaskedMatrix((SparseMatrix) matrix,
rightReordering);
} else {
leftSplit = new RowMaskedMatrix(matrix, leftReordering);
rightSplit = new RowMaskedMatrix(matrix, rightReordering);
}
}
/**
* Returns a {@link DoubleVector} representing the secord largest eigen
* vector for the data set.
*/
protected abstract DoubleVector computeSecondEigenVector(Matrix matrix,
int vectorLength);
/**
* {@inheritDoc}
*/
public Matrix getLeftCut() {
return leftSplit;
}
/**
* {@inheritDoc}
*/
public Matrix getRightCut() {
return rightSplit;
}
/**
* {@inheritDoc}
*/
public int[] getLeftReordering() {
return leftReordering;
}
/**
* {@inheritDoc}
*/
public int[] getRightReordering() {
return rightReordering;
}
/**
* {@inheritDoc}
*/
public double getKMeansObjective() {
// Note that the scaled vector handles any recursive scaling.
DoubleVector centroid = new ScaledDoubleVector(
matrixRowSums, 1/((double) dataMatrix.rows()));
double score = 0;
for (int r = 0; r < dataMatrix.rows(); ++r)
score += VectorMath.dotProduct(
centroid, dataMatrix.getRowVector(r));
return score;
}
/**
* {@inheritDoc}
*/
public double getKMeansObjective(
double alpha, double beta,
int leftNumClusters, int[] leftAssignments,
int rightNumClusters, int[] rightAssignments) {
double score = 0;
score += kMeansObjective(leftNumClusters, leftAssignments, leftSplit);
score += kMeansObjective(
rightNumClusters, rightAssignments, rightSplit);
return score;
}
/**
* Returns the K-Means objective over an arbitrary clustering assignment for
* the data set.
*/
public static double kMeansObjective(int numClusters,
int[] assignments,
Matrix data) {
// Initialize the clusters.
double score = 0;
DoubleVector[] centroids = new DoubleVector[numClusters];
double[] sizes = new double[numClusters];
for (int i = 0; i < centroids.length; ++i)
centroids[i] = new DenseVector(data.columns());
// Add vectors to each cluster.
for (int i = 0; i < assignments.length; ++i) {
VectorMath.add(centroids[assignments[i]], data.getRowVector(i));
sizes[assignments[i]]++;
}
// Scale non empty centroids. Note that the scaled vector handles any
// recursive scaling.
for (int i = 0; i < centroids.length; ++i)
if (sizes[i] != 0)
centroids[i] = new ScaledDoubleVector(centroids[i],1d/sizes[i]);
// Compute the total distance of each asisgned point to it's centroid.
for (int i = 0; i < assignments.length; ++i)
score += VectorMath.dotProduct(
centroids[assignments[i]], data.getRowVector(i));
return score;
}
/**
* {@inheritDoc}
*/
public double getSplitObjective(
double alpha, double beta,
int leftNumClusters, int[] leftAssignments,
int rightNumClusters, int[] rightAssignments) {
// Compute the objective when we keep the two branches split.
double intraClusterScore = 0;
// Compute the intra cluster score for the left region.
int[] leftClusterCounts = new int[leftNumClusters];
intraClusterScore += computeIntraClusterScore(
leftAssignments, leftSplit, leftClusterCounts);
// Compute the intra cluster score for the right region.
int[] rightClusterCounts = new int[rightNumClusters];
intraClusterScore += computeIntraClusterScore(
rightAssignments, rightSplit, rightClusterCounts);
// Compute the inter cluster score. Since the sum of the rho vector is
// equivalent to the total summation of the affinity matrix, the inter
// cluster score is the sum of similarity scores not covered by the
// intra cluster score, without any duplicate similarity scores.
double interClusterScore = (pSum - numRows) / 2.0 - intraClusterScore;
// Compute the number of comparisons made for each region.
int pairCount = comparisonCount(leftClusterCounts) +
comparisonCount(rightClusterCounts);
// Reset the intraClusterScore such that a low intra cluster similarity
// leads to a higher score.
intraClusterScore = pairCount - intraClusterScore;
return alpha * intraClusterScore + beta * interClusterScore;
}
/**
* {@inheritDoc}
*/
public double getMergedObjective(double alpha, double beta) {
double intraScore = pSum - numRows / 2.0;
double pairCount = (numRows * (numRows -1)) / 2.0;
return alpha * (pairCount - intraScore);
}
/**
* Computes the inter cluster objective for a clustering result.
*
* @param result The set of cluster assignments for a set of vectors.
* @param m the matrix containing each row in the cluster result.
*/
private static double computeIntraClusterScore(int[] assignments,
Matrix m,
int[] numComparisons) {
DoubleVector[] centroids = new DoubleVector[numComparisons.length];
int[] centroidSizes = new int[numComparisons.length];
double intraClusterScore = 0;
for (int i = 0; i < assignments.length; ++i) {
int assignment = assignments[i];
DoubleVector v = m.getRowVector(i);
if (centroids[assignment] == null)
centroids[assignment] = Vectors.copyOf(v);
else {
DoubleVector centroid = centroids[assignment];
intraClusterScore += (centroidSizes[assignment] -
VectorMath.dotProduct(v, centroid));
VectorMath.add(centroid, v);
numComparisons[assignment] += centroidSizes[assignment];
}
centroidSizes[assignment]++;
}
return intraClusterScore;
}
/**
* Returns the number of comparisons made for a cluster.
*/
protected static int comparisonCount(int[] clusterSizes) {
int total = 0;
for (int count : clusterSizes)
total += count;
return total;
}
/**
* Returns a {@link DoubleVector} that is the orthonormalized version of
* {@code v} with respect to {@code other}. This orthonormalization is done
* by simply modifying the value of v[0] such that it balances out the
* similarity between {@code v} and {@code other} in all other dimensions.
*/
protected static DoubleVector orthonormalize(DoubleVector v,
DoubleVector other) {
double dot = VectorMath.dotProduct(v, other);
dot -= v.get(0) * other.get(0);
if (other.get(0) != 0d) {
dot /= other.get(0);
v.add(0, -dot);
}
dot = VectorMath.dotProduct(v, v);
if (1/dot == 0d || dot == 0)
return v;
// Note that the scaled vector handles any recursive scaling.
return new ScaledDoubleVector(v, 1/dot);
}
/**
* Returns the index at which {@code matrix} should be cut such that the
* conductance between the two partitions is minimized. This is done such
* that the sparsity of the data matrix is maintained and all the entire
* operation is linear with respect to the number of non zeros in the
* matrix.
*/
protected static int computeCut(Matrix matrix,
DoubleVector rho,
double rhoSum,
DoubleVector matrixRowSums) {
// Compute the conductance of the newly sorted matrix.
DoubleVector x = new DenseVector(matrix.columns());
DoubleVector y = matrixRowSums;
VectorMath.subtract(y, x);
// First compute x and y, which are summations of different cuts of the
// matrix, starting with x being the first row and y being the summation
// of all other rows. While doing this, also compute different
// summations of values in the rho vector using the same cut.
VectorMath.add(x, matrix.getRowVector(0));
double rhoX = rho.get(0);
double rhoY = rhoSum - rho.get(0);
// Compute the dot product between the first possible cut.
double u = VectorMath.dotProduct(x, y);
// Find the current conductance for the cut, assume that this is the
// best so far.
double minConductance = u / Math.min(rhoX, rhoY);
int cutIndex = 0;
// Compute the other possible cuts, ignoring the last cut, which would
// leave no data points in a partition. The cut with the smallest
// conductance is maintained.
for (int i = 1; i < rho.length() - 2; ++i) {
// Compute the new value of u, the denominator for computing the
// conductance.
DoubleVector vector = matrix.getRowVector(i);
double xv = VectorMath.dotProduct(x, vector);
double yv = VectorMath.dotProduct(y, vector);
u = u - xv + yv + 1;
// Shift over vectors from y to x.
VectorMath.add(x, vector);
VectorMath.subtract(y, vector);
// Shift over values from the rho vector.
rhoX += rho.get(i);
rhoY -= rho.get(i);
// Recompute the new conductance and check if it's the smallest.
double conductance = u / Math.min(rhoX, rhoY);
if (conductance <= minConductance) {
minConductance = conductance;
cutIndex = i;
}
}
return cutIndex+1;
}
/**
* Returns the dot product between the transpose of a given matrix and a
* given vector. This method has special casing for a {@code SparseMatrix}.
* This method also assumes that {@code matrix} is row based and iterates
* over each of the values in the row before iterating over another row.
*/
protected static DoubleVector computeMatrixTransposeV(Matrix matrix,
DoubleVector v) {
DoubleVector newV = new DenseVector(matrix.columns());
if (matrix instanceof SparseMatrix) {
SparseMatrix smatrix = (SparseMatrix) matrix;
for (int r = 0; r < smatrix.rows(); ++r) {
SparseDoubleVector row = smatrix.getRowVector(r);
int[] nonZeros = row.getNonZeroIndices();
for (int c : nonZeros)
newV.add(c, row.get(c) * v.get(r));
}
} else {
for (int r = 0; r < matrix.rows(); ++r)
for (int c = 0; c < matrix.columns(); ++c)
newV.add(c, matrix.get(r, c) * v.get(r));
}
return newV;
}
/**
* Computes the dot product between a given matrix and a given vector {@code
* newV}. The result is stored in {@code v}. This method has special
* casing for when {@code matrix} is a {@code SparseMatrix}. This method
* also assumes that {@code matrix} is row based and iterates over each of
* the values in the row before iterating over another row.
*/
protected static void computeMatrixDotV(Matrix matrix,
DoubleVector newV,
DoubleVector v) {
// Special case for sparse matrices.
if (matrix instanceof SparseMatrix) {
SparseMatrix smatrix = (SparseMatrix) matrix;
for (int r = 0; r < smatrix.rows(); ++r) {
double vValue = 0;
SparseDoubleVector row = smatrix.getRowVector(r);
int[] nonZeros = row.getNonZeroIndices();
for (int c : nonZeros)
vValue += row.get(c) * newV.get(c);
v.set(r, vValue);
}
} else {
// Handle dense matrices.
for (int r = 0; r < matrix.rows(); ++r) {
double vValue = 0;
for (int c = 0; c < matrix.columns(); ++c)
vValue += matrix.get(r, c) * newV.get(c);
v.set(r, vValue);
}
}
}
/**
* Compute the row sums of the values in {@code matrix} and returns the
* values in a vector of length {@code matrix.columns()}.
*/
protected static DoubleVector computeMatrixRowSum(
T matrix) {
DoubleVector rowSums = new DenseVector(matrix.columns());
for (int r = 0; r < matrix.rows(); ++r)
VectorMath.add(rowSums, matrix.getRowVector(r));
return rowSums;
}
/**
* A simple comparable data struct holding a row vector's weight and the
* vector's original index in a matrix.
*/
protected static class Index implements Comparable {
public final double weight;
public final int index;
public Index(double weight, int index) {
this.weight = weight;
this.index = index;
}
public int compareTo(Index other) {
if (this.weight > other.weight)
return 1;
if (this.weight < other.weight)
return -1;
return this.index - other.index;
}
}
}
© 2015 - 2025 Weber Informatics LLC | Privacy Policy