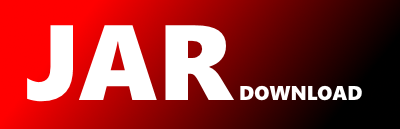
edu.ucla.sspace.matrix.MinSimilarityAffinityMatrixCreator Maven / Gradle / Ivy
Go to download
Show more of this group Show more artifacts with this name
Show all versions of sspace-wordsi Show documentation
Show all versions of sspace-wordsi Show documentation
The S-Space Package is a collection of algorithms for building
Semantic Spaces as well as a highly-scalable library for designing new
distributional semantics algorithms. Distributional algorithms process text
corpora and represent the semantic for words as high dimensional feature
vectors. This package also includes matrices, vectors, and numerous
clustering algorithms. These approaches are known by many names, such as
word spaces, semantic spaces, or distributed semantics and rest upon the
Distributional Hypothesis: words that appear in similar contexts have
similar meanings.
The newest version!
package edu.ucla.sspace.matrix;
import edu.ucla.sspace.similarity.SimilarityFunction;
import edu.ucla.sspace.vector.DoubleVector;
import edu.ucla.sspace.vector.SparseDoubleVector;
import java.io.BufferedInputStream;
import java.io.DataInputStream;
import java.io.File;
import java.io.FileInputStream;
import java.io.IOError;
import java.io.IOException;
import java.io.PrintWriter;
import java.util.logging.Logger;
/**
* This {@link AffinityMatrixCreator} adds an edge between two data points, i
* and j, if the similarity between them is above a certain threshold. This
* relationship is symmetric.
*
* @author David Jurgens
* @author Keith Stevens
*/
public class MinSimilarityAffinityMatrixCreator
implements AffinityMatrixCreator {
private static final Logger LOG =
Logger.getLogger(MinSimilarityAffinityMatrixCreator.class.getName());
private SimilarityFunction edgeSim;
private SimilarityFunction kernelSim;
private double edgeSimThreshold;
public void setParams(double... params) {
this.edgeSimThreshold = params[0];
}
/**
* {@inheritDoc}
*/
public void setFunctions(SimilarityFunction edgeSim,
SimilarityFunction kernelSim) {
this.edgeSim = edgeSim;
this.kernelSim = kernelSim;
}
/**
* {@inheritDoc}
*/
public MatrixFile calculate(Matrix input) {
try {
File affMatrixFile = File.createTempFile("affinty-matrix",".dat");
PrintWriter affMatrixWriter = new PrintWriter(affMatrixFile);
int rows = input.rows();
// Iterate through each row, i, in the data matrix and compare row i
// to each proceeding row, j. If the similarity is above the edge
// similarity threshold, emit an edge between row i and row j and
// between row j and row i, assuming that the edge similarity metric
// is symmetric. Each edge is written in the Matlab Sparse matrix
// format.
for (int i = 0; i < rows; ++i) {
LOG.fine("computing affinity for row " + i);
DoubleVector row1 = input.getRowVector(i);
for (int j = i+1; j < rows; ++j) {
DoubleVector row2 = input.getRowVector(j);
double dataSimilarity = edgeSim.sim(row1, row2);
// If the edge similarity is above the threshold, compute
// the kernel similarity for each new edge.
if (dataSimilarity > edgeSimThreshold) {
double edgeWeight = kernelSim.sim(row1, row2);
affMatrixWriter.printf("%d %d %f\n",i+1,j+1,edgeWeight);
// If the kernel metric is symmetric, just reuse the
// previously calculated edge weight. Otherwise
// recalculate it.
edgeWeight = (kernelSim.isSymmetric())
? edgeWeight
: kernelSim.sim(row2, row1);
affMatrixWriter.printf("%d %d %f\n",j+1,i+1,edgeWeight);
}
}
}
affMatrixWriter.close();
return new MatrixFile(affMatrixFile, MatrixIO.Format.MATLAB_SPARSE);
} catch (IOException ioe) {
throw new IOError(ioe);
}
}
/**
* {@inheritDoc}
*/
public MatrixFile calculate(MatrixFile input) {
return calculate(input, false);
}
/**
* {@inheritDoc}
*/
public MatrixFile calculate(MatrixFile input, boolean useColumns) {
File matrixFile = input.getFile();
MatrixIO.Format format = input.getFormat();
// IMPLEMENTATION NOTE: since the user has requested the matrix be dealt
// with as a file, we need to keep the matrix on disk. However, the
// input matrix format may not be conducive to efficiently comparing
// rows with each other (e.g. MATLAB_SPARSE is inefficient), so convert
// the matrix to a better format.
try {
LOG.fine("Converting input matrix to new format for faster " +
"calculation of the affinity matrix");
// Keep the matrix on disk, but convert it to a transposed SVDLIBC
// sparse binary, which allows for easier efficient row-by-row
// comparisons (which are really columns). Note that if the data is
// already in this format, the conversion is a no-op.
//
// NOTE: the !useColumns is used for the transpose because if we
// want to use the rows, we need the data transposed to begin with
// since the SVDLIBC sparse binary will give us column information
// to start with
File converted =
MatrixIO.convertFormat(matrixFile, format,
MatrixIO.Format.SVDLIBC_SPARSE_BINARY,
!useColumns);
LOG.fine("Calculating the affinity matrix");
// Read off the matrix dimensions
DataInputStream dis = new DataInputStream(
new BufferedInputStream(new FileInputStream(converted)));
// CRITICAL NOTE: because we are interpreting the columns as rows,
// the dimensions are read in *reverse order* from how they are
// stored in the file.
int cols = dis.readInt();
int rows = dis.readInt();
dis.close();
// Once we know the matrix dimensions, create an iterator over the
// data, and repeatedly loop through the columns (which are really
// rows in the original matrix) to create the affinity matrix.
File affMatrixFile = File.createTempFile("affinity-matrix",".dat");
PrintWriter affMatrixWriter = new PrintWriter(affMatrixFile);
// Keep track of the first row and have a reference to the next row.
// The nextRow reference avoid us having to advance into data
// unnecessarily to retrieval the vector for processing to start
SparseDoubleVector curRow = null;
SparseDoubleVector nextRow = null;
SvdlibcSparseBinaryFileRowIterator matrixIter =
new SvdlibcSparseBinaryFileRowIterator(converted);
for (int row = 0; row < rows; ++row) {
LOG.fine("computing affinity for row " + row);
// Loop through each of the rows, gathering the statistics
// necessary to compute the affinity matrix.
for (int other = 0; other < rows; ++other) {
// Special case for the very first row
if (row == 0 && curRow == null) {
curRow = matrixIter.next();
continue;
}
SparseDoubleVector otherRow = matrixIter.next();
// Special case for the similarity threshold, which is
// symmetric. In this case, we can skip over processing any
// rows that occur before the current row
if (other < row)
continue;
// Save the row that will be used next so we have it to do
// comparisons with for earlier rows in the file
if (other == row + 1)
nextRow = otherRow;
// Determine if the current row and the other row should be
// linked in the affinity matrix. For code simplicity, both
// the k-nearest neighbors and the similarity threshold code
// are supported within the I/O, with the caller specifying
// which to use.
double dataSimilarity = edgeSim.sim(curRow, otherRow);
if (dataSimilarity > edgeSimThreshold) {
double edgeWeight = kernelSim.sim(curRow, otherRow);
affMatrixWriter.printf("%d %d %f\n",
row+1, other+1, edgeWeight);
// If the kernel metric is symmetric, just reuse the
// previously calculated edge weight. Otherwise
// recalculate it.
edgeWeight = (kernelSim.isSymmetric())
? edgeWeight
: kernelSim.sim(otherRow, curRow);
affMatrixWriter.printf("%d %d %f\n",
other+1, row+1, edgeWeight);
}
}
curRow = nextRow;
matrixIter.reset();
}
// Finish writing the matrix
affMatrixWriter.close();
return new MatrixFile(affMatrixFile, MatrixIO.Format.MATLAB_SPARSE);
} catch (IOException ioe) {
throw new IOError(ioe);
}
}
}
© 2015 - 2025 Weber Informatics LLC | Privacy Policy