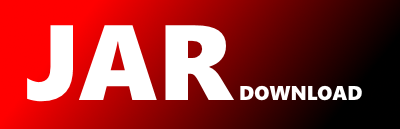
edu.ucla.sspace.matrix.SVD Maven / Gradle / Ivy
Show all versions of sspace-wordsi Show documentation
/*
* Copyright 2009 David Jurgens
*
* This file is part of the S-Space package and is covered under the terms and
* conditions therein.
*
* The S-Space package is free software: you can redistribute it and/or modify
* it under the terms of the GNU General Public License version 2 as published
* by the Free Software Foundation and distributed hereunder to you.
*
* THIS SOFTWARE IS PROVIDED "AS IS" AND NO REPRESENTATIONS OR WARRANTIES,
* EXPRESS OR IMPLIED ARE MADE. BY WAY OF EXAMPLE, BUT NOT LIMITATION, WE MAKE
* NO REPRESENTATIONS OR WARRANTIES OF MERCHANT- ABILITY OR FITNESS FOR ANY
* PARTICULAR PURPOSE OR THAT THE USE OF THE LICENSED SOFTWARE OR DOCUMENTATION
* WILL NOT INFRINGE ANY THIRD PARTY PATENTS, COPYRIGHTS, TRADEMARKS OR OTHER
* RIGHTS.
*
* You should have received a copy of the GNU General Public License
* along with this program. If not, see .
*/
package edu.ucla.sspace.matrix;
import edu.ucla.sspace.matrix.MatrixIO.Format;
import edu.ucla.sspace.matrix.Matrix.Type;
import edu.ucla.sspace.matrix.factorization.SingularValueDecompositionLibC;
import edu.ucla.sspace.matrix.factorization.SingularValueDecompositionLibJ;
import java.io.BufferedReader;
import java.io.DataInputStream;
import java.io.File;
import java.io.FileInputStream;
import java.io.FileReader;
import java.io.InputStreamReader;
import java.io.IOException;
import java.io.PrintWriter;
import java.lang.reflect.Constructor;
import java.lang.reflect.InvocationTargetException;
import java.lang.reflect.Method;
import java.util.logging.Level;
import java.util.logging.Logger;
/**
* A utililty class for invoking different implementations of the Singular
* Value Decomposition (SVD). The SVD is a way of factoring any matrix A into
* three matrices U Σ
* VT such that Σ is a diagonal matrix containing the singular values
* of A. The singular
* values of Σ
* are ordered according to which causes the most variance in the values of
* A.
*
*
*
* All SVD operations return an array of three {@link Matrix} instances that
* correspond to U Σ
* and
* VT. Regardless of which algorithm is used, the
* matrices will be returned in this same orientation.
*
*
*
* Five different SVD algorithms are possible:
*
*
* - SVDLIBC
*
* - Matlab svds
*
*
* - GNU
* Octave svds - Note that
* this is an optional package and requires that the ARPACK bindings
* for Octave is installed.
*
* - COLT SVD
*
* - JAMA
* SVD
*
*
*
* Support for these algorithms requires that they are invokable from the path
* used to start the current JVM, or in the case of JAMA, are available in the
* classpath. Note that if JAMA is used in conjunction with a {@code .jar}
* executable, it's location needs to be specified with the {@code jama.path}
* system property. This can be set on the command line using
* -Djama.path=path/to/jama. Similarly, if COLT is to be used in
* conjunction with a {@code .jar}, the location of the {@code colt.jar} file
* should be specified using the {@code colt.path} system property.
*
*
*
* Users may select which algorithm to use manually using the {@code Algorithm}
* enum. The additional enum value {@code ANY} will select the fastest
* algorithm available. If no algorithm is available, a {@code
* UnsupporteOperationException} is thrown at run-time.
*
*
*
* This class will automatically convert a file to the appropriate matrix format
* for the required algorithm.
*
* @author David Jurgens
*
* @see MatrixIO
*/
public class SVD {
private static final Logger SVD_LOGGER =
Logger.getLogger(SVD.class.getName());
/**
* Which algorithm to use for computing the Singular Value Decomposition
* (SVD) of a matrix.
*/
public enum Algorithm {
SVDLIBC,
SVDLIBJ,
MATLAB,
OCTAVE,
JAMA,
COLT,
ANY
}
/**
* Uninstantiable
*/
private SVD() { }
/**
* Returns the fastest {@link MatrixFactorization} implementation of
* Singular Value Decomposition available, or {@code null} if no
* implementation is available.
*/
public static MatrixFactorization getFastestAvailableFactorization() {
if (isSVDLIBCavailable())
return new SingularValueDecompositionLibC();
else
return new SingularValueDecompositionLibJ();
}
/**
* Returns the {@link MatrixFactorization} implementation corresponding to
* the {@link Algorithm} name.
*/
public static MatrixFactorization getFactorization(Algorithm alg) {
switch (alg) {
case SVDLIBJ:
return new SingularValueDecompositionLibJ();
default:
return new SingularValueDecompositionLibC();
}
}
/**
* Returns the fastest available SVD algorithm supported on the current
* system.
*
*
*
* Use {@link getFastestAvailableFactorization} instead.
*
* @return the fastest algorithm available or {@code null} if no SVD
* algorithm is available
*/
@Deprecated
static Algorithm getFastestAvailableAlgorithm() {
if (isSVDLIBCavailable())
return Algorithm.SVDLIBC;
else
return Algorithm.SVDLIBJ;
// NOTE: commented out because SVDLIBJ is now included in the
// distribution and is likely faster than all of these implementations,
// though this should probably be tested to confirm. -jurgens
/*
else if (isMatlabAvailable())
return Algorithm.MATLAB;
else if (isOctaveAvailable())
return Algorithm.OCTAVE;
else if (isJAMAavailable())
return Algorithm.JAMA;
else if (isColtAvailable())
return Algorithm.COLT;
else
return null;
*/
}
/**
* Returns U, S, VT matrices for the SVD of the matrix using the
* fastest available SVD algorithm to generate the specified number of
* singular values.
*
*
*
* Use a {@link MatrixFactorization} implementation instead.
*
* @param m the matrix on which the SVD should be computed
* @param dimensions the number of singular values to calculate
*
* @return an array of {@code Matrix} objects for the U, S, and
* VT matrices in that order
*
* @throws UnsupportedOperationException if no SVD algorithm is available
*/
@Deprecated
public static Matrix[] svd(Matrix m, int dimensions) {
// Determine which algorithm is the fastest in order to decide which
// format the matrix file should be written
return svd(m, getFastestAvailableAlgorithm(), dimensions);
}
/**
* Returns U, S, VT matrices for the SVD of the matrix using the
* specified SVD algorithm to generate the specified number of singular
* values.
*
*
*
* Use a {@link MatrixFactorization} implementation instead.
*
* @param m the matrix on which the SVD should be computed
* @param algorithm the algorithm to use in computing the SVD
* @param dimensions the number of singular values to calculate
*
* @return an array of {@code Matrix} objects for the U, S, and
* VT matrices in that order
*
* @throws UnsupportedOperationException if no SVD algorithm is available
*/
@Deprecated
public static Matrix[] svd(Matrix m, Algorithm algorithm, int dimensions) {
// Determine which algorithm is the fastest in order to decide which
// format the matrix file should be written
if (algorithm.equals(Algorithm.ANY))
algorithm = getFastestAvailableAlgorithm();
if (algorithm == null)
throw new UnsupportedOperationException(
"No SVD algorithm is available on this system");
Format fmt = null;
switch (algorithm) {
// In the case of COLT or JAMA, avoid writing the matrix to disk
// altogether, and just pass it in directly. This avoids the I/O
// overhead, althought both methods require that the matrix be converted
// into arrays first.
case SVDLIBJ:
return SvdlibjDriver.svd(m, dimensions);
case COLT:
return coltSVD(m.toDenseArray(), !(m instanceof SparseMatrix),
dimensions);
case JAMA:
return jamaSVD(m.toDenseArray(), dimensions);
// Otherwise, covert to binary SVDLIBC
case SVDLIBC:
fmt = Format.SVDLIBC_SPARSE_BINARY;
break;
// Or to Matlab sparse to cover Matlab and Octave
default:
fmt = Format.MATLAB_SPARSE;
}
try {
File tmpFile = File.createTempFile("matrix-svd", ".dat");
tmpFile.deleteOnExit();
MatrixIO.writeMatrix(m, tmpFile, fmt);
return svd(tmpFile, algorithm, fmt, dimensions);
}
catch (IOException ioe) {
SVD_LOGGER.log(Level.SEVERE, "convertFormat", ioe);
}
throw new UnsupportedOperationException(
"SVD algorithm failed in writing matrix to disk");
}
/**
* Returns U, S, VT matrices for the SVD of the matrix file in
* {@link Format#MATLAB_SPARSE Matlab sparse} format using the specified SVD
* algorithm to generate the specified number of singular values.
*
*
*
* Use a {@link MatrixFactorization} implementation instead.
*
* @param matrix a file containing a matrix
* @param dimensions the number of singular values to calculate
*
* @return an array of {@code Matrix} objects for the U, S, and
* VT matrices in that order
*
* @throws UnsupportedOperationException if no SVD algorithm is available
*/
@Deprecated
public static Matrix[] svd(File matrix, int dimensions) {
return svd(matrix, Algorithm.ANY,
Format.MATLAB_SPARSE, dimensions);
}
/**
* Returns U, S, VT matrices for the SVD of the matrix file in
* {@link Format#MATLAB_SPARSE Matlab sparse} format using the specified SVD
* algorithm to generate the specified number of singular values.
*
*
*
* Use a {@link MatrixFactorization} implementation instead.
*
* @param matrix a file containing a matrix
* @param alg which algorithm to use for computing the SVD
* @param dimensions the number of singular values to calculate
*
* @return an array of {@code Matrix} objects for the U, S, and
* VT matrices in that order
*
* @throws UnsupportedOperationException if the provided SVD algorithm is
* unavailable
*/
@Deprecated
public static Matrix[] svd(File matrix, Algorithm alg, int dimensions) {
return svd(matrix, alg, Format.MATLAB_SPARSE, dimensions);
}
/**
* Returns U, S, VT matrices for the SVD of the matrix file in
* specified format using the the fastst SVD algorithm available to generate
* the specified number of singular values.
*
*
*
* Use a {@link MatrixFactorization} implementation instead.
*
* @param matrix a file containing a matrix
* @param format the format of the input matrix file
* @param dimensions the number of singular values to calculate
*
* @return an array of {@code Matrix} objects for the U, S, and
* VT matrices in that order
*
* @throws UnsupportedOperationException if no SVD algorithm is available
*/
@Deprecated
public static Matrix[] svd(File matrix, Format format, int dimensions) {
return svd(matrix, Algorithm.ANY, format, dimensions);
}
/**
* Returns U, S, VT matrices for the SVD of the matrix file in
* specified format using the the specified SVD algorithm available to
* generate the specified number of singular values.
*
*
*
* Use a {@link MatrixFactorization} implementation instead.
*
* @param matrix a file containing a matrix
* @param alg which algorithm to use for computing the SVD
* @param format the format of the input matrix file
* @param dimensions the number of singular values to calculate
*
* @return an array of {@code Matrix} objects for the U, S, and
* VT matrices in that order
*
* @throws UnsupportedOperationException if the provided SVD algorithm is
* unavailable
*/
@Deprecated
public static Matrix[] svd(File matrix, Algorithm alg,
Format format, int dimensions) {
try {
File converted = null;
switch (alg) {
case SVDLIBC: {
// check whether the input matrix is in an SVDLIBC-acceptable
// format already and if not convert
switch (format) {
case SVDLIBC_DENSE_BINARY:
case SVDLIBC_DENSE_TEXT:
case SVDLIBC_SPARSE_TEXT:
case SVDLIBC_SPARSE_BINARY:
converted = matrix;
break;
default:
SVD_LOGGER.fine("converting input matrix format " +
"from" + format + " to SVDLIBC " +
"ready format");
converted = MatrixIO.convertFormat(
matrix, format, Format.SVDLIBC_SPARSE_BINARY);
format = Format.SVDLIBC_SPARSE_BINARY;
break;
}
return svdlibc(converted, dimensions, format);
}
case SVDLIBJ: {
return SvdlibjDriver.svd(matrix, format, dimensions);
}
case JAMA: {
@SuppressWarnings("deprecation")
double[][] inputMatrix =
MatrixIO.readMatrixArray(matrix, format);
return jamaSVD(inputMatrix, dimensions);
}
case MATLAB:
// If the format of the input matrix isn't immediately useable
// by Matlab convert it
if (!format.equals(Format.MATLAB_SPARSE)) {
matrix = MatrixIO.convertFormat(
matrix, format, Format.MATLAB_SPARSE);
}
return matlabSVDS(matrix, dimensions);
case OCTAVE:
// If the format of the input matrix isn't immediately useable
// by Octave convert it
if (!format.equals(Format.MATLAB_SPARSE)) {
matrix = MatrixIO.convertFormat(
matrix, format, Format.MATLAB_SPARSE);
}
return octaveSVDS(matrix, dimensions);
case COLT: {
@SuppressWarnings("deprecation")
double[][] m = MatrixIO.readMatrixArray(matrix, format);
return coltSVD(m, Matrices.isDense(format), dimensions);
}
case ANY:
// NOTE: Since the addition of SVDLIBJ, all algorithms except
// SVDLIBC have been rendered obsolete. Therefore, we see if
// SVDLIBC is available and then default to SVDLIBJ.
if (isSVDLIBCavailable()) {
try {
// check whether the input matrix is in an
// SVDLIBC-acceptable format already and if not convert
switch (format) {
case SVDLIBC_DENSE_BINARY:
case SVDLIBC_DENSE_TEXT:
case SVDLIBC_SPARSE_TEXT:
case SVDLIBC_SPARSE_BINARY:
converted = matrix;
break;
default:
SVD_LOGGER.fine("converting input matrix format " +
"from" + format + " to SVDLIBC" +
" ready format");
converted = MatrixIO.convertFormat(
matrix, format, Format.SVDLIBC_SPARSE_BINARY);
format = Format.SVDLIBC_SPARSE_BINARY;
break;
}
return svdlibc(converted, dimensions, format);
} catch (UnsupportedOperationException uoe) { }
}
else {
// Default to SVDLIBJ as all remaining algorithms will be
// slower
return SvdlibjDriver.svd(matrix, format, dimensions);
}
}
}
catch (IOException ioe) {
SVD_LOGGER.log(Level.SEVERE, "convertFormat", ioe);
}
// required for compilation
throw new UnsupportedOperationException("Unknown algorithm: " + alg);
}
/**
* Returns {@code true} if the JAMA library is available
*/
private static boolean isJAMAavailable() {
try {
Class> clazz = loadJamaMatrixClass();
} catch (ClassNotFoundException cnfe) {
return false;
}
return true;
}
/**
* Reflectively loads the JAMA Matrix class. If the class is not
* immediately loadable from the existing classpath, then this method will
* attempt to use the {@code jama.path} system environment variable to load
* it from an external resource.
*/
private static Class> loadJamaMatrixClass()
throws ClassNotFoundException {
try {
Class> clazz = Class.forName("Jama.Matrix");
return clazz;
} catch (ClassNotFoundException cnfe) {
// If we see a CNFE, don't immediately give up. It's most likely
// that this class is being invoked from inside a .jar, so see if
// the user specified where JAMA is manually.
String jamaProp = System.getProperty("jama.path");
// if not, rethrow since the class does not exist
if (jamaProp == null)
throw cnfe;
File jamaJarFile = new File(jamaProp);
try {
// Otherwise, try to load the class from the specified .jar file
java.net.URLClassLoader classLoader =
new java.net.URLClassLoader(
new java.net.URL[] { jamaJarFile.toURI().toURL() });
Class> clazz = Class.forName("Jama.Matrix", true,
classLoader);
return clazz;
} catch (Exception e) {
// fall through and rethrow original
}
throw cnfe;
}
}
/**
* Returns {@code true} if the COLT library is available
*/
private static boolean isColtAvailable() {
try {
// Try loading just the sparse matrix class, as if it is there, the
// other matrix classes will be there as well
Class> clazz = loadColtSparseMatrixClass();
} catch (ClassNotFoundException cnfe) {
return false;
}
return true;
}
/**
* Reflectively loads the COLT {@code SparseDoubleMatrix2D} class.
*/
private static Class> loadColtSparseMatrixClass()
throws ClassNotFoundException {
String coltMatrixClassName =
"cern.colt.matrix.impl.SparseDoubleMatrix2D";
return loadColtClass(coltMatrixClassName);
}
/**
* Reflectively loads the COLT {@code DenseDoubleMatrix2D} class.
*/
private static Class> loadColtDenseMatrixClass()
throws ClassNotFoundException {
String coltMatrixClassName =
"cern.colt.matrix.impl.DenseDoubleMatrix2D";
return loadColtClass(coltMatrixClassName);
}
/**
* Reflectively loads the COLT {@code SingularValueDecompositoin} class.
*/
private static Class> loadColtSVDClass()
throws ClassNotFoundException {
String coltSVDClassName =
"cern.colt.matrix.linalg.SingularValueDecomposition";
return loadColtClass(coltSVDClassName);
}
/**
* Reflectively loads the COLT-related class. If the class is not
* immediately loadable from the existing classpath, then this method will
* attempt to use the {@code colt.path} system environment variable to load
* it from an external resource.
*/
private static Class> loadColtClass(String className)
throws ClassNotFoundException {
try {
Class> clazz =
Class.forName(className);
return clazz;
} catch (ClassNotFoundException cnfe) {
// If we see a CNFE, don't immediately give up. It's most likely
// that this class is being invoked from inside a .jar, so see if
// the user specified where COLT is manually.
String coltProp = System.getProperty("colt.path");
// if not, rethrow since the class does not exist
if (coltProp == null)
throw cnfe;
File coltJarFile = new File(coltProp);
try {
// Otherwise, try to load the class from the specified .jar
// file. If the ClassLoader for loading all COLT classes has
// not yet been initialized, do so now. We need to maintain
// only one class loader for all COLT classes, otherwise the
// reflective invocation will fail; the constructor type system
// does not work correctly if the parameter objects have been
// loaded from a different class loader. --jurgens
if (coltClassLoader == null) {
coltClassLoader =
new java.net.URLClassLoader(
new java.net.URL[] { coltJarFile.toURI().toURL() });
}
Class> clazz = Class.forName(className, true,
coltClassLoader);
return clazz;
} catch (Exception e) {
// fall through and rethrow original
}
throw cnfe;
}
}
// A private static field that is only initialized if COLT is used and the
// classes are not available using the default class loader. Note that this
// field was intentionally put here to indicate where it was initialized in
// the method above, and to prevent developer confusion about why the SVD
// class would even need a custom class loader
private static java.net.URLClassLoader coltClassLoader = null;
/**
* Returns {@code true} if the SVDLIBC library is available
*/
public static boolean isSVDLIBCavailable() {
try {
Process svdlibc = Runtime.getRuntime().exec("svd");
svdlibc.waitFor();
} catch (Exception e) {
return false;
}
return true;
}
/**
* Returns {@code true} if Octave is available
*/
public static boolean isOctaveAvailable() {
try {
Process octave = Runtime.getRuntime().exec("octave -v");
octave.waitFor();
} catch (Exception e) {
return false;
}
return true;
}
/**
* Returns {@code true} if Matlab is available
*/
public static boolean isMatlabAvailable() {
try {
Process matlab = Runtime.getRuntime().exec("matlab -h");
matlab.waitFor();
} catch (Exception ioe) {
return false;
}
return true;
}
/**
* Computes the SVD using Jama.
*
* @param inputMatrix a 2D double array representation of the matrix for
* which the SVD should be computed
* @param dimensions the number of singular values to calculate
*
* @return an array of {@code Matrix} objects for the U, S, and V matrices
* in that order
*
* @throws UnsupportedOperationException if the JAMA SVD algorithm is
* unavailable or if any error occurs during the process
*/
static Matrix[] jamaSVD(double[][] inputMatrix, int dimensions) {
// Use reflection to load the JAMA classes and perform all the
// operation in order to avoid any compile-time dependencies on the
// package.
try {
SVD_LOGGER.fine("attempting JAMA");
isJAMAavailable();
int rows = inputMatrix.length;
int cols = inputMatrix[0].length; // assume at least one row
Class> clazz = loadJamaMatrixClass();
Constructor> c = clazz.getConstructor(double[][].class);
Object jamaMatrix =
c.newInstance(new Object[] { inputMatrix } );
Method svdMethod = clazz.getMethod("svd", new Class[] {});
Object svdObject = svdMethod.invoke(jamaMatrix, new Object[] {});
// covert the JAMA u,s,v matrices to our matrices
String[] matrixMethods = new String[] {"getU", "getS", "getV"};
String[] matrixNames = new String[] {"JAMA-U", "JAMA-S", "JAMA-V"};
Matrix[] usv = new Matrix[3];
// Loop to avoid repeating reflection code
for (int i = 0; i < 3; ++i) {
Method matrixAccessMethod = svdObject.getClass().
getMethod(matrixMethods[i], new Class[] {});
Object matrixObject = matrixAccessMethod.invoke(
svdObject, new Object[] {});
Method toArrayMethod = matrixObject.getClass().
getMethod("getArray", new Class[] {});
double[][] matrixArray = (double[][])(toArrayMethod.
invoke(matrixObject, new Object[] {}));
// JAMA computes the full SVD, so the output matrices need to be
// truncated to the desired number of dimensions
resize:
switch (i) {
case 0: { // U array
Matrix u = Matrices.create(rows, dimensions,
Type.DENSE_IN_MEMORY);
// fill the U matrix by copying over the values
for (int row = 0; row < rows; ++row) {
for (int col = 0; col < dimensions; ++col) {
u.set(row, col, matrixArray[row][col]);
}
}
usv[i] = u;
break resize;
}
case 1: { // S array
// special case for the diagonal matrix
Matrix s = new DiagonalMatrix(dimensions);
for (int diag = 0; diag < dimensions; ++diag) {
s.set(diag, diag, matrixArray[diag][diag]);
}
usv[i] = s;
break resize;
}
case 2: { // V array
// create it on disk since it's not expected that people
// will access this matrix
Matrix v = Matrices.create(dimensions, cols,
Type.DENSE_ON_DISK);
// Fill the V matrix by copying over the values. Note that
// we manually transpose the matrix because JAMA returns the
// result transposed from what we specify.
for (int row = 0; row < dimensions; ++row) {
for (int col = 0; col < cols; ++col) {
v.set(row, col, matrixArray[col][row]);
}
}
usv[i] = v;
}
}
}
return usv;
} catch (ClassNotFoundException cnfe) {
SVD_LOGGER.log(Level.SEVERE, "JAMA", cnfe);
} catch (NoSuchMethodException nsme) {
SVD_LOGGER.log(Level.SEVERE, "JAMA", nsme);
} catch (InstantiationException ie) {
SVD_LOGGER.log(Level.SEVERE, "JAMA", ie);
} catch (IllegalAccessException iae) {
SVD_LOGGER.log(Level.SEVERE, "JAMA", iae);
} catch (InvocationTargetException ite) {
SVD_LOGGER.log(Level.SEVERE, "JAMA", ite);
}
throw new UnsupportedOperationException(
"JAMA-based SVD is not available on this system");
}
/**
* Computes the SVD using COLT.
*
* @param inputMatrix a 2D double array representation of the matrix for
* which the SVD should be computed
* @param isDense {@code true} if the data contained in the double array is
* mostly non-zero. This paramater affects how COLT performs the SVD
* calcuation internally
* @param dimensions the number of singular values to calculate
*
* @return an array of {@code Matrix} objects for the U, S, and V matrices
* in that order
*
* @throws UnsupportedOperationException if the COLT SVD algorithm is
* unavailable or if any error occurs during the process
*/
static Matrix[] coltSVD(double[][] inputMatrix, boolean isDense,
int dimensions) {
// Use reflection to load the COLT classes and perform all the
// operation in order to avoid any compile-time dependencies on the
// package.
try {
SVD_LOGGER.fine("attempting COLT");
int rows = inputMatrix.length;
int cols = inputMatrix[0].length; // assume at least one row
// COLT provides both a sparse and dense Matrix implementation.
// Estimate which one would be more efficient based on the format
// type.
Class> clazz = (isDense)
? loadColtDenseMatrixClass()
: loadColtSparseMatrixClass();
Constructor> c = clazz.getConstructor(double[][].class);
// create the COLT matrix using the java 2D array as values. Note
// that these values are automatically copied, which makes them
// present in memory at the same time. This could be particulary
// inefficient if the values are also in memory elsewhere when they
// were used to write to disk.
//
// A possible option would be determine the matrix dimension but
// still keep the values on disk, then read the values off the disk.
// This would be slower, but may present a large memory savings -
// especially for sparse matrices. Keeping values on disk should be
// considered if it is later discovered that this method presents a
// performance bottleneck for COLT users. --jurgens 7/7/09
Object coltMatrix =
c.newInstance(new Object[] { inputMatrix } );
// One we are done with the inputMatrix, null it out to free up
// memory if this method was the only thread referencing it
inputMatrix = null;
Class> svdClass = loadColtSVDClass();
// Load the base class of both matrix classes for the constructor.
// We need this class for looking up the correct type for the SVD
// class constructor.
Class> matrixBaseClass = loadColtClass(
"cern.colt.matrix.DoubleMatrix2D");
// Load the constructor that takes in a DoubleMatrix2D
Constructor> svdConstructor =
svdClass.getConstructor(matrixBaseClass);
// Compute the full SVD of the matrix
Object svdObject =
svdConstructor.newInstance(coltMatrix);
Matrix[] usv = new Matrix[3];
// covert the COLT u,s,v matrices to our matrices
String[] matrixMethods = new String[] {"getU", "getS", "getV"};
String[] matrixNames = new String[] {"COLT-U", "COLT-S", "COLT-V"};
// Loop to avoid repeating reflection boilerplate code
for (int i = 0; i < 3; ++i) {
Method matrixAccessMethod = svdObject.getClass().
getMethod(matrixMethods[i], new Class[] {});
Object matrixObject = matrixAccessMethod.invoke(
svdObject, new Object[] {});
Method toArrayMethod = matrixObject.getClass().
getMethod("toArray", new Class[] {});
// COLT computes the full SVD, so the output matrices need to be
// truncated to the desired number of dimensions
resize:
switch (i) {
case 0: { // U array
// get the full array
double[][] matrixArray = (double[][])(toArrayMethod.
invoke(matrixObject, new Object[] {}));
Matrix u = Matrices.create(rows, dimensions,
Type.DENSE_IN_MEMORY);
// fill the U matrix by copying over the values
for (int row = 0; row < rows; ++row) {
for (int col = 0; col < dimensions; ++col) {
u.set(row, col, matrixArray[row][col]);
}
}
usv[i] = u;
break resize;
}
case 1: { // S array
// Special case for the diagonal matrix. Unlike U and V, it
// would be a giant waste to load in the full 2D array for a
// diagonal matrix. Therefore just reflectively use the
// matrix accessors to save memory.
Matrix s = new DiagonalMatrix(dimensions);
Method get = matrixObject.getClass().
getMethod("get", new Class[] {Integer.TYPE,
Integer.TYPE});
for (int diag = 0; diag < dimensions; ++diag) {
double value = ((Double)(get.invoke(matrixObject,
new Object[] {Integer.valueOf(diag),
Integer.valueOf(diag) }))).doubleValue();
s.set(diag, diag, value);
}
usv[i] = s;
break resize;
}
case 2: { // V array
// get the full array
double[][] matrixArray = (double[][])(toArrayMethod.
invoke(matrixObject, new Object[] {}));
// create it on disk since it's not expected that people
// will access this matrix
Matrix v = Matrices.create(dimensions, cols,
Type.DENSE_ON_DISK);
// Fill the V matrix by copying over the values.
for (int row = 0; row < dimensions; ++row) {
for (int col = 0; col < cols; ++col) {
v.set(row, col, matrixArray[row][col]);
}
}
usv[i] = v;
}
}
}
return usv;
} catch (ClassNotFoundException cnfe) {
SVD_LOGGER.log(Level.SEVERE, "COLT", cnfe);
} catch (NoSuchMethodException nsme) {
SVD_LOGGER.log(Level.SEVERE, "COLT", nsme);
} catch (InstantiationException ie) {
SVD_LOGGER.log(Level.SEVERE, "COLT", ie);
} catch (IllegalAccessException iae) {
SVD_LOGGER.log(Level.SEVERE, "COLT", iae);
} catch (InvocationTargetException ite) {
SVD_LOGGER.log(Level.SEVERE, "COLT", ite);
}
throw new UnsupportedOperationException(
"COLT-based SVD is not available on this system");
}
/**
* Computes the SVD using SVDLIBC.
*
* @param matrix a file containing a matrix
* @param dimensions the number of singular values to calculate
*
* @return an array of {@code Matrix} objects for the U, S, and V matrices
* in that order
*
* @throws UnsupportedOperationException if the SVDLIBC SVD algorithm is
* unavailable or if any error occurs during the process
*/
static Matrix[] svdlibc(File matrix, int dimensions, Format format) {
try {
String formatString = "";
// output the correct formatting flags based on the matrix type
switch (format) {
case SVDLIBC_DENSE_BINARY:
formatString = " -r db ";
break;
case SVDLIBC_DENSE_TEXT:
formatString = " -r dt ";
break;
case SVDLIBC_SPARSE_BINARY:
formatString = " -r sb ";
break;
case SVDLIBC_SPARSE_TEXT:
// Do nothing since it's the default format.
break;
default:
throw new UnsupportedOperationException(
"Format type is not accepted");
}
File outputMatrixFile = File.createTempFile("svdlibc", ".dat");
outputMatrixFile.deleteOnExit();
String outputMatrixPrefix = outputMatrixFile.getAbsolutePath();
SVD_LOGGER.fine("creating SVDLIBC factor matrices at: " +
outputMatrixPrefix);
String commandLine = "svd -o " + outputMatrixPrefix + formatString +
" -w dt " + // output is dense binary
" -d " + dimensions + " " + matrix.getAbsolutePath();
SVD_LOGGER.fine(commandLine);
Process svdlibc = Runtime.getRuntime().exec(commandLine);
BufferedReader stdout = new BufferedReader(
new InputStreamReader(svdlibc.getInputStream()));
BufferedReader stderr = new BufferedReader(
new InputStreamReader(svdlibc.getErrorStream()));
StringBuilder output = new StringBuilder("SVDLIBC output:\n");
for (String line = null; (line = stderr.readLine()) != null; ) {
output.append(line).append("\n");
}
SVD_LOGGER.fine(output.toString());
int exitStatus = svdlibc.waitFor();
SVD_LOGGER.fine("svdlibc exit status: " + exitStatus);
// If SVDLIBC was successful in generating the files, return them.
if (exitStatus == 0) {
File Ut = new File(outputMatrixPrefix + "-Ut");
File S = new File(outputMatrixPrefix + "-S");
File Vt = new File(outputMatrixPrefix + "-Vt");
// SVDLIBC returns the matrices in U', S, V' with U and V of
// transposed. To ensure consistence, transpose the U matrix
return new Matrix[] {
// load U in memory, since that is what most algorithms will
// be using (i.e. it is the word space). SVDLIBC returns
// this as U transpose, so correct it by indicating that the
// read operation should transpose the matrix as it is built
MatrixIO.readMatrix(Ut, Format.SVDLIBC_DENSE_TEXT,
Type.DENSE_IN_MEMORY, true),
// Sigma only has n values for an n^2 matrix, so make it
// sparse. Note that even if we specify the output to be in
// dense binary, the signular vectors are still reported as
// text
readSVDLIBCsingularVector(S),
// V could be large, so just keep it on disk.
MatrixIO.readMatrix(Vt, Format.SVDLIBC_DENSE_TEXT,
Type.DENSE_ON_DISK)
};
}
else {
StringBuilder sb = new StringBuilder();
for (String line = null; (line = stderr.readLine()) != null; ) {
sb.append(line).append("\n");
}
// warning or error?
SVD_LOGGER.warning("svdlibc exited with error status. " +
"stderr:\n" + sb.toString());
}
} catch (IOException ioe) {
SVD_LOGGER.log(Level.SEVERE, "SVDLIBC", ioe);
} catch (InterruptedException ie) {
SVD_LOGGER.log(Level.SEVERE, "SVDLIBC", ie);
}
throw new UnsupportedOperationException(
"SVDLIBC is not correctly installed on this system");
}
/**
* Generates a diagonal {@link Matrix} from the special-case file format
* that SVDLIBC uses to output the Σ matrix.
*/
private static Matrix readSVDLIBCsingularVector(File sigmaMatrixFile)
throws IOException {
BufferedReader br =
new BufferedReader(new FileReader(sigmaMatrixFile));
int dimension = -1;
int valsSeen = 0;
Matrix m = null;
for (String line = null; (line = br.readLine()) != null; ) {
String[] vals = line.split("\\s+");
for (int i = 0; i < vals.length; ++i) {
// the first value seen should be the number of singular values
if (dimension == -1) {
dimension = Integer.parseInt(vals[i]);
m = new DiagonalMatrix(dimension);
}
else {
m.set(valsSeen, valsSeen, Double.parseDouble(vals[i]));
++valsSeen;
}
}
}
return m;
}
/**
* Computes the SVD using Matlab.
*
* @param matrix a file containing a matrix
* @param dimensions the number of singular values to calculate
*
* @return an array of {@code Matrix} objects for the U, S, and V matrices
* in that order
*
* @throws UnsupportedOperationException if the Matlab SVD algorithm is
* unavailable or if any error occurs during the process
*/
static Matrix[] matlabSVDS(File matrix, int dimensions) {
try {
// create the matlab file for executing
File uOutput = File.createTempFile("matlab-svds-U",".dat");
File sOutput = File.createTempFile("matlab-svds-S",".dat");
File vOutput = File.createTempFile("matlab-svds-V",".dat");
if (SVD_LOGGER.isLoggable(Level.FINE)) {
SVD_LOGGER.fine("writing Matlab output to files:\n" +
" " + uOutput + "\n" +
" " + sOutput + "\n" +
" " + vOutput + "\n");
}
uOutput.deleteOnExit();
sOutput.deleteOnExit();
vOutput.deleteOnExit();
String commandLine = "matlab -nodisplay -nosplash -nojvm";
SVD_LOGGER.fine(commandLine);
Process matlab = Runtime.getRuntime().exec(commandLine);
// capture the input so we know then Matlab is finished
BufferedReader br = new BufferedReader(
new InputStreamReader(matlab.getInputStream()));
// pipe Matlab the program to execute
PrintWriter pw = new PrintWriter(matlab.getOutputStream());
pw.println(
"Z = load('" + matrix.getAbsolutePath() + "','-ascii');\n" +
"A = spconvert(Z);\n" +
"% Remove the raw data file to save space\n" +
"clear Z;\n" +
"[U, S, V] = svds(A, " + dimensions + " );\n" +
"save " + uOutput.getAbsolutePath() + " U -ASCII\n" +
"save " + sOutput.getAbsolutePath() + " S -ASCII\n" +
"save " + vOutput.getAbsolutePath() + " V -ASCII\n" +
"fprintf('Matlab Finished\\n');");
pw.close();
// capture the output
StringBuilder output = new StringBuilder("Matlab svds output:\n");
for (String line = null; (line = br.readLine()) != null; ) {
output.append(line).append("\n");
if (line.equals("Matlab Finished")) {
matlab.destroy();
}
}
SVD_LOGGER.fine(output.toString());
int exitStatus = matlab.waitFor();
SVD_LOGGER.fine("Matlab svds exit status: " + exitStatus);
// If Matlab was successful in generating the files, return them.
if (exitStatus == 0) {
// Matlab returns the matrices in U, S, V, with none of
// transposed. To ensure consistence, transpose the V matrix
return new Matrix[] {
// load U in memory, since that is what most algorithms will be
// using (i.e. it is the word space)
MatrixIO.readMatrix(uOutput, Format.DENSE_TEXT,
Type.DENSE_IN_MEMORY),
// Sigma only has n values for an n^2 matrix, so make it sparse
MatrixIO.readMatrix(sOutput, Format.DENSE_TEXT,
Type.SPARSE_ON_DISK),
// V could be large, so just keep it on disk. Furthermore,
// Matlab does not transpose V, so transpose it
MatrixIO.readMatrix(vOutput, Format.DENSE_TEXT,
Type.DENSE_ON_DISK, true)
};
}
} catch (IOException ioe) {
SVD_LOGGER.log(Level.SEVERE, "Matlab svds", ioe);
} catch (InterruptedException ie) {
SVD_LOGGER.log(Level.SEVERE, "Matlab svds", ie);
}
throw new UnsupportedOperationException(
"Matlab svds is not correctly installed on this system");
}
/**
* Computes the SVD using Octave.
*
* @param matrix a file containing a matrix
* @param dimensions the number of singular values to calculate
*
* @return an array of {@code Matrix} objects for the U, S, and V matrices
* in that order
*
* @throws UnsupportedOperationException if the Octave SVD algorithm is
* unavailable or if any error occurs during the process
*/
static Matrix[] octaveSVDS(File matrix, int dimensions) {
try {
// create the octave file for executing
File octaveFile = File.createTempFile("octave-svds",".m");
File uOutput = File.createTempFile("octave-svds-U",".dat");
File sOutput = File.createTempFile("octave-svds-S",".dat");
File vOutput = File.createTempFile("octave-svds-V",".dat");
octaveFile.deleteOnExit();
uOutput.deleteOnExit();
sOutput.deleteOnExit();
vOutput.deleteOnExit();
// Print the customized Octave program to a file.
PrintWriter pw = new PrintWriter(octaveFile);
pw.println(
"Z = load('" + matrix.getAbsolutePath() + "','-ascii');\n" +
"A = spconvert(Z);\n" +
"% Remove the raw data file to save space\n" +
"clear Z;\n" +
"[U, S, V] = svds(A, " + dimensions + " );\n" +
"save(\"-ascii\", \"" + uOutput.getAbsolutePath() + "\", \"U\");\n" +
"save(\"-ascii\", \"" + sOutput.getAbsolutePath() + "\", \"S\");\n" +
"save(\"-ascii\", \"" + vOutput.getAbsolutePath() + "\", \"V\");\n" +
"fprintf('Octave Finished\\n');\n");
pw.close();
// build a command line where octave executes the previously
// constructed file
String commandLine = "octave " + octaveFile.getAbsolutePath();
SVD_LOGGER.fine(commandLine);
Process octave = Runtime.getRuntime().exec(commandLine);
BufferedReader br = new BufferedReader(
new InputStreamReader(octave.getInputStream()));
BufferedReader stderr = new BufferedReader(
new InputStreamReader(octave.getErrorStream()));
// capture the output
StringBuilder output = new StringBuilder("Octave svds output:\n");
for (String line = null; (line = br.readLine()) != null; ) {
output.append(line).append("\n");
}
SVD_LOGGER.fine(output.toString());
int exitStatus = octave.waitFor();
SVD_LOGGER.fine("Octave svds exit status: " + exitStatus);
// If Octave was successful in generating the files, return them.
if (exitStatus == 0) {
// Octave returns the matrices in U, S, V, with none of
// transposed. To ensure consistence, transpose the V matrix
return new Matrix[] {
// load U in memory, since that is what most algorithms will be
// using (i.e. it is the word space)
MatrixIO.readMatrix(uOutput, Format.DENSE_TEXT,
Type.DENSE_IN_MEMORY),
// Sigma only has n values for an n^2 matrix, so make it sparse
MatrixIO.readMatrix(sOutput, Format.DENSE_TEXT,
Type.SPARSE_ON_DISK),
// V could be large, so just keep it on disk. Furthermore,
// Octave does not transpose V, so transpose it
MatrixIO.readMatrix(vOutput, Format.DENSE_TEXT,
Type.DENSE_ON_DISK, true)
};
}
else {
StringBuilder sb = new StringBuilder();
for (String line = null; (line = stderr.readLine()) != null; ) {
sb.append(line).append("\n");
}
// warning or error?
SVD_LOGGER.warning("Octave exited with error status. " +
"stderr:\n" + sb.toString());
}
} catch (IOException ioe) {
SVD_LOGGER.log(Level.SEVERE, "Octave svds", ioe);
} catch (InterruptedException ie) {
SVD_LOGGER.log(Level.SEVERE, "Octave svds", ie);
}
throw new UnsupportedOperationException(
"Octave svds is not correctly installed on this system");
}
}