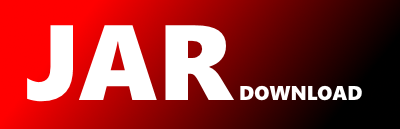
examples.stats.example3.example.md Maven / Gradle / Ivy
Go to download
Show more of this group Show more artifacts with this name
Show all versions of jstat Show documentation
Show all versions of jstat Show documentation
Java Library for Statistical Analysis.
The newest version!
# Example 6: Goodness of fit of regression line
## Contents
* [Overview](#overview)
* [Goodness of fit of regression line](#goodness_of_fit)
* [Import files](#include_files)
* [The main function](#m_func)
* [Results](#results)
* [Source Code](#source_code)
## Overview
### Goodness of fit of regression line
## Import files
```
package examples.stats.example3;
import optimization.GradientDescent;
import optimization.GDInput;
import utils.DefaultIterativeAlgorithmController;
import utils.IterativeAlgorithmResult;
import datasets.DenseMatrixSet;
import datastructs.RowBuilder;
import datasets.VectorDouble;
import datastructs.RowType;
import maths.errorfunctions.MSEVectorFunction;
import maths.errorfunctions.SSEVectorFunction;
import maths.functions.LinearVectorPolynomial;
import ml.regression.LinearRegressor;
import tech.tablesaw.api.Table;
import utils.ListMaths;
import utils.TableDataSetLoader;
import java.io.File;
import java.io.IOException;
```
## The main function
```
public class Example3 {
public static void main(String[] args)throws IOException {
// load the data
Table dataSet = TableDataSetLoader.loadDataSet(new File("src/main/resources/datasets/car_plant.csv"));
Vector labels = new Vector(dataSet, "Electricity Usage");
Table reducedDataSet = dataSet.removeColumns("Electricity Usage").first(dataSet.rowCount());
DenseMatrixSet denseMatrixSet = new DenseMatrixSet(RowType.Type.DOUBLE_VECTOR, new RowBuilder(), reducedDataSet.rowCount(), 2, 1.0);
denseMatrixSet.setColumn(1, reducedDataSet.doubleColumn(0));
LinearVectorPolynomial hypothesis = new LinearVectorPolynomial(1);
LinearRegressor regressor = new LinearRegressor(hypothesis);
GDInput gdInput = new GDInput();
gdInput.showIterations = false;
gdInput.eta=0.01;
gdInput.errF = new MSEVectorFunction(hypothesis);
gdInput.iterationContorller = new DefaultIterativeAlgorithmController(10000,1.0e-8);
BatchGradientDescent gdSolver = new BatchGradientDescent(gdInput);
IterativeAlgorithmResult result = (IterativeAlgorithmResult) regressor.train(denseMatrixSet, labels, gdSolver);
System.out.println(result);
System.out.println("Intercept: "+hypothesis.getCoeff(0)+" slope: "+hypothesis.getCoeff(1));
// let's see the max error over the dateset
Vector errors = regressor.getErrors(denseMatrixSet, labels);
double maxError = ListMaths.max(errors.getRawData());
System.out.println("Maximum error over dataset: "+maxError);
// let's get an estimate of the error variance.
//The error variance sigma^2 can be estimated by considering the deviations between the observed
//data values y_i and their fitted values \hat(y)_i . Specifically, the sum of squares for error SSE is defined
//to be the sum of the squares of these deviations
Vector yhat = regressor.predict(denseMatrixSet);
double sseError = SSEVectorFunction.error(labels, yhat);
double sigma2_hat = sseError/ (yhat.size()-2);
System.out.println("Estimate of error variance: "+ sigma2_hat);
// interval estimation
double Sxx = ListMaths.sxx(denseMatrixSet.getColumn(1).getRawData());
System.out.println("Estimate of Sxx: "+Sxx);
// standard error for the slope
double se_slope = Math.sqrt(sigma2_hat)/Math.sqrt(Sxx);
System.out.println("Standard error for the slope: "+se_slope);
// t-statistic
double t = hypothesis.getCoeff(1)/se_slope;
System.out.println("t-statistic: "+t);
//The two-sided p-value is calculated as
//p-value = 2 × P(X > 6.37) approx 0
//where the random variable X has a t-distribution with 10 degrees of freedom. This low p-value
//indicates that the null hypothesis is not plausible and so the slope parameter is known to be
//nonzero. In other words, it has been established that the distribution of electricity usage does
//depend on the level of production.
// The proportion of the total variability in the dependent variable y that is accounted for by
// the regression line is given by the coefficient of determination.
// This coefficient takes a value between 0 and 1, and the closer it is to one the smaller is the
// sum of squares for error SSE in relation to
// the sum of squares for regression SSR. Thus, larger values of R^2 tend to indicate that the data
// points are closer to the fitted regression line. Nevertheless, a low
// value of R^2 should not necessarily be interpreted as implying that the fitted regression line is
// not appropriate or is not useful. A fitted regression line may be accurate and informative even
// though a small value of R^2 is obtained because of a large error variance sigma62.
double sst = ListMaths.sse(labels.getRawData());
double r_sqr = 1.0- sseError/sst;
System.out.println("Coefficient of determination: "+r_sqr);
}
}
```
## Results
```
Converged: true
Tolerance: 9.995007266283551E-9
# Threads: 1
Iterations: 7076
Intercept: 0.37857734128519877 slope: 0.5049674670001678
Maximum error over dataset: 0.2780755638140122
Estimate of error variance: 0.02992964035512725
Estimate of Sxx: 4.8723000000000525
Standard error for the slope: 0.07837611613854814
t-statistic: 6.442874333138931
Coefficient of determination: 0.8019860710106865
```
## Source Code
Example3.java
© 2015 - 2025 Weber Informatics LLC | Privacy Policy