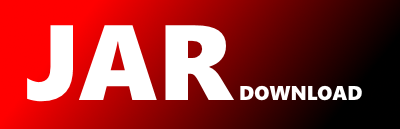
net.maizegenetics.analysis.association.GenomicSelectionPlugin Maven / Gradle / Ivy
Go to download
Show more of this group Show more artifacts with this name
Show all versions of tassel Show documentation
Show all versions of tassel Show documentation
TASSEL is a software package to evaluate traits associations, evolutionary patterns, and linkage
disequilibrium.
package net.maizegenetics.analysis.association;
import net.maizegenetics.stats.linearmodels.FactorModelEffect;
import net.maizegenetics.dna.snp.ExportUtils;
import net.maizegenetics.matrixalgebra.Matrix.DoubleMatrix;
import net.maizegenetics.matrixalgebra.Matrix.DoubleMatrixFactory;
import net.maizegenetics.util.OpenBitSet;
import net.maizegenetics.util.TableReportBuilder;
import net.maizegenetics.phenotype.CategoricalAttribute;
import net.maizegenetics.phenotype.NumericAttribute;
import net.maizegenetics.phenotype.Phenotype;
import net.maizegenetics.phenotype.Phenotype.ATTRIBUTE_TYPE;
import net.maizegenetics.phenotype.PhenotypeAttribute;
import net.maizegenetics.phenotype.PhenotypeBuilder;
import net.maizegenetics.phenotype.PhenotypeUtils;
import net.maizegenetics.phenotype.TaxaAttribute;
import net.maizegenetics.plugindef.AbstractPlugin;
import net.maizegenetics.plugindef.DataSet;
import net.maizegenetics.plugindef.Datum;
import org.apache.log4j.Logger;
import javax.swing.*;
import java.awt.*;
import java.net.URL;
import java.util.ArrayList;
import java.util.Arrays;
import java.util.Collections;
import java.util.Comparator;
import java.util.LinkedList;
import java.util.List;
import java.util.stream.Collectors;
import java.util.stream.IntStream;
import net.maizegenetics.plugindef.PluginParameter;
import net.maizegenetics.stats.EMMA.EMMAforDoubleMatrix;
import net.maizegenetics.stats.linearmodels.BasicShuffler;
import net.maizegenetics.taxa.TaxaList;
import net.maizegenetics.taxa.TaxaListBuilder;
import net.maizegenetics.taxa.TaxaListUtils;
import net.maizegenetics.taxa.Taxon;
import net.maizegenetics.taxa.distance.DistanceMatrix;
import net.maizegenetics.taxa.distance.DistanceMatrixUtils;
import net.maizegenetics.taxa.distance.WriteDistanceMatrix;
import org.apache.commons.math3.stat.correlation.PearsonsCorrelation;
import org.apache.commons.math3.stat.descriptive.DescriptiveStatistics;
public class GenomicSelectionPlugin extends AbstractPlugin {
private static final Logger myLogger = Logger.getLogger(GenomicSelectionPlugin.class);
private PluginParameter performCrossValidation = new
PluginParameter.Builder<>("doCV", true, Boolean.class)
.description("Perform cross-validation: True or False")
.guiName("Perform cross-validation")
.build();
private PluginParameter kFolds = new
PluginParameter.Builder<>("kFolds", 5, Integer.class)
.description("Number of folds to use for k-fold cross-validation (default = 5)")
.guiName("Number of folds")
.dependentOnParameter(performCrossValidation)
.build();
private PluginParameter nIterations = new
PluginParameter.Builder<>("nIter", 20, Integer.class)
.description("Number of iterations when running k-fold cross-validation (default = 20)")
.guiName("Number of iterations")
.dependentOnParameter(performCrossValidation)
.build();
public GenomicSelectionPlugin(Frame parentFrame, boolean isInteractive) {
super(parentFrame, isInteractive);
}
public DataSet processData(DataSet input) {
//extract phenotypes
List myDataList = input.getDataOfType(Phenotype.class);
if (myDataList.size() == 0)
throw new IllegalArgumentException("No phenotype selected.");
if (myDataList.size() > 1)
throw new IllegalArgumentException("Too many phenotypes selected.");
Phenotype myPhenotype = (Phenotype) myDataList.get(0).getData();
String inputPhenotypeName = myDataList.get(0).getName();
//extract kinship matrix
myDataList = input.getDataOfType(DistanceMatrix.class);
if (myDataList.size() == 0)
throw new IllegalArgumentException("No kinship matrix selected.");
if (myDataList.size() > 1)
throw new IllegalArgumentException("Too many kinship matrices selected.");
DistanceMatrix kinship = (DistanceMatrix) myDataList.get(0).getData();
//Remove phenos for which no kinship is present
TaxaList phenoTaxa = myPhenotype.taxa();
TaxaList kinTaxa = kinship.getTaxaList();
TaxaList jointTaxa = TaxaListUtils.getCommonTaxa(phenoTaxa,kinTaxa);
Phenotype reducedPheno = new PhenotypeBuilder()
.fromPhenotype(myPhenotype)
.keepTaxa(jointTaxa)
.build().get(0);
if (performCrossValidation.value()) {
return processDataforCrossValidation(reducedPheno, kinship, inputPhenotypeName);
} else {
return processDataforPrediction(reducedPheno, kinship, inputPhenotypeName);
}
}
public DataSet processDataforPrediction(Phenotype myPhenotype, DistanceMatrix kinshipMatrix, String inputPhenotypeName) {
//create a report builder for the results
String[] columnHeaders = new String[]{"Trait","Taxon","Observed","Predicted","PEV"};
String tableName = "Genomic Prediction Results";
TableReportBuilder myReportBuilder = TableReportBuilder.getInstance(tableName, columnHeaders);
//run the analysis
//Remove kinship for which no pheno is present
List phenoTaxa = myPhenotype.taxaAttribute().allTaxaAsList();
TaxaList phenoTaxaList = new TaxaListBuilder().addAll(phenoTaxa).build();
DistanceMatrix myKinship = DistanceMatrixUtils.keepTaxa(kinshipMatrix,phenoTaxaList);
for (PhenotypeAttribute attr : myPhenotype.attributeListOfType(ATTRIBUTE_TYPE.data)) {
NumericAttribute dataAttribute = (NumericAttribute) attr;
String traitname = dataAttribute.name();
double[] phenotypeData = AssociationUtils.convertFloatArrayToDouble(dataAttribute.floatValues());
int nObs = phenotypeData.length;
DoubleMatrix phenotype = DoubleMatrixFactory.DEFAULT.make(nObs, 1, phenotypeData);
DoubleMatrix fixedEffects = fixedEffectMatrix(myPhenotype);
//Run EMMA using G-BLUP constructor (data, fixed, kinship)
DoubleMatrix kinship = DoubleMatrixFactory.DEFAULT.make(myKinship.getClonedDistances());
EMMAforDoubleMatrix runEMMA = new EMMAforDoubleMatrix(phenotype,fixedEffects,kinship);
runEMMA.setCalculatePEV(true);
runEMMA.solve();
runEMMA.calculateBlupsPredicted();
//report results
//{"Trait","Taxon","Observed","Predicted","PEV"}
TaxaAttribute myTaxa = myPhenotype.taxaAttribute();
DoubleMatrix predictedValues = runEMMA.getPred();
DoubleMatrix pevs = runEMMA.getPev();
for (int obs = 0; obs < nObs; obs++) {
Object[] reportRow = new Object[]{
traitname,
myTaxa.taxon(obs).getName(),
phenotype.get(obs, 0),
predictedValues.get(obs, 0),
pevs.get(obs, 0)};
myReportBuilder.add(reportRow);
}
}
String datumName = "Prediction_" + inputPhenotypeName;
String comment = "Genomic Prediction for " + inputPhenotypeName;
DataSet myReportSet = new DataSet(new Datum(datumName, myReportBuilder.build(), comment), this);
return myReportSet;
}
public DataSet processDataforCrossValidation(Phenotype reducedPheno, DistanceMatrix kinshipOriginal, String inputPhenotypeName) {
DoubleMatrix phenotype;
DoubleMatrix fixedEffects;
//create a report builder for results
String[] columnHeaders = new String[]{"Trait","Iteration","Fold","Accuracy"};
String tableName = "Genomic Prediction Accuracy";
TableReportBuilder myReportBuilder = TableReportBuilder.getInstance(tableName, columnHeaders);
ArrayList commentList = new ArrayList();
//create an index that contains all attributes except the data attributes
//the last index value will be unassigned and will take the value of each data attribute one at a time
int[] taxaAttrIndex = reducedPheno.attributeIndicesOfType(ATTRIBUTE_TYPE.taxa);
int[] dataAttrIndex = reducedPheno.attributeIndicesOfType(ATTRIBUTE_TYPE.data);
int[] factorAttrIndex = reducedPheno.attributeIndicesOfType(ATTRIBUTE_TYPE.factor);
int[] covariateAttrIndex = reducedPheno.attributeIndicesOfType(ATTRIBUTE_TYPE.covariate);
int nAttributes = taxaAttrIndex.length + factorAttrIndex.length + covariateAttrIndex.length + 1;
int[] singlePhenotypeIndex = new int[nAttributes];
int counter = 0;
for (int addIndex : taxaAttrIndex) singlePhenotypeIndex[counter++] = addIndex;
for (int addIndex : factorAttrIndex) singlePhenotypeIndex[counter++] = addIndex;
for (int addIndex : covariateAttrIndex) singlePhenotypeIndex[counter++] = addIndex;
int numberOfIterations = nIterations.value();
int numberOfFolds = kFolds.value();
int numberOfTraits = dataAttrIndex.length;
int numberOfComputes = numberOfIterations * numberOfFolds * numberOfTraits;
int updateProgressValue = Math.max(1, numberOfComputes / 100);
int computeCount = 0;
for (int singleDataIndex : dataAttrIndex) {
singlePhenotypeIndex[nAttributes - 1] = singleDataIndex;
Phenotype singlePhenotype = new PhenotypeBuilder().fromPhenotype(reducedPheno)
.keepAttributes(singlePhenotypeIndex).removeMissingObservations().build().get(0);
NumericAttribute dataAttribute = (NumericAttribute) singlePhenotype.attributeListOfType(ATTRIBUTE_TYPE.data).get(0);
String traitname = dataAttribute.name();
//Remove kinship for which no pheno is present
TaxaList phenoTaxa = singlePhenotype.taxa();
TaxaList kinTaxa = kinshipOriginal.getTaxaList();
TaxaList jointTaxa = TaxaListUtils.getCommonTaxa(phenoTaxa,kinTaxa);
DistanceMatrix myKinship = DistanceMatrixUtils.keepTaxa(kinshipOriginal,jointTaxa);
//get phenotype data
double[] phenotypeData = AssociationUtils.convertFloatArrayToDouble(dataAttribute.floatValues());
int nObs = phenotypeData.length;
phenotype = DoubleMatrixFactory.DEFAULT.make(nObs, 1, phenotypeData);
//debug export -- COMMENT OUT after debugging
// PhenotypeUtils.write(singlePhenotype, String.format("/Users/pbradbury/temp/phenotype_%s_.txt", traitname));
// WriteDistanceMatrix.saveDelimitedDistanceMatrix(myKinship, String.format("/Users/pbradbury/temp/kinship_%s_.txt", traitname));
fixedEffects = fixedEffectMatrix(singlePhenotype);
DoubleMatrix kinship = DoubleMatrixFactory.DEFAULT.make(myKinship.getClonedDistances());
//Create folds for cross-validation
//this division automatically rounds down. feature of java, tested to confirm.
int foldSize = nObs/kFolds.value();
int[] seq = IntStream.range(0,nObs).toArray();
BasicShuffler.reset();//seed is 111 by default within BasicShuffler. This line returns to beginning of that sequence.
double[] rValues = new double[numberOfIterations*numberOfFolds];
int rValueIndex = 0;
//Iterate through number of iterations specified.
for (int iter = 0 ; iter < numberOfIterations ; iter++){
BasicShuffler.shuffle(seq);
//Iterate through folds for cross-validation
int startFold = 0;
for (int fold = 0 ; fold < numberOfFolds ; fold++){
//Set prediction fold to missing
DoubleMatrix phenoTraining = phenotype.copy();
int endFold = startFold + foldSize;
if (fold == numberOfFolds - 1) endFold = nObs;
for (int ndx = startFold ; ndx < endFold ; ndx++){
phenoTraining.set(seq[ndx],0,Double.NaN);
}
//debug -- export masked phenotypes for validation -- COMMENT OUT after testing
// PhenotypeUtils.write(singlePhenotype, String.format("/Users/pbradbury/temp/masked_phenotype_%d:%d_%s_.txt", iter, fold, dataAttribute.name()));
//Run EMMA using G-BLUP constructor (data, fixed, kinship)
EMMAforDoubleMatrix runEMMA = new EMMAforDoubleMatrix(phenoTraining,fixedEffects,kinship);
runEMMA.solve();
runEMMA.calculateBlupsPredicted();
double[] predictions = runEMMA.getPred().to1DArray();
// double[] blups = runEMMA.getBlup().to1DArray(); //debug
int testSize = endFold - startFold;
double[] testPredictions = new double[testSize];
double[] testObserved = new double[testSize];
// double[] testBlups = new double[testSize]; //debug
for (int ndx = 0 ; ndx < testSize ; ndx++){
int seqIndex = seq[ndx + startFold];
testPredictions[ndx] = predictions[seqIndex];
testObserved[ndx] = phenotype.get(seqIndex,0);
// testBlups[ndx] = blups[seqIndex]; //debug
}
PearsonsCorrelation Pearsons = new PearsonsCorrelation();
double rval = Pearsons.correlation(testPredictions,testObserved);
// double blupRval = Pearsons.correlation(testBlups,testObserved); //debug
rValues[rValueIndex++] = rval;
myReportBuilder.add(new Object[]{traitname, new Integer(iter), new Integer(fold), new Double(rval)});
startFold = endFold;
computeCount++;
if (computeCount % updateProgressValue == 0) fireProgress(computeCount/updateProgressValue);
}
}
DescriptiveStatistics stats = new DescriptiveStatistics(rValues);
double meanR = stats.getMean();
double varR = stats.getVariance();
double sdR = Math.sqrt(varR/rValues.length);
System.out.printf("For phenotype %s\n",dataAttribute.name());
System.out.printf("Mean from genomic prediction = %1.4f\n",meanR);
System.out.printf("Standard deviation of mean from genomic prediction = %1.8f\n",sdR);
commentList.add(" ");
commentList.add(String.format("For phenotype %s",dataAttribute.name()));
commentList.add(String.format("Mean from genomic prediction = %1.4f",meanR));
commentList.add(String.format("Standard deviation of mean from genomic prediction = %1.8f",sdR));
}
String comment = "Genomic Prediction Accuracy Summary:\n";
for (String commentLine : commentList) {
comment += commentLine + "\n";
}
DataSet returnData = new DataSet(new Datum("Accuracy_" + inputPhenotypeName,myReportBuilder.build(),comment), this);
fireProgress(100);
return returnData;
}
private DoubleMatrix fixedEffectMatrix(Phenotype aPhenotype) {
List factorAttributeList = aPhenotype.attributeListOfType(ATTRIBUTE_TYPE.factor);
List covariateAttributeList = aPhenotype.attributeListOfType(ATTRIBUTE_TYPE.covariate);
int numberOfFactors = factorAttributeList.size();
int numberOfCovariates = covariateAttributeList.size();
int numberOfEffects = numberOfFactors + numberOfCovariates + 1;
int nObs = aPhenotype.numberOfObservations();
DoubleMatrix fixedEffects;
if (numberOfEffects > 1) {
DoubleMatrix[][] effects = new DoubleMatrix[1][numberOfEffects];
effects[0][0] = DoubleMatrixFactory.DEFAULT.make(nObs, 1, 1);
for (int i = 0; i < numberOfFactors; i++) {
CategoricalAttribute fa = (CategoricalAttribute) factorAttributeList.get(i);
FactorModelEffect fme = new FactorModelEffect(fa.allIntValues(), true);
effects[0][i + 1] = fme.getX();
}
for (int i = 0; i < numberOfCovariates; i++) {
NumericAttribute na = (NumericAttribute) covariateAttributeList.get(i);
double[] values = AssociationUtils.convertFloatArrayToDouble(na.floatValues());
effects[0][i + numberOfFactors + 1] = DoubleMatrixFactory.DEFAULT.make(nObs, 1, values);
}
fixedEffects = DoubleMatrixFactory.DEFAULT.compose(effects);
} else {
fixedEffects = DoubleMatrixFactory.DEFAULT.make(nObs, 1, 1);
}
return fixedEffects;
}
@Override
public ImageIcon getIcon() {
URL imageURL = GenomicSelectionPlugin.class.getResource("/net/maizegenetics/analysis/images/LinearAssociation.gif");
if (imageURL == null) {
return null;
} else {
return new ImageIcon(imageURL);
}
}
@Override
public String getButtonName() {
return "Genomic Selection";
}
@Override
public String getToolTipText() {
return "Predict Phenotypes using G-BLUP for Genomic Selection";
}
@Override
public String pluginDescription() {
return "Predicts phenotypes using G-BLUP for genomic selection using a user-inputted kinship matrix and phenotype(s).";
}
@Override
public String getCitation() {
return "C Diepenbrock, P Bradbury (2015) First Annual Tassel Hackathon";
}
// The following getters and setters were auto-generated.
// Please use this method to re-generate.
//
// public static void main(String[] args) {
// GeneratePluginCode.generate(GenomicSelectionPlugin.class);
// }
/**
* Convenience method to run plugin with one return object.
* @param input
*/
public DataSet runPlugin(DataSet input) {
return (DataSet) performFunction(input).getData(0).getData();
}
/**
* Perform cross-validation: True or False
*
* @return Perform cross-validation
*/
public Boolean performCrossValidation() {
return performCrossValidation.value();
}
/**
* Set Perform cross-validation. Perform cross-validation:
* True or False
*
* @param value Perform cross-validation
*
* @return this plugin
*/
public GenomicSelectionPlugin performCrossValidation(Boolean value) {
performCrossValidation = new PluginParameter<>(performCrossValidation, value);
return this;
}
/**
* Number of folds to use for k-fold cross-validation
* (default = 5)
*
* @return Number of folds
*/
public Integer kFolds() {
return kFolds.value();
}
/**
* Set Number of folds. Number of folds to use for k-fold
* cross-validation (default = 5)
*
* @param value Number of folds
*
* @return this plugin
*/
public GenomicSelectionPlugin kFolds(Integer value) {
kFolds = new PluginParameter<>(kFolds, value);
return this;
}
/**
* Number of iterations when running k-fold cross-validation
* (default = 20)
*
* @return Number of iterations
*/
public Integer nIterations() {
return nIterations.value();
}
/**
* Set Number of iterations. Number of iterations when
* running k-fold cross-validation (default = 20)
*
* @param value Number of iterations
*
* @return this plugin
*/
public GenomicSelectionPlugin nIterations(Integer value) {
nIterations = new PluginParameter<>(nIterations, value);
return this;
}
}
© 2015 - 2025 Weber Informatics LLC | Privacy Policy