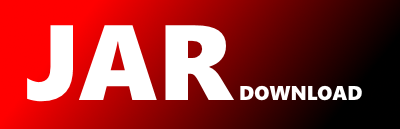
net.maizegenetics.analysis.modelfitter.StepwiseAdditiveModelFitter Maven / Gradle / Ivy
Go to download
Show more of this group Show more artifacts with this name
Show all versions of tassel Show documentation
Show all versions of tassel Show documentation
TASSEL is a software package to evaluate traits associations, evolutionary patterns, and linkage
disequilibrium.
package net.maizegenetics.analysis.modelfitter;
import java.util.ArrayList;
import java.util.Arrays;
import java.util.List;
import java.util.Optional;
import java.util.Spliterator;
import java.util.function.Function;
import java.util.function.Predicate;
import java.util.stream.Collectors;
import java.util.stream.IntStream;
import java.util.stream.Stream;
import java.util.stream.StreamSupport;
import net.maizegenetics.stats.linearmodels.*;
import org.apache.commons.math3.distribution.FDistribution;
import org.apache.log4j.Logger;
import org.apache.log4j.spi.RootLogger;
import net.maizegenetics.analysis.association.AssociationUtils;
import net.maizegenetics.dna.map.Chromosome;
import net.maizegenetics.dna.snp.GenotypeTable;
import net.maizegenetics.matrixalgebra.Matrix.DoubleMatrix;
import net.maizegenetics.matrixalgebra.Matrix.DoubleMatrixFactory;
import net.maizegenetics.matrixalgebra.Matrix.DoubleMatrixFactory.FactoryType;
import net.maizegenetics.phenotype.CategoricalAttribute;
import net.maizegenetics.phenotype.GenotypePhenotype;
import net.maizegenetics.phenotype.NumericAttribute;
import net.maizegenetics.phenotype.Phenotype;
import net.maizegenetics.phenotype.PhenotypeAttribute;
import net.maizegenetics.phenotype.PhenotypeBuilder;
import net.maizegenetics.phenotype.TaxaAttribute;
import net.maizegenetics.phenotype.Phenotype.ATTRIBUTE_TYPE;
import net.maizegenetics.taxa.Taxon;
import net.maizegenetics.util.BitSet;
import net.maizegenetics.util.OpenBitSet;
import net.maizegenetics.util.TableReport;
import net.maizegenetics.util.TableReportBuilder;
/**
* A multi-threaded stepwise model fitter for additive only models. To use it first construct an object then call
* runAnalysis(). After the analysis has run, any of several reports will be available using getters. If a permutation
* test is requested it is run first. The analysis then fits SNPs consecutively,
* then rescans the full model to determine if any of the SNPs has a better choice given all the other
* SNPs in the model. As part of the rescan it calculates a support interval for each of the SNPs. Reports available include
* the steps taken to fit the model with sequential p-values, an anova with marginal p-values, a report of marker effects,
* a post-scan anova, post-scan marker effects, and a permutation report. It will also output a list of model residuals by
* chromosome. For each chromosome, it reports the residuals from a model that includes all effects, except the markers
* on that chromosome.
*/
public class StepwiseAdditiveModelFitter {
//replacement for StepwiseOLSModelFitter that has many of the same features but is not multi-threaded
private static Logger myLogger = RootLogger.getLogger(StepwiseAdditiveModelFitter.class);
protected final GenotypePhenotype myGenoPheno;
protected final GenotypeTable myGenotype;
protected final Phenotype myPhenotype;
protected final List dataAttributeList;
protected final List covariateAttributeList;
protected final List factorAttributeList;
protected final String dataname;
protected double[] y; //data for current phenotype, missing values removed
protected String currentTraitName;
protected List myModel;
protected int numberOfBaseEffects;
protected SweepFastLinearModel mySweepFast;
protected BitSet missing;
protected List mySites;
protected FactorModelEffect nestingFactor;
protected List nestingFactorLevelNames;
protected final double rescanAlpha = 0.05;
protected List allOfTheResidualPhenotypes;
//user defined parameters
protected int numberOfPermutations = 0;
protected double permutationAlpha = 0.05;
protected double enterLimit = 1e-5;
protected double exitLimit = 2e-5;
protected boolean useReferenceProbability = true;
private boolean isNested = true;
private String nestingEffectName = "family";
protected AdditiveSite.CRITERION modelSelectionCriterion = AdditiveSite.CRITERION.pval;
protected int maxSitesInModel = 10;
private boolean useResiduals = false;
protected boolean createAnovaReport = true;
protected boolean createPostScanEffectsReport = true;
protected boolean createPreScanEffectsReport = true;
protected boolean createStepReport = true;
protected boolean createResidualsByChr = false;
//TableReport builders
private final TableReportBuilder anovaReportBuilder =
TableReportBuilder.getInstance("Anova", new String[] { "Trait", "Name", "Chr", "Position",
"df", "MS", "F", "probF", "MarginalRsq" });
private final TableReportBuilder anovaCIReportBuilder =
TableReportBuilder.getInstance("Anova", new String[] { "Trait", "Name", "Chr", "Position",
"df", "MS", "F", "probF", "MarginalRsq", "SuppLeft", "SuppRight" });
protected TableReportBuilder markerEffectReportBuilder =
TableReportBuilder.getInstance("Marker Effects", new String[] { "Trait", "SiteID", "Chr", "Position",
"Within", "Estimate" });
protected TableReportBuilder markerEffectCIReportBuilder =
TableReportBuilder.getInstance("Marker Effects", new String[] { "Trait", "SiteID", "Chr", "Position",
"Within", "Estimate" });
protected final TableReportBuilder permutationReportBuilder =
TableReportBuilder.getInstance("Empirical Null", new String[] { "Trait", "p-value" });
private final TableReportBuilder stepsReportBuilder =
TableReportBuilder.getInstance("Steps", new String[] { "Trait", "SiteID", "Chr", "Position",
"action", "df", "MS", "F", "probF", "AIC", "BIC", "mBIC", "ModelRsq" });
/**
*
* @param genopheno a GenotypePhenotype object
* @param datasetName a name for the genopheno
* @throws IllegalArgumentException if any phenotype data is missing
*/
public StepwiseAdditiveModelFitter(GenotypePhenotype genopheno, String datasetName) {
myGenoPheno = genopheno;
dataname = datasetName;
myGenotype = myGenoPheno.genotypeTable();
myPhenotype = myGenoPheno.phenotype();
//refuse to run if there is missing data for any trait, since that would require rebuilding the site list
Optional missingTest = testPhenotypeForMissingData(myPhenotype);
if (missingTest.isPresent()) {
throw new IllegalArgumentException("Missing data: " + missingTest.get());
}
dataAttributeList = myPhenotype.attributeListOfType(ATTRIBUTE_TYPE.data);
covariateAttributeList = myPhenotype.attributeListOfType(ATTRIBUTE_TYPE.covariate);
factorAttributeList = myPhenotype.attributeListOfType(ATTRIBUTE_TYPE.factor);
}
/**
* @param pheno a Phenotype
* @return true, if there is missing data for any attribute, false otherwise
*/
public Optional testPhenotypeForMissingData(Phenotype pheno) {
int nattr = pheno.numberOfAttributes();
int nobs = pheno.numberOfObservations();
for (int attr = 0; attr < nattr; attr++) {
for (int i = 0; i < nobs; i++) {
if (pheno.isMissing(i, attr)) {
String msg = String.format("Value missing for %s, observation %d", pheno.attribute(attr).name(), i);
return Optional.of(msg);
}
}
}
return Optional.empty();
}
/**
* This is called to run the analysis
*/
public void runAnalysis() {
//load the markers into the appropriate additive site list
if (useReferenceProbability) {
mySites =
IntStream.range(0, myGenotype.numberOfSites())
.mapToObj(s -> {
int ntaxa = myPhenotype.numberOfObservations();
float[] cov = myGenoPheno.referenceProb(s);
return new RefProbAdditiveSite(s, myGenotype.chromosomeName(s), myGenotype.chromosomalPosition(s), myGenotype.siteName(s), modelSelectionCriterion, cov);
})
.collect(Collectors.toList());
} else { // use genotype
mySites =
IntStream.range(0, myGenotype.numberOfSites())
.mapToObj(s -> new GenotypeAdditiveSite(s, myGenotype.chromosomeName(s), myGenotype.chromosomalPosition(s), myGenotype.siteName(s),
modelSelectionCriterion, myGenoPheno.genotypeAllTaxa(s), myGenotype.majorAllele(s), myGenotype.majorAlleleFrequency(s)))
.collect(Collectors.toList());
}
//for each phenotype:
if (createResidualsByChr)
allOfTheResidualPhenotypes = new ArrayList<>();
for (PhenotypeAttribute phenoAttr : dataAttributeList) {
currentTraitName = phenoAttr.name();
//build the base model
List myBaseModel = baseModel(phenoAttr);
myModel = new ArrayList<>(myBaseModel);
numberOfBaseEffects = myModel.size();
if (isNested)
nestingFactor =
(FactorModelEffect) myModel.stream().filter(me -> me.getID().equals(nestingEffectName)).findFirst().get();
//call fitModel()
fitModel();
//add to reports
if (createAnovaReport)
addToAnovaReport(Optional.empty());
if (createPreScanEffectsReport)
addToMarkerEffectReport(false);
//call scanFindCI()
long start = System.nanoTime();
List intervalList = scanToFindCI();
myLogger.info(String.format("Rescan in %d ms", (System.nanoTime() - start) / 1000000));
//created a new scanned model
myModel = new ArrayList<>(myBaseModel);
for (int[] interval : intervalList) {
if (isNested) {
AdditiveSite as = mySites.get(interval[0]);
ModelEffect ncme =
new NestedCovariateModelEffect(as.getCovariate(), nestingFactor);
ncme.setID(as);
myModel.add(ncme);
} else {
AdditiveSite as = mySites.get(interval[0]);
myModel.add(new CovariateModelEffect(as.getCovariate(), as));
}
}
mySweepFast = new SweepFastLinearModel(myModel, y);
//add to reports
if (createAnovaReport)
addToAnovaReport(Optional.of(intervalList));
if (createPostScanEffectsReport)
addToMarkerEffectReport(true);
//scoop up the residuals by chromosome
if (createResidualsByChr)
allOfTheResidualPhenotypes.addAll(generateChromosomeResidualsFromCurrentModel());
}
}
protected void fitModel() {
//run the permutation test, if requested
System.out.println("Running permutation test, if requested.");
long start = System.nanoTime();
DoubleMatrixFactory.setDefault(FactoryType.ejml); //because ejml is faster
if (numberOfPermutations > 0)
runPermutationTest();
myLogger.info(String.format("Permutation test run in %d ms.\n", (System.nanoTime() - start) / 1000000));
//loop through forward-backward steps until the stop criterion is met
Optional lastTermRemoved = Optional.empty();
SweepFastLinearModel sflm = new SweepFastLinearModel(myModel, y);
start = System.nanoTime();
double selectionCriterionValue = 0;
switch (modelSelectionCriterion) {
case pval:
selectionCriterionValue = 1;
break;
case aic:
selectionCriterionValue =
aic(sflm.getResidualSSdf()[0], y.length, sflm.getFullModelSSdf()[1]);
break;
case bic:
selectionCriterionValue =
bic(sflm.getResidualSSdf()[0], y.length, sflm.getFullModelSSdf()[1]);
break;
case mbic:
selectionCriterionValue =
mbic(sflm.getResidualSSdf()[0], y.length, sflm.getFullModelSSdf()[1], mySites.size());
break;
}
while (!Double.isNaN(selectionCriterionValue = forwardStep(selectionCriterionValue))) {
//if the forward step tries to add the term just removed by the backward step, stop fitting terms
if (lastTermRemoved.isPresent()) {
AdditiveSite lastSiteRemoved = (AdditiveSite) lastTermRemoved.get().getID();
AdditiveSite lastSiteAdded = (AdditiveSite) myModel.get(myModel.size() - 1).getID();
if (lastSiteRemoved.siteNumber() == lastSiteAdded.siteNumber())
break;
}
do {
lastTermRemoved = backwardStep();
} while (lastTermRemoved.isPresent());
int numberOfSitesInModel = myModel.size() - numberOfBaseEffects;
if (numberOfSitesInModel >= maxSitesInModel)
break;
}
myLogger.info(String.format("Model fit in %d ms.\n", (System.nanoTime() - start) / 1000000));
}
protected List baseModel(PhenotypeAttribute phenotypeBeingTested) {
//build the base model and y for this phenotype with missing values deleted
List myBaseModel;
missing = new OpenBitSet(phenotypeBeingTested.missing());
for (PhenotypeAttribute pa : covariateAttributeList)
missing.union(pa.missing());
for (PhenotypeAttribute pa : factorAttributeList)
missing.union(pa.missing());
y =
AssociationUtils.getNonMissingDoubles((float[]) phenotypeBeingTested.allValues(), missing);
int numberNotMissing = y.length;
myBaseModel = new ArrayList<>();
int[] mean = new int[numberNotMissing];
ModelEffect me = new FactorModelEffect(mean, false, "mean");
myBaseModel.add(me);
for (PhenotypeAttribute pa : factorAttributeList) {
CategoricalAttribute ca = (CategoricalAttribute) pa;
String[] caLabels = AssociationUtils.getNonMissingValues(ca.allLabels(), missing);
ArrayList factorLabels = new ArrayList<>();
int[] levels = ModelEffectUtils.getIntegerLevels(caLabels, factorLabels);
if (pa.name().equals(nestingEffectName))
nestingFactorLevelNames = factorLabels;
me = new FactorModelEffect(levels, true, pa.name());
myBaseModel.add(me);
}
for (PhenotypeAttribute pa : covariateAttributeList) {
NumericAttribute numAttr = (NumericAttribute) pa;
double[] cov = AssociationUtils.getNonMissingDoubles(numAttr.floatValues(), missing);
me = new CovariateModelEffect(cov, pa.name());
myBaseModel.add(me);
}
return myBaseModel;
}
protected double forwardStep(double prevCriterionValue) {
//do this in parallel
//create a stream returning AdditiveSites that have an ordering; select the max
//criteria can be one of SS, pvalue, aic, bic, mbic (handled by ForwardStepAdditiveSpliterator)
Spliterator siteEvaluator;
if (isNested) {
siteEvaluator =
new ForwardStepNestedAdditiveSpliterator(mySites, myModel, y, nestingFactor);
} else {
siteEvaluator = new ForwardStepAdditiveSpliterator(mySites, myModel, y);
}
Optional bestSite =
StreamSupport.stream(siteEvaluator, true).max((a, b) -> a.compareTo(b));
if (!bestSite.isPresent())
return Double.NaN;
ModelEffect nextEffect;
if (isNested) {
nextEffect =
new NestedCovariateModelEffect(bestSite.get().getCovariate(), nestingFactor);
nextEffect.setID(bestSite.get());
} else {
nextEffect = new CovariateModelEffect(bestSite.get().getCovariate(), bestSite.get());
}
myModel.add(nextEffect);
mySweepFast = new SweepFastLinearModel(myModel, y);
double[] siteSSdf = mySweepFast.getIncrementalSSdf(myModel.size() - 1);
double[] errorSSdf = mySweepFast.getResidualSSdf();
double F, p;
F = siteSSdf[0] / siteSSdf[1] / errorSSdf[0] * errorSSdf[1];
// p = 1 - (new FDistribution(siteSSdf[1], errorSSdf[1]).cumulativeProbability(F));
p = LinearModelUtils.Ftest(F, siteSSdf[1], errorSSdf[1]);
boolean addToModel = false;
double criterionValue = Double.NaN;
switch (modelSelectionCriterion) {
case pval:
criterionValue = p;
if (p < enterLimit)
addToModel = true;
break;
case aic:
criterionValue = aic(errorSSdf[0], y.length, mySweepFast.getFullModelSSdf()[0]);
if (criterionValue < prevCriterionValue)
addToModel = true;
break;
case bic:
criterionValue = bic(errorSSdf[0], y.length, mySweepFast.getFullModelSSdf()[0]);
if (criterionValue < prevCriterionValue)
addToModel = true;
break;
case mbic:
criterionValue =
mbic(errorSSdf[0], y.length, mySweepFast.getFullModelSSdf()[0], mySites.size());
if (criterionValue < prevCriterionValue)
addToModel = true;
break;
}
if (addToModel) {
addToStepsReport(bestSite.get().siteNumber(), mySweepFast, "add", siteSSdf, errorSSdf, F, p);
return criterionValue;
}
addToStepsReport(bestSite.get().siteNumber(), mySweepFast, "stop", siteSSdf, errorSSdf, F, p);
myModel.remove(myModel.size() - 1);
mySweepFast = new SweepFastLinearModel(myModel, y);
return Double.NaN;
}
protected void addToStepsReport(int siteNumber, SweepFastLinearModel theModel, String action, double[] siteSSdf, double[] errorSSdf, double F, double p) {
//add this to the steps report builder which has columns
//"Trait","SiteID","Chr","Position","action","df","MS","F","probF","AIC","BIC","mBIC","ModelRsq"
Object[] row = new Object[13];
int col = 0;
double[] modelSSdf = theModel.getFullModelSSdf();
double[] modelcfmSSdf = theModel.getModelcfmSSdf();
int nsites = mySites.size();
int N = y.length;
row[col++] = currentTraitName;
row[col++] = myGenotype.positions().siteName(siteNumber);
row[col++] = myGenotype.positions().chromosome(siteNumber).getName();
row[col++] = new Integer(myGenotype.positions().get(siteNumber).getPosition());
row[col++] = action;
row[col++] = new Integer((int) siteSSdf[1]);
row[col++] = new Double(siteSSdf[0] / siteSSdf[1]);
row[col++] = new Double(F);
row[col++] = new Double(p);
row[col++] = new Double(aic(errorSSdf[0], N, modelSSdf[1]));
row[col++] = new Double(bic(errorSSdf[0], N, modelSSdf[1]));
row[col++] = new Double(mbic(errorSSdf[0], N, modelSSdf[1], nsites));
row[col++] = new Double(modelcfmSSdf[0] / (modelcfmSSdf[0] + errorSSdf[0]));
stepsReportBuilder.add(row);
myLogger.info(String.format("site %s, action = %s, p = %1.5e\n", myGenotype.positions().siteName(siteNumber), action, p));
}
private Optional backwardStep() {
if (modelSelectionCriterion == AdditiveSite.CRITERION.pval)
return backwardStepPval();
return backwardStepXic();
}
private Optional backwardStepPval() {
int numberOfEffects = myModel.size();
double[] lowestSSdf = new double[] { Double.MAX_VALUE, 0 };
int effectWithLowestSS = -1;
for (int effect = numberOfBaseEffects; effect < numberOfEffects; effect++) {
double[] ssdf = mySweepFast.getMarginalSSdf(effect);
if (ssdf[0] < lowestSSdf[0]) {
lowestSSdf = ssdf;
effectWithLowestSS = effect;
}
}
double[] errorSSdf = mySweepFast.getResidualSSdf();
double F = lowestSSdf[0] / lowestSSdf[1] / errorSSdf[0] * errorSSdf[1];
double p = 1 - (new FDistribution(lowestSSdf[1], errorSSdf[1]).cumulativeProbability(F));
if (p > exitLimit) {
int siteNumber = ((AdditiveSite) myModel.get(effectWithLowestSS).getID()).siteNumber();
addToStepsReport(siteNumber, mySweepFast, "remove", lowestSSdf, errorSSdf, F, p);
ModelEffect removedEffect = myModel.remove(effectWithLowestSS);
mySweepFast = new SweepFastLinearModel(myModel, y);
return Optional.of(removedEffect);
}
return Optional.empty();
}
private Optional backwardStepXic() {
int numberOfParameters = myModel.size();
double lowestVal = Double.MAX_VALUE;
int effectWithLowestVal = -1;
double[] errorSSdf = mySweepFast.getResidualSSdf();
double[] modelSSdf = mySweepFast.getFullModelSSdf();
for (int effect = numberOfBaseEffects; effect < numberOfParameters; effect++) {
double[] margSSdf = mySweepFast.getMarginalSSdf(effect);
double RSS = errorSSdf[0] + margSSdf[0];
double df = modelSSdf[1] - margSSdf[1];
double valReducedModel = Double.MAX_VALUE;
switch (modelSelectionCriterion) {
case aic:
valReducedModel = aic(RSS, y.length, df);
break;
case bic:
valReducedModel = bic(RSS, y.length, df);
break;
case mbic:
valReducedModel = mbic(RSS, y.length, df, mySites.size());
break;
}
if (valReducedModel < lowestVal) {
lowestVal = valReducedModel;
effectWithLowestVal = effect;
}
}
//if the reduced model has an xic value less than the full model it is a better model and should replace the better model
//it will be necessary to keep from readding this term in the next forward step
double valFullModel = Double.MAX_VALUE;
switch (modelSelectionCriterion) {
case aic:
valFullModel = aic(errorSSdf[0], y.length, modelSSdf[1]);
break;
case bic:
valFullModel = bic(errorSSdf[0], y.length, modelSSdf[1]);
break;
case mbic:
valFullModel = mbic(errorSSdf[0], y.length, modelSSdf[1], mySites.size());
break;
}
if (lowestVal < valFullModel) { //remove the offending term
ModelEffect removedEffect = myModel.remove(effectWithLowestVal);
int siteNumber = ((AdditiveSite) myModel.get(effectWithLowestVal).getID()).siteNumber();
double[] siteSSdf = mySweepFast.getMarginalSSdf(effectWithLowestVal);
double F = siteSSdf[0] / siteSSdf[1] / errorSSdf[0] * errorSSdf[1];
double p = 1 - (new FDistribution(siteSSdf[1], errorSSdf[1]).cumulativeProbability(F));
addToStepsReport(siteNumber, mySweepFast, "remove", siteSSdf, errorSSdf, F, p);
mySweepFast = new SweepFastLinearModel(myModel, y);
return Optional.of(removedEffect);
}
return Optional.empty();
}
protected List scanToFindCI() {
//define an IntFunction that finds interval endpoints
//the interval is bounded by the first points that when added to the model result in the marginal p of the test site <= alpha
Function intervalFinder = me -> {
//scan steps:
//1. find interval end points
//2. determine if any point in the interval gives a better model fit (ssmodel) than the original
//3. if no, return support interval
//4. if yes, replace the original with that point and rescan then return support interval
AdditiveSite scanSite = (AdditiveSite) me.getID();
myLogger.info(String.format("Scanning site %d, %s, pos = %d", scanSite.siteNumber(), myGenotype.chromosome(scanSite.siteNumber()), myGenotype.chromosomalPosition(scanSite.siteNumber())));
int[] support = findCI(me, myModel);
List baseModel = new ArrayList<>(myModel);
baseModel.remove(me);
AdditiveSite bestSite = bestTerm(baseModel, support);
if (!bestSite.equals(scanSite)) {
ModelEffect bestEffect;
if (isNested) {
bestEffect =
new NestedCovariateModelEffect(new CovariateModelEffect(bestSite.getCovariate(), bestSite), nestingFactor);
bestEffect.setID(bestSite);
} else {
bestEffect = new CovariateModelEffect(bestSite.getCovariate(), bestSite);
}
baseModel.add(bestEffect);
support = findCI(bestEffect, baseModel);
}
return support;
};
return myModel.stream().skip(numberOfBaseEffects).parallel().map(intervalFinder).collect(Collectors.toList());
}
protected int[] findCI(ModelEffect me, List theModel) {
AdditiveSite site = (AdditiveSite) me.getID();
int testedSiteNumber = site.siteNumber();
int effectNumber = theModel.indexOf(me);
Chromosome thisChr = myGenotype.positions().chromosome(testedSiteNumber);
int leftndx, rightndx;
leftndx = rightndx = testedSiteNumber;
//make sure site list is an array list for efficient retrieval
ArrayList siteArrayList;
if (mySites instanceof ArrayList)
siteArrayList = (ArrayList) mySites;
else
siteArrayList = new ArrayList<>(mySites);
do {
leftndx--;
if (leftndx == -1 || !myGenotype.positions().chromosome(leftndx).equals(thisChr)) {
leftndx++;
break;
}
} while (testAddedTerm(effectNumber, siteArrayList.get(leftndx), theModel) > rescanAlpha);
do {
rightndx++;
if (rightndx == myGenotype.numberOfSites() || !myGenotype.positions().chromosome(rightndx).equals(thisChr)) {
rightndx--;
break;
}
} while (testAddedTerm(effectNumber, siteArrayList.get(rightndx), theModel) > rescanAlpha);
return new int[] { testedSiteNumber, leftndx, rightndx };
}
protected double testAddedTerm(int testedTerm, AdditiveSite addedTerm, List theModel) {
List testingModel = new ArrayList<>(theModel);
if (isNested) {
NestedCovariateModelEffect ncme =
new NestedCovariateModelEffect(addedTerm.getCovariate(), nestingFactor);
testingModel.add(ncme);
} else {
CovariateModelEffect cme = new CovariateModelEffect(addedTerm.getCovariate());
testingModel.add(cme);
}
SweepFastLinearModel sflm = new SweepFastLinearModel(testingModel, y);
sflm.getResidualSSdf();
double[] residualSSdf = sflm.getResidualSSdf();
double[] marginalSSdf = sflm.getMarginalSSdf(testedTerm);
double F = marginalSSdf[0] / marginalSSdf[1] / residualSSdf[0] * residualSSdf[1];
//debug
double prob = 1;
try {
prob -= (new FDistribution(marginalSSdf[1], residualSSdf[1]).cumulativeProbability(F));
} catch(Exception e) {
//do nothing
}
return prob;
}
protected AdditiveSite bestTerm(List baseModel, int[] interval) {
List intervalList = mySites.subList(interval[1], interval[2]);
PartitionedLinearModel plm =
new PartitionedLinearModel(baseModel, new SweepFastLinearModel(baseModel, y));
if (isNested) {
return intervalList.stream()
.map(s -> {
plm.testNewModelEffect(new NestedCovariateModelEffect(s.getCovariate(), nestingFactor));
s.criterionValue(plm.getModelSS());
return s;
})
.reduce((a, b) -> a.criterionValue() >= b.criterionValue() ? a : b)
.get();
} else {
return intervalList.stream()
.map(s -> {
s.criterionValue(plm.testNewModelEffect(s.getCovariate()));
return s;
})
.reduce((a, b) -> a.criterionValue() >= b.criterionValue() ? a : b)
.get();
}
}
public void runPermutationTest() {
//parallel version of permutation test
int enterLimitIndex = (int) (permutationAlpha * numberOfPermutations); //index of percentile to be used for the enter limit
//create the permutedData
SweepFastLinearModel sflm = new SweepFastLinearModel(myModel, y);
double[] yhat = sflm.getPredictedValues().to1DArray();
double[] residuals = sflm.getResiduals().to1DArray();
BasicShuffler.shuffle(residuals);
List permutedData = Stream.iterate(residuals, BasicShuffler.shuffleDouble())
.limit(numberOfPermutations)
.map(a -> {
double[] permutedValues = Arrays.copyOf(a, a.length);
for (int i = 0; i < a.length; i++)
permutedValues[i] += yhat[i];
return permutedValues;
})
.collect(Collectors.toList());
//find the minimum p values for each site
double[] maxP = new double[numberOfPermutations];
Arrays.fill(maxP, 1.0);
double[] minP;
List plist = new ArrayList<>();
if (isNested) {
ModelEffect nestWithin =
myModel.stream().filter(me -> nestingEffectName.equals(me.getID())).findFirst().get();
minP =
StreamSupport.stream(new NestedCovariatePermutationTestSpliterator(permutedData, mySites, myModel, nestWithin), true)
.peek(mp -> plist.add(mp))
.reduce(maxP, (a, b) -> {
int n = a.length;
for (int i = 0; i < n; i++) {
if (a[i] > b[i])
a[i] = b[i];
}
return a;
});
} else {
minP =
StreamSupport.stream(new CovariatePermutationTestSpliterator(permutedData, mySites, myModel), true).reduce(maxP, (a, b) -> {
int n = a.length;
for (int i = 0; i < n; i++) {
if (a[i] > b[i])
a[i] = b[i];
}
return a;
});
}
Arrays.sort(minP);
enterLimit = minP[enterLimitIndex];
exitLimit = 2 * enterLimit;
myLogger.info(String.format("Permutation results for %s: enterLimit = %1.5e, exitLimit = %1.5e\n", currentTraitName, enterLimit, exitLimit));
//add values to permutation report : "Trait","p-value"
Arrays.stream(minP).forEach(d -> permutationReportBuilder.add(new Object[] {
currentTraitName, new Double(d) }));
}
private List generateChromosomeResidualsFromCurrentModel() {
List chrResidualPhenotypeList = new ArrayList<>();
List attributes = new ArrayList<>();
List types = new ArrayList<>();
Taxon[] allTaxa = myPhenotype.taxaAttribute().allTaxa();
Taxon[] nonmissingTaxa = AssociationUtils.getNonMissingValues(allTaxa, missing);
attributes.add(new TaxaAttribute(Arrays.asList(nonmissingTaxa)));
types.add(ATTRIBUTE_TYPE.taxa);
//this next step will include family in the return Phenotype
//any covariates will not be included
for (PhenotypeAttribute factor : factorAttributeList) {
String[] values = ((CategoricalAttribute) factor).allLabels();
attributes.add(new CategoricalAttribute(factor.name(), AssociationUtils.getNonMissingValues(values, missing)));
types.add(ATTRIBUTE_TYPE.factor);
}
//How many chromosomes in the data?
Chromosome[] myChromosomes = myGenotype.chromosomes();
for (Chromosome chr : myChromosomes) {
myLogger.info(String.format("Calculating residuals for %s, %s", chr.getName(), currentTraitName));
List chrAttributes = new ArrayList<>(attributes);
List chrTypes = new ArrayList<>(types);
String traitname =
String.format("%s_chr_%s", currentTraitName, chr.getName());
//create a model without this chromosome
Predicate notInChr = me -> {
if (me.getID() instanceof AdditiveSite) {
int siteNumber = ((AdditiveSite) me.getID()).siteNumber();
return !chr.equals(myGenotype.positions().chromosome(siteNumber));
}
return true;
};
List chrModel = myModel.stream()
.filter(notInChr)
.collect(Collectors.toList());
SweepFastLinearModel sflm = new SweepFastLinearModel(chrModel, y);
//add the residuals to the Phenotype
DoubleMatrix resid = sflm.getResiduals();
float[] data = AssociationUtils.convertDoubleArrayToFloat(resid.to1DArray());
chrAttributes.add(new NumericAttribute(traitname, data, new OpenBitSet(data.length)));
chrTypes.add(ATTRIBUTE_TYPE.data);
chrResidualPhenotypeList.add(new PhenotypeBuilder().fromAttributeList(chrAttributes, chrTypes).build().get(0));
}
return chrResidualPhenotypeList;
}
protected void addToAnovaReport(Optional> intervalList) {
//which has header: "Trait","Name","Chr","Position","df","MS","F","probF","MarginalRsq"
//CI header: "Trait","Name","Chr","Position","df","MS","F","probF","MarginalRsq","SuppLeft","SuppRight"
double[] errorSSdf = mySweepFast.getResidualSSdf();
double errorMS = errorSSdf[0] / errorSSdf[1];
double[] modelcfmSSdf = mySweepFast.getModelcfmSSdf();
double totalSScfm = modelcfmSSdf[0] + errorSSdf[0];
for (int i = 0; i < myModel.size(); i++) {
ModelEffect me = myModel.get(i);
Object[] row;
if (intervalList.isPresent())
row = new Object[11];
else
row = new Object[9];
int col = 0;
row[col++] = currentTraitName;
Object id = me.getID();
if (id instanceof AdditiveSite) {
AdditiveSite as = (AdditiveSite) id;
row[col++] = myGenotype.positions().siteName(as.siteNumber());
row[col++] = myGenotype.positions().chromosome(as.siteNumber());
row[col++] = new Integer(myGenotype.positions().get(as.siteNumber()).getPosition());
} else {
row[col++] = id.toString();
row[col++] = "--";
row[col++] = "--";
}
double[] ssdf = mySweepFast.getMarginalSSdf(i);
double F = ssdf[0] / ssdf[1] / errorMS;
double p;
try {
p = LinearModelUtils.Ftest(F, ssdf[1], errorSSdf[1]);
} catch (Exception e) {
p = Double.NaN;
}
row[col++] = new Integer((int) ssdf[1]);
row[col++] = new Double(ssdf[0] / ssdf[1]);
row[col++] = new Double(F);
row[col++] = new Double(p);
row[col++] = new Double(ssdf[0] / totalSScfm);
if (intervalList.isPresent()) {
if (i >= numberOfBaseEffects) {
int markerNumber = i - numberOfBaseEffects;
int[] interval = intervalList.get().get(markerNumber);
row[col++] = new Integer(myGenotype.positions().get(interval[1]).getPosition());
row[col++] = new Integer(myGenotype.positions().get(interval[2]).getPosition());
} else {
row[col++] = "--";
row[col++] = "--";
}
anovaCIReportBuilder.add(row);
} else {
anovaReportBuilder.add(row);
}
}
//add a row for the error:
Object[] row;
if (intervalList.isPresent())
row = new Object[11];
else
row = new Object[9];
int col = 0;
row[col++] = currentTraitName;
row[col++] = "Error";
row[col++] = "--";
row[col++] = "--";
row[col++] = new Integer((int) errorSSdf[1]);
row[col++] = new Double(errorMS);
row[col++] = "--";
row[col++] = "--";
row[col++] = "--";
if (intervalList.isPresent()) {
row[col++] = "--";
row[col++] = "--";
anovaCIReportBuilder.add(row);
} else {
anovaReportBuilder.add(row);
}
}
protected void addToMarkerEffectReport(boolean CI) {
//header: "Trait","SiteID","Chr","Position","Within","Estimate"
double[] beta = mySweepFast.getBeta();
int numberOfEffects = myModel.size();
if (isNested) {
int numberOfMarkers = numberOfEffects - numberOfBaseEffects;
int numberOfFactorLevels = nestingFactor.getNumberOfLevels();
int numberOfMarkerEstimates = numberOfMarkers * numberOfFactorLevels;
int estCounter = beta.length - numberOfMarkerEstimates;
for (int m = 0; m < numberOfMarkers; m++) {
int site =
((AdditiveSite) myModel.get(numberOfBaseEffects + m).getID()).siteNumber();
String siteID = myGenotype.siteName(site);
String chr = myGenotype.positions().chromosomeName(site);
Integer pos = myGenotype.positions().get(site).getPosition();
for (int f = 0; f < numberOfFactorLevels; f++) {
Object[] row = new Object[6];
int col = 0;
row[col++] = currentTraitName;
row[col++] = siteID;
row[col++] = chr;
row[col++] = pos;
row[col++] = nestingFactorLevelNames.get(f);
row[col++] = new Double(beta[estCounter++]);
if (CI)
markerEffectCIReportBuilder.add(row);
else
markerEffectReportBuilder.add(row);
}
}
} else {
int numberOfMarkers = numberOfEffects - numberOfBaseEffects;
int firstMarker = beta.length - numberOfMarkers;
IntStream.range(0, numberOfMarkers).forEach(m -> {
Object[] row = new Object[6];
int col = 0;
row[col++] = currentTraitName;
int site =
((AdditiveSite) myModel.get(numberOfBaseEffects + m).getID()).siteNumber();
row[col++] = myGenotype.siteName(site);
row[col++] = myGenotype.positions().chromosomeName(site);
row[col++] = myGenotype.positions().get(site).getPosition();
row[col++] = "--";
row[col++] = new Double(beta[firstMarker + m]);
if (CI)
markerEffectCIReportBuilder.add(row);
else
markerEffectReportBuilder.add(row);
});
}
}
public void numberOfPermutations(int nperm) {
numberOfPermutations = nperm;
}
public void permutationAlpha(double alpha) {
permutationAlpha = alpha;
}
public void enterLimit(double limit) {
enterLimit = limit;
}
public void exitLimit(double limit) {
exitLimit = limit;
}
public void useReferenceProbability(boolean useRefProb) {
useReferenceProbability = useRefProb;
}
public void isNested(boolean nested) {
isNested = nested;
}
public void nestingEffectName(String factorName) {
nestingEffectName = factorName;
}
public void modelSelectionCriterion(AdditiveSite.CRITERION criterion) {
modelSelectionCriterion = criterion;
}
public void maxSitesInModel(int maxSites) {
maxSitesInModel = maxSites;
}
public void useResiduals(boolean useResid) {
useResiduals = useResid;
}
public void createAnovaReport(boolean createIt) {
createAnovaReport = createIt;
}
public void createPostScanEffectsReport(boolean createIt) {
createPostScanEffectsReport = createIt;
}
public void createPreScanEffectsReport(boolean createIt) {
createPreScanEffectsReport = createIt;
}
public void createResidualsByChr(boolean createIt) {
createResidualsByChr = createIt;
}
public void createStepReport(boolean createIt) {
createStepReport = createIt;
}
public TableReport getAnovaReport() {
return anovaReportBuilder.build();
}
public TableReport getAnovaReportWithCI() {
return anovaCIReportBuilder.build();
}
public TableReport getMarkerEffectReport() {
return markerEffectReportBuilder.build();
}
public TableReport getMarkerEffectReportWithCI() {
return markerEffectCIReportBuilder.build();
}
public List getResidualPhenotypesByChromosome() {
return allOfTheResidualPhenotypes;
}
public TableReport getPermutationReport() {
return permutationReportBuilder.build();
}
public TableReport getSteps() {
return stepsReportBuilder.build();
}
public static double aic(double RSS, int N, double modelDf) {
return N * Math.log(RSS / N) + 2 * modelDf;
}
public static double bic(double RSS, int N, double modelDf) {
return N * Math.log(RSS / N) + Math.log(N) * modelDf;
}
public static double mbic(double RSS, int N, double modelDf, int numberOfSites) {
return N * Math.log(RSS) + Math.log(N) * modelDf + 2 * modelDf
* Math.log(numberOfSites / 2.2 - 1);
}
}
© 2015 - 2025 Weber Informatics LLC | Privacy Policy