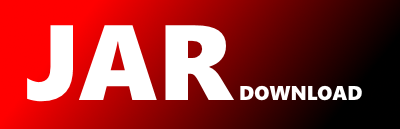
net.maizegenetics.analysis.numericaltransform.ImputationPlugin Maven / Gradle / Ivy
Go to download
Show more of this group Show more artifacts with this name
Show all versions of tassel Show documentation
Show all versions of tassel Show documentation
TASSEL is a software package to evaluate traits associations, evolutionary patterns, and linkage
disequilibrium.
package net.maizegenetics.analysis.numericaltransform;
/*
* Plugin for imputation methods in Numerical Transformations.
* @author - Janu Verma
*/
import net.maizegenetics.dna.snp.GenotypeTable;
import net.maizegenetics.dna.snp.GenotypeTableBuilder;
import net.maizegenetics.dna.snp.score.ReferenceProbabilityBuilder;
import net.maizegenetics.phenotype.Phenotype;
import net.maizegenetics.phenotype.PhenotypeAttribute;
import net.maizegenetics.phenotype.NumericAttribute;
import net.maizegenetics.phenotype.PhenotypeBuilder;
import net.maizegenetics.dna.snp.GenotypeTableUtils;
import net.maizegenetics.plugindef.AbstractPlugin;
import net.maizegenetics.plugindef.DataSet;
import net.maizegenetics.plugindef.Datum;
import net.maizegenetics.plugindef.PluginParameter;
import net.maizegenetics.util.OpenBitSet;
import java.awt.Frame;
import java.util.ArrayList;
import java.util.List;
import org.apache.log4j.Logger;
import javax.swing.*;
public class ImputationPlugin extends AbstractPlugin {
private static final Logger myLogger = Logger.getLogger(ImputationPlugin.class);
private enum distanceChoice {
Euclidean, Manhattan, Cosine
}
private PluginParameter byMean = new PluginParameter.Builder<>("ByMean", false, Boolean.class)
.guiName("Imputation by mean")
.description("If imputation is performed by computing mean of the respective column")
.build();
private PluginParameter nearestNeighbors = new PluginParameter.Builder<>("nearestNeighbors", 5, Integer.class)
.guiName("Number of nearest neighbors to be evaluated")
.description("Choice of k in k-nearest neighbors algorithm. Default is 5.")
.build();
// Use enum to set the distance metric.
private PluginParameter distance = new PluginParameter.Builder<>("distance", distanceChoice.Euclidean, distanceChoice.class)
.guiName("Choose Distance type")
.description("Distance choice for computing nearest neighbors. Default choice is Euclidean distance.")
.build();
/**
* Creates a new instance of the ImputationPlugin
*/
public ImputationPlugin(Frame parentFrame, boolean isInteractive) {
super(parentFrame, isInteractive);
}
@Override
protected void preProcessParameters(DataSet input) {
if ((input == null) || (input.getSize() != 1)) {
throw new IllegalArgumentException("ImputationPlugin: preProcessParameters: Please select one Genotype Table or Phenotype.");
}
List data = input.getDataOfType(new Class[]{GenotypeTable.class, Phenotype.class});
if (data.size() != 1) {
throw new IllegalArgumentException("ImputationPlugin: preProcessParameters: Please select one Genotype Table or Phenotype.");
}
}
@Override
public DataSet processData(DataSet input) {
List datumList = input.getDataOfType(GenotypeTable.class);
//check size of datumList, throw error if not equal to one
if (datumList.size() != 1) { //not a GenotypeTable
datumList = input.getDataOfType(Phenotype.class);
if (datumList.size() != 1) {
throw new IllegalArgumentException("ImputationPlugin: Input must me a genotype table or phenotype.");
}
Phenotype myPhenotype = (Phenotype) datumList.get(0).getData();
// Only restrict to the numerical(data) attributes.
// Indices of the data attributes.
int[] dataAttrIndices = myPhenotype.attributeIndicesOfType(Phenotype.ATTRIBUTE_TYPE.data);
//dimensions of the data matrix.
int dataAttributes = dataAttrIndices.length;
int dataObservations = myPhenotype.numberOfObservations();
//Initialize the data matrix. This will be of size observations * data_attaributes.
double[][] data = new double[dataObservations][dataAttributes];
for (int s = 0; s < dataObservations; s++) {
for (int t = 0; t < dataAttributes; t++) {
if (!(myPhenotype.isMissing(s, dataAttrIndices[t]))) {
data[s][t] = (Float) myPhenotype.value(s, dataAttrIndices[t]);
} else {
data[s][t] = Double.NaN;
}
}
}
double[][] result;
// Convert the input file into a matrix, data.
Boolean imputationByMean;
imputationByMean = by_mean();
if (imputationByMean) {
result = ImputationByMean.impute(data);
} else {
boolean isManhattan = false;
boolean isCosine = false;
distanceChoice alpha = distance_choice();
if (alpha == distanceChoice.Manhattan) {
isManhattan = true;
}
if (alpha == distanceChoice.Cosine) {
isCosine = true;
}
Integer Nbrs = nearest_neighbors();
result = kNearestNeighbors.impute(data, Nbrs, isManhattan, isCosine);
}
List attributes = new ArrayList<>();
List types = new ArrayList<>();
attributes.add(myPhenotype.taxaAttribute());
types.add(Phenotype.ATTRIBUTE_TYPE.taxa);
attributes.addAll(myPhenotype.attributeListOfType(Phenotype.ATTRIBUTE_TYPE.factor));
for (int i = 0; i < myPhenotype.numberOfAttributesOfType(Phenotype.ATTRIBUTE_TYPE.factor); i++) {
types.add(Phenotype.ATTRIBUTE_TYPE.factor);
}
attributes.addAll(myPhenotype.attributeListOfType(Phenotype.ATTRIBUTE_TYPE.covariate));
for (int i = 0; i < myPhenotype.numberOfAttributesOfType(Phenotype.ATTRIBUTE_TYPE.covariate); i++) {
types.add(Phenotype.ATTRIBUTE_TYPE.covariate);
}
for (int i = 0; i < dataAttributes; i++) {
float[] attrData = new float[dataObservations];
for (int j = 0; j < dataObservations; j++) {
attrData[j] = (float) result[j][i];
}
PhenotypeAttribute oldAttribute = myPhenotype.attribute(dataAttrIndices[i]);
NumericAttribute myAttribute = new NumericAttribute(oldAttribute.name(), attrData, new OpenBitSet(dataObservations));
attributes.add(myAttribute);
types.add(Phenotype.ATTRIBUTE_TYPE.data);
}
Phenotype imputedPhenotype = new PhenotypeBuilder().fromAttributeList(attributes, types).build().get(0);
StringBuilder nameBuilder = new StringBuilder("Imputed_");
nameBuilder.append(datumList.get(0).getName());
StringBuilder commentBuilder = new StringBuilder(getMethodString());
commentBuilder.append("\nfrom ").append(datumList.get(0).getName());
Datum newDatum = new Datum(nameBuilder.toString(), imputedPhenotype, commentBuilder.toString());
return new DataSet(newDatum, this);
} else { //it is a GenotypeTable
GenotypeTable myGenotype = (GenotypeTable) datumList.get(0).getData();
int nsites = myGenotype.numberOfSites();
int ntaxa = myGenotype.numberOfTaxa();
//ReferenceProbability myProb;
double[][] data;
if (!myGenotype.hasReferenceProbablity()) {
data = GenotypeTableUtils.convertGenotypeToDoubleProbability(myGenotype, true);
} else {
//myProb = myGenotype.referenceProbability();
data = new double[nsites][ntaxa];
for (int s = 0; s < nsites; s++) {
for (int t = 0; t < ntaxa; t++) {
data[s][t] = myGenotype.referenceProbability(t, s);
}
}
}
double[][] result;
// Convert the input file into a matrix, data.
Boolean imputationByMean;
imputationByMean = by_mean();
if (imputationByMean) {
result = ImputationByMean.impute(data);
} else {
boolean isManhattan = false;
boolean isCosine = false;
distanceChoice alpha = distance_choice();
if (alpha == distanceChoice.Manhattan) {
isManhattan = true;
}
if (alpha == distanceChoice.Cosine) {
isCosine = true;
}
Integer Nbrs = nearest_neighbors();
result = kNearestNeighbors.impute(data, Nbrs, isManhattan, isCosine);
}
//build new ReferenceProbability
ReferenceProbabilityBuilder refBuilder = ReferenceProbabilityBuilder.getInstance(ntaxa, nsites, myGenotype.taxa());
for (int t = 0; t < ntaxa; t++) {
float[] values = new float[nsites];
for (int s = 0; s < nsites; s++) {
values[s] = (float) result[s][t];
}
refBuilder.addTaxon(t, values);
}
//build new GenotypeTable
GenotypeTable imputedGenotype = GenotypeTableBuilder.getInstance(myGenotype.genotypeMatrix(), myGenotype.positions(),
myGenotype.taxa(), myGenotype.depth(), myGenotype.alleleProbability(), refBuilder.build(), myGenotype.dosage(),
myGenotype.annotations());
StringBuilder nameBuilder = new StringBuilder("Imputed_");
nameBuilder.append(datumList.get(0).getName());
StringBuilder commentBuilder = new StringBuilder(getMethodString());
commentBuilder.append("\nfrom ").append(datumList.get(0).getName());
Datum newDatum = new Datum(nameBuilder.toString(), imputedGenotype, commentBuilder.toString());
return new DataSet(newDatum, this);
}
}
private String getMethodString() {
if (byMean.value()) return "Missing values imputed as mean of trait.";
return String.format("Missing values imputed using K-nearest neigbor with %s distance.", distance.value().name());
}
@Override
public String pluginDescription() {
return "This plugin takes an input file (genotype/phenotype) with missing values"
+ "and imputes the missing values using one the chosen methods."
+ "It returns the imputed file.";
}
@Override
public ImageIcon getIcon() {
return null;
}
@Override
public String getButtonName() {
return "Numerical Impute";
}
@Override
public String getToolTipText() {
return "Numerical Impute";
}
public distanceChoice distance_choice() {
return distance.value();
}
public ImputationPlugin distance_choice(distanceChoice value) {
distance = new PluginParameter<>(distance, value);
return this;
}
public Boolean by_mean() {
return byMean.value();
}
public ImputationPlugin by_mean(Boolean value) {
byMean = new PluginParameter<>(byMean, value);
return this;
}
public Integer nearest_neighbors() {
return nearestNeighbors.value();
}
public ImputationPlugin nearest_neighbors(Integer value) {
nearestNeighbors = new PluginParameter<>(nearestNeighbors, value);
return this;
}
}
© 2015 - 2025 Weber Informatics LLC | Privacy Policy