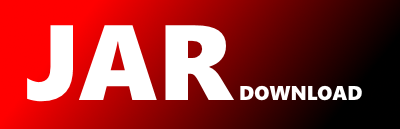
net.maizegenetics.stats.PCA.ClassicMds Maven / Gradle / Ivy
Go to download
Show more of this group Show more artifacts with this name
Show all versions of tassel Show documentation
Show all versions of tassel Show documentation
TASSEL is a software package to evaluate traits associations, evolutionary patterns, and linkage
disequilibrium.
package net.maizegenetics.stats.PCA;
import java.util.stream.IntStream;
import net.maizegenetics.matrixalgebra.Matrix.DoubleMatrix;
import net.maizegenetics.matrixalgebra.Matrix.DoubleMatrixFactory;
import net.maizegenetics.matrixalgebra.Matrix.DoubleMatrixFactory.FactoryType;
import net.maizegenetics.matrixalgebra.decomposition.EigenvalueDecomposition;
import net.maizegenetics.taxa.distance.DistanceMatrix;
public class ClassicMds {
//The method implemented in this class was adapted from R source code for the function cmdscale()
//expected input is a distance matrix
private DistanceMatrix myDistanceMatrix;
private EigenvalueDecomposition eigenDecomp;
private DoubleMatrix eigenVectors;
private int numberOfPositiveEigenvalues;
private double tol = 1e-8 ;
private int[] eigenSort;
public ClassicMds(DistanceMatrix dm) {
myDistanceMatrix = dm;
testDMforMissing();
calculatePCs();
}
public int maximumNumberOfPCs() {
return numberOfPositiveEigenvalues;
}
public double[] getPrincipalCoordinate(int index) {
if (index > numberOfPositiveEigenvalues - 1) return null;
double eval = Math.sqrt(eigenDecomp.getEigenvalue(eigenSort[index]));
int ntaxa = myDistanceMatrix.numberOfTaxa();
double[] pc = new double[ntaxa];
for (int i = 0; i < ntaxa; i++) pc[i] = eigenVectors.get(i, eigenSort[index]) * eval;
return pc;
}
public double getEigenvalue(int index) {
return eigenDecomp.getEigenvalue(eigenSort[index]);
}
private void calculatePCs() {
//square values in the distance matrix, then double center them
DoubleMatrix dm = SquaredDoubleMatrixFromDistanceMatrix();
int n = dm.numberOfRows();
//double center the matrix
for (int r = 0; r < n; r++) {
double mean = dm.rowSum(r) / n;
for (int c = 0; c < n; c++) {
dm.set(r,c, dm.get(r, c) - mean);
}
}
for (int c = 0; c < n; c++) {
double mean = dm.columnSum(c) / n;
for (int r = 0; r < n; r++) {
dm.set(r,c, dm.get(r, c) - mean);
}
}
//finally multiply by -1/2
dm.scalarMultEquals(-0.5);
//get an eigenvalue decomposition
eigenDecomp = dm.getEigenvalueDecomposition();
//calculate PC's for positive eigenvalues
numberOfPositiveEigenvalues = 0;
double[] eval = eigenDecomp.getEigenvalues();
for (int i = 0; i < n; i++) if (eval[i] > tol) numberOfPositiveEigenvalues++;
eigenVectors = eigenDecomp.getEigenvectors();
//determine the sort order
int nEigenvalues = eval.length;
eigenSort = IntStream.range(0, nEigenvalues).boxed().sorted((a,b) -> {
if (eval[a] > eval[b]) return -1;
if (eval[a] < eval[b]) return 1;
return 0;
}).mapToInt(I -> I.intValue()).toArray();
}
private DoubleMatrix SquaredDoubleMatrixFromDistanceMatrix() {
int n = myDistanceMatrix.getSize();
DoubleMatrix dm = DoubleMatrixFactory.DEFAULT.make(n, n);
for (int r = 0; r < n; r++) {
for (int c = 0; c < n; c++) {
double val = myDistanceMatrix.getDistance(r, c);
val *= val;
dm.set(r, c, val);
}
}
return dm;
}
private void testDMforMissing() {
int n = myDistanceMatrix.getSize();
for (int r = 0; r < n; r++) {
for (int c = 0; c < n; c++) {
if (!Double.isFinite(myDistanceMatrix.getDistance(r, c))) {
throw new RuntimeException("Distance matrix contains missing values in ClassicMds.");
}
}
}
}
}
© 2015 - 2025 Weber Informatics LLC | Privacy Policy