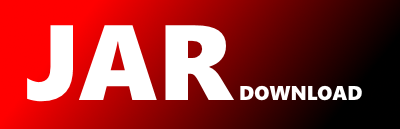
net.maizegenetics.stats.PCA.PrinComp Maven / Gradle / Ivy
Go to download
Show more of this group Show more artifacts with this name
Show all versions of tassel Show documentation
Show all versions of tassel Show documentation
TASSEL is a software package to evaluate traits associations, evolutionary patterns, and linkage
disequilibrium.
package net.maizegenetics.stats.PCA;
import net.maizegenetics.matrixalgebra.Matrix.DoubleMatrix;
import net.maizegenetics.matrixalgebra.Matrix.DoubleMatrixFactory;
import net.maizegenetics.matrixalgebra.decomposition.SingularValueDecomposition;
public class PrinComp {
public enum PC_TYPE {corr, cov};
SingularValueDecomposition svd;
DoubleMatrix datamatrix;
/**
* The class uses singular value decomposition to find the eigenvalues, eigenvectors and principal components of either the covariance or correlation matrix
* of the data. If covariance, then the result is the equivalent of finding the eighvalue decomposition of XX'/(n-1) where X is the data matrix with the
* column means subtracted from the columns. If correlation, the column values are also scaled.
* That is, after the mean is subtracted, the values are divided by the standard deviation.
* @param data a matrix of data
* @param type should the analysis use the covariance (cov) or the correlation (corr) matrix of the data
*/
public PrinComp(DoubleMatrix data, PC_TYPE type) {
datamatrix = data;
datamatrix = centerCols(data);
if (type == PC_TYPE.corr) scaleCenteredMatrix(datamatrix);
double multiplier = 1.0 / Math.sqrt(datamatrix.numberOfRows() - 1);
svd = datamatrix.scalarMult(multiplier).getSingularValueDecomposition();
}
/**
* @return a double[] of eigenvalues from the decomposition of either the covariance or correlation matrix of the data
*/
public double[] getEigenValues() {
double[] singularvals = svd.getSingularValues();
int n = singularvals.length;
double[] eigenvals = new double[n];
for (int i = 0; i < n; i++) eigenvals[i] = singularvals[i] * singularvals[i];
return eigenvals;
}
/**
* @return a column vector of eigenvalues from the decomposition of either the covariance or correlation matrix of the data
*/
public DoubleMatrix getEigenValuesAsColumnVector() {
double[] eigenvals = getEigenValues();
int n = eigenvals.length;
return DoubleMatrixFactory.DEFAULT.make(n, 1, eigenvals);
}
/**
* @return a square matrix with diagonal equal to the eigenvalues of the covariance or correlation matrix of the data
*/
public DoubleMatrix getEigenvalueMatrix() {
return DoubleMatrixFactory.DEFAULT.diagonal(getEigenValues());
}
/**
* @return the eigenvectors from the decomposition of either the covariance or correlation matrix of the data
*/
public DoubleMatrix getEigenVectors() {
return svd.getV(false);
}
/**
* calculated as data * eigenvectors
* @return all of the principal components
*/
public DoubleMatrix getPrincipalComponents() {
return datamatrix.mult(svd.getV(false));
}
private DoubleMatrix centerCols(DoubleMatrix data) {
int nrows = data.numberOfRows();
int ncols = data.numberOfColumns();
DoubleMatrix dm = data.copy();
for (int c = 0; c < ncols; c++) {
double colmean = dm.columnSum(c) / nrows;
for (int r = 0; r < nrows; r++) {
dm.set(r, c, dm.get(r, c) - colmean);
}
}
return dm;
}
private void scaleCenteredMatrix(DoubleMatrix data) {
int nrows = data.numberOfRows();
int ncols = data.numberOfColumns();
for (int c = 0; c < ncols; c++) {
double sumsq = 0;
for (int r = 0; r < nrows; r++) {
double val = data.get(r, c);
sumsq += val * val;
}
double stdDev = Math.sqrt(sumsq/(nrows - 1));
for (int r = 0; r < nrows; r++) {
double val = data.get(r, c);
data.set(r, c, val/stdDev);
}
}
}
}
© 2015 - 2025 Weber Informatics LLC | Privacy Policy