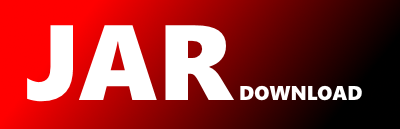
net.maizegenetics.analysis.association.RidgeRegressionEmmaPlugin Maven / Gradle / Ivy
Go to download
Show more of this group Show more artifacts with this name
Show all versions of tassel Show documentation
Show all versions of tassel Show documentation
TASSEL is a software package to evaluate traits associations, evolutionary patterns, and linkage
disequilibrium.
package net.maizegenetics.analysis.association;
import net.maizegenetics.stats.linearmodels.FactorModelEffect;
import net.maizegenetics.dna.snp.GenotypeTable;
import net.maizegenetics.matrixalgebra.Matrix.DoubleMatrix;
import net.maizegenetics.matrixalgebra.Matrix.DoubleMatrixFactory;
import net.maizegenetics.util.OpenBitSet;
import net.maizegenetics.util.SimpleTableReport;
import net.maizegenetics.phenotype.CategoricalAttribute;
import net.maizegenetics.phenotype.GenotypePhenotype;
import net.maizegenetics.phenotype.NumericAttribute;
import net.maizegenetics.phenotype.Phenotype;
import net.maizegenetics.phenotype.Phenotype.ATTRIBUTE_TYPE;
import net.maizegenetics.phenotype.PhenotypeAttribute;
import net.maizegenetics.phenotype.PhenotypeBuilder;
import net.maizegenetics.phenotype.TaxaAttribute;
import net.maizegenetics.plugindef.AbstractPlugin;
import net.maizegenetics.plugindef.DataSet;
import net.maizegenetics.plugindef.Datum;
import org.apache.log4j.Logger;
import javax.swing.*;
import java.awt.*;
import java.net.URL;
import java.util.ArrayList;
import java.util.LinkedList;
import java.util.List;
public class RidgeRegressionEmmaPlugin extends AbstractPlugin {
private static final Logger myLogger = Logger.getLogger(RidgeRegressionEmmaPlugin.class);
public RidgeRegressionEmmaPlugin(Frame parentFrame, boolean isInteractive) {
super(parentFrame, isInteractive);
}
@Override
public DataSet performFunction(DataSet input) {
try {
List datasets = input.getDataOfType(GenotypePhenotype.class);
if (datasets.size() < 1) {
String msg = "No datasets of an appropriate type were selected for the GS analysis.";
myLogger.error(msg);
if (isInteractive()) {
JOptionPane.showMessageDialog(getParentFrame(), msg, "GS Error", JOptionPane.ERROR_MESSAGE);
}
return null;
}
LinkedList results = new LinkedList();
for (Datum dataset : datasets) {
try {
LinkedList aResult = null;
aResult = processData(dataset);
if (aResult != null) {
results.addAll(aResult);
fireDataSetReturned(new DataSet(aResult, this));
}
} catch (Exception e) {
StringBuilder msg = new StringBuilder("Error in GS processing " + dataset.getName());
msg.append(". ").append(e.getMessage());
myLogger.error(msg.toString());
e.printStackTrace();
if (isInteractive()) {
JOptionPane.showMessageDialog(getParentFrame(), msg.toString(), "GS Error", JOptionPane.ERROR_MESSAGE);
}
}
}
return new DataSet(results, this);
} finally {
fireProgress(100);
}
}
public LinkedList processData(Datum dataset) {
DoubleMatrix phenotype;
DoubleMatrix genotype;
DoubleMatrix fixedEffects;
LinkedList theResults = new LinkedList();
GenotypePhenotype myGenoPheno = (GenotypePhenotype) dataset.getData();
GenotypeTable myGenotype = myGenoPheno.genotypeTable();
Phenotype myPhenotype = myGenoPheno.phenotype();
if (!myGenotype.hasReferenceProbablity()) {
throw new IllegalArgumentException("Incorrect data type. A numeric genotype was not found.");
}
//numbers of different things
int numberOfMarkers = myGenotype.numberOfSites();
List dataAttributeList = myPhenotype.attributeListOfType(ATTRIBUTE_TYPE.data);
List factorAttributeList = myPhenotype.attributeListOfType(ATTRIBUTE_TYPE.factor);
List covariateAttributeList = myPhenotype.attributeListOfType(ATTRIBUTE_TYPE.covariate);
TaxaAttribute myTaxaAttribute = myPhenotype.taxaAttribute();
//iterate through the phenotypes
for (PhenotypeAttribute attr : dataAttributeList) {
NumericAttribute dataAttribute = (NumericAttribute) attr;
//get phenotype data
double[] phenotypeData = AssociationUtils.convertFloatArrayToDouble(dataAttribute.floatValues());
int nObs = phenotypeData.length;
phenotype = DoubleMatrixFactory.DEFAULT.make(nObs, 1, phenotypeData);
//make the fixed effect matrix
int numberOfFactors = factorAttributeList.size();
int numberOfCovariates = covariateAttributeList.size();
int numberOfEffects = numberOfFactors + numberOfCovariates + 1;
if (numberOfEffects > 1) {
DoubleMatrix[][] effects = new DoubleMatrix[1][numberOfEffects];
effects[0][0] = DoubleMatrixFactory.DEFAULT.make(nObs, 1, 1);
for (int i = 0; i < numberOfFactors; i++) {
CategoricalAttribute fa = (CategoricalAttribute) factorAttributeList.get(i);
FactorModelEffect fme = new FactorModelEffect(fa.allIntValues(), true);
effects[0][i + 1] = fme.getX();
}
for (int i = 0; i < numberOfCovariates; i++) {
NumericAttribute na = (NumericAttribute) covariateAttributeList.get(i);
double[] values = AssociationUtils.convertFloatArrayToDouble(na.floatValues());
effects[0][i + numberOfFactors + 1] = DoubleMatrixFactory.DEFAULT.make(nObs, 1, values);
}
fixedEffects = DoubleMatrixFactory.DEFAULT.compose(effects);
} else {
fixedEffects = DoubleMatrixFactory.DEFAULT.make(nObs, 1, 1);
}
//get the genotypes
genotype = DoubleMatrixFactory.DEFAULT.make(nObs, numberOfMarkers);
String[] markerNames = new String[numberOfMarkers];
for (int m = 0; m < numberOfMarkers; m++) {
for (int i = 0; i < nObs; i++) {
genotype.set(i, m, myGenotype.referenceProbability(i, m));
}
markerNames[m] = myGenotype.siteName(m);
}
RegRidgeEmmaDoubleMatrix ridgeRegression = new RegRidgeEmmaDoubleMatrix(phenotype, fixedEffects, genotype);
ridgeRegression.solve();
//output the gebv's for the taxa
float[] gebv = AssociationUtils.convertDoubleArrayToFloat(ridgeRegression.getBlups());
String phenoName = attr.name();
ArrayList attributeList = new ArrayList<>();
ArrayList typeList = new ArrayList<>();
attributeList.add(myTaxaAttribute);
typeList.add(ATTRIBUTE_TYPE.taxa);
attributeList.add(new NumericAttribute(phenoName + "_GEBV", gebv, new OpenBitSet(nObs)));
typeList.add(ATTRIBUTE_TYPE.data);
Phenotype gebvPheno = new PhenotypeBuilder().assignName("GEBV_" + phenoName)
.fromAttributeList(attributeList, typeList)
.build().get(0);
String datumName = "GEBVs_" + phenoName + "_" + dataset.getName();
StringBuilder comment = new StringBuilder("Ridge Regression from ");
comment.append(dataset.getName()).append(":\n");
comment.append("Genomic Estimated Breeding Values (GEBVs)\n");
comment.append("trait = ").append(phenoName).append("\n");
comment.append(nObs).append(" lines");
theResults.add(new Datum(datumName, gebvPheno, comment.toString()));
//output the marker blups for the markers as a report
double[] markerBlups = ridgeRegression.getMrkBlups();
Object[][] blupTable = new Object[numberOfMarkers][2];
for (int i = 0; i < numberOfMarkers; i++) {
blupTable[i][0] = markerNames[i];
blupTable[i][1] = new Double(markerBlups[i]);
}
SimpleTableReport str = new SimpleTableReport("Marker BLUPs for " + dataset.getName(), new String[]{"Marker", phenoName + "_BLUP"}, blupTable);
datumName = dataset.getName() + "_marker BLUPs_" + phenoName;
comment = new StringBuilder("Ridge Regression from ");
comment.append(dataset.getName()).append(":\n");
comment.append("Marker BLUPs\n");
comment.append("trait = ").append(phenoName).append("\n");
comment.append(numberOfMarkers).append(" markers");
theResults.add(new Datum(datumName, str, comment.toString()));
}
return theResults;
}
@Override
public ImageIcon getIcon() {
URL imageURL = RidgeRegressionEmmaPlugin.class.getResource("/net/maizegenetics/analysis/images/LinearAssociation.gif");
if (imageURL == null) {
return null;
} else {
return new ImageIcon(imageURL);
}
}
@Override
public String getButtonName() {
return "Genomic Selection";
}
@Override
public String getToolTipText() {
return "Predict Phenotypes using Ridge Regression for Genomic Selection";
}
}
© 2015 - 2025 Weber Informatics LLC | Privacy Policy