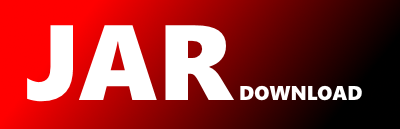
net.maizegenetics.analysis.imputation.LDKNNiImputationPlugin Maven / Gradle / Ivy
Go to download
Show more of this group Show more artifacts with this name
Show all versions of tassel Show documentation
Show all versions of tassel Show documentation
TASSEL is a software package to evaluate traits associations, evolutionary patterns, and linkage
disequilibrium.
package net.maizegenetics.analysis.imputation;
import com.google.common.collect.ArrayListMultimap;
import com.google.common.collect.MinMaxPriorityQueue;
import com.google.common.collect.Multimap;
import com.google.common.collect.Range;
import net.maizegenetics.analysis.popgen.LDResult;
import net.maizegenetics.analysis.popgen.LinkageDisequilibrium;
import net.maizegenetics.dna.map.Position;
import net.maizegenetics.dna.map.PositionList;
import net.maizegenetics.dna.map.PositionListBuilder;
import net.maizegenetics.dna.snp.*;
import net.maizegenetics.plugindef.*;
import net.maizegenetics.taxa.Taxon;
import net.maizegenetics.util.Tuple;
import org.apache.log4j.Logger;
import javax.swing.*;
import java.awt.*;
import java.util.ArrayList;
import java.util.List;
import java.util.concurrent.atomic.LongAdder;
import java.util.stream.Collectors;
import java.util.stream.IntStream;
import static net.maizegenetics.dna.snp.GenotypeTable.UNKNOWN_DIPLOID_ALLELE;
import static net.maizegenetics.dna.snp.GenotypeTableUtils.getDiploidValue;
import static net.maizegenetics.dna.snp.GenotypeTableUtils.getUnphasedSortedDiploidValue;
/**
* This imputation algorithm uses LD to identify good predictors for each SNP,
* and then uses the high LD SNPs to identify K- Nearest Neighbors.
* The genotype is called with a weighted mode of the KNNs.
*
* @author Daniel Money (Developer and developed the algorithm)
* @author Ed Buckler (assisted in conversion to TASSEL)
*/
public class LDKNNiImputationPlugin extends AbstractPlugin {
private PluginParameter highLDSSites = new PluginParameter.Builder<>("highLDSSites", 30, Integer.class)
.range(Range.closed(2, 2000))
.guiName("High LD Sites")
.description("Number of sites in high LD to use in imputation")
.build();
private PluginParameter knnTaxa = new PluginParameter.Builder<>("knnTaxa", 10, Integer.class)
.range(Range.closed(2, 200))
.guiName("Number of nearest neighbors")
.description("Number of neighbors to use in imputation")
.build();
private PluginParameter maxDistance = new PluginParameter.Builder<>("maxLDDistance", 10_000_000, Integer.class)
.guiName("Max distance between site to find LD")
.description("Maximum physical distance between sites to search for LD (-1 for no distance cutoff - unlinked chromosomes will be tested)")
.build();
private static final Logger myLogger = Logger.getLogger(LDKNNiImputationPlugin.class);
public LDKNNiImputationPlugin() {
super(null, false);
}
public LDKNNiImputationPlugin(Frame parentFrame, boolean isInteractive) {
super(parentFrame, isInteractive);
}
@Override
protected void preProcessParameters(DataSet input) {
List alignInList = input.getDataOfType(GenotypeTable.class);
if (alignInList.size() != 1) {
throw new IllegalArgumentException("LDKNNiImputationPlugin: preProcessParameters: Please select one Genotype Table.");
}
}
@Override
public DataSet processData(DataSet input) {
// Load in the genotype table
Datum genoDatum=input.getDataOfType(GenotypeTable.class).get(0);
GenotypeTable genotypeTable = (GenotypeTable)genoDatum.getData();
// Create a multimap of the SNPs in highest LD with each SNP
/*Debatable on what to calc*/ // Multimap highLDMap = getHighLDMap(GenotypeTableBuilder.getHomozygousInstance(genotypeTable), highLDSSites());
Multimap highLDMap = getHighLDMap(genotypeTable, highLDSSites());
System.out.println("LD calculated");
GenotypeTableBuilder incSiteBuilder = GenotypeTableBuilder.getSiteIncremental(genotypeTable.taxa());
//Start imputing site by site
long time=System.nanoTime();
LongAdder sites1Kdone=new LongAdder();
IntStream.range(0, genotypeTable.numberOfSites()).parallel().forEach(posIndex ->
{
Position position = genotypeTable.positions().get(posIndex);
PositionList positionList = PositionListBuilder.getInstance(new ArrayList<>(highLDMap.get(position)));
byte[] currGenos = genotypeTable.genotypeAllTaxa(posIndex);
byte[] newGenos = new byte[currGenos.length];
//set monomorphic sites to the major allele
if (!genotypeTable.isPolymorphic(posIndex)) {
byte monomorphicGenotype = getDiploidValue(genotypeTable.majorAllele(posIndex), genotypeTable.majorAllele(posIndex));
for (int i = 0; i < newGenos.length; i++) {
newGenos[i] = (currGenos[i] == UNKNOWN_DIPLOID_ALLELE) ? monomorphicGenotype : currGenos[i];
}
} else {
//create a site specific in memory filtered alignment to use
final GenotypeTable ldGenoTable = GenotypeTableBuilder.getGenotypeCopyInstance(FilterGenotypeTable.getInstance(genotypeTable, positionList));
final int numberSites = ldGenoTable.numberOfSites();
double[] taxaCoverage = IntStream.range(0, ldGenoTable.numberOfTaxa()).sequential() //used determine when insufficient overlap is likely
.mapToDouble(t -> (double) ldGenoTable.totalNonMissingForTaxon(t) / (double) numberSites)
.toArray();
for (int taxon = 0; taxon < currGenos.length; taxon++) {
newGenos[taxon] = currGenos[taxon];
if (currGenos[taxon] == UNKNOWN_DIPLOID_ALLELE) { //starting imputing
Multimap closeGenotypes = getClosestNonMissingTaxa(genotypeTable.taxa().get(taxon), genotypeTable,
ldGenoTable, position, taxaCoverage, knnTaxa());
if (closeGenotypes.isEmpty()) { //this is empty when two few high LD sites are shared between the target taxon and all others
newGenos[taxon] = UNKNOWN_DIPLOID_ALLELE;
} else {
newGenos[taxon] = impute(closeGenotypes, highLDSSites()); //set to weight mode genotype
}
}
}//);
}
incSiteBuilder.addSite(position, newGenos);
if ((posIndex + 1) % 100 == 0) {
sites1Kdone.add(100);
fireProgress(33 + ((int) (66 * sites1Kdone.longValue()) / genotypeTable.numberOfSites()));
System.out.println(sites1Kdone.longValue() + ":" + ((System.nanoTime() - time) / 1_000_000) / sites1Kdone.longValue());
}
});
GenotypeTable impGenotypeTable = incSiteBuilder.build();
return new DataSet(new Datum(genoDatum.getName()+"_KNNimp",impGenotypeTable,"Imputed genotypes by KNN imputation"),this);
}
/**
* Create a multimap of distances between the target taxon with the closest observed genotypes. Distance is calculated
* between the target taxon and all other taxa for the ldGenoTable (subset of high LD sites).
* @param inputTaxon Taxon being imputed
* @param genotypeTable Master genotype table
* @param ldGenoTable Subset genotype table with only the high LD sites
* @param targetPosition The position in the master genotype table being imputed.
* @param numberOfTaxa number of genotypes to retain
* @return Map of distance to genotype call for the target position
*/
private Multimap getClosestNonMissingTaxa(Taxon inputTaxon, GenotypeTable genotypeTable, GenotypeTable ldGenoTable,
Position targetPosition, double[] inputCoverage, int numberOfTaxa) {
final int targetPosIdx = genotypeTable.positions().indexOf(targetPosition);
final int inputTaxonIdx = genotypeTable.taxa().indexOf(inputTaxon);
byte[] inputTaxonGenotypes = ldGenoTable.genotypeAllSites(inputTaxonIdx);
// double inputTaxonCoverage=(double)ldGenoTable.totalNonMissingForTaxon(inputTaxonIdx)/(double)ldGenoTable.numberOfSites();
MinMaxPriorityQueue> topTaxa = IntStream.range(0, genotypeTable.numberOfTaxa())
.filter(closeTaxonIdx -> closeTaxonIdx != inputTaxonIdx) //do not test itself
.filter(closeTaxonIdx -> inputCoverage[closeTaxonIdx] * inputCoverage[inputTaxonIdx]*(double)ldGenoTable.numberOfSites()>10) //skip tests with
.filter(closeTaxonIdx -> genotypeTable.genotype(closeTaxonIdx, targetPosIdx) != GenotypeTable.UNKNOWN_DIPLOID_ALLELE) //ignore taxa with the genotype not scored
/*Bad*/ // .mapToObj(closeTaxonIdx -> new Tuple<>(IBSDistanceMatrix.computeHetDistances(inputTaxonGenotypes, ldGenoTable.genotypeAllSites(closeTaxonIdx), 10)[0], genotypeTable.genotype(closeTaxonIdx, targetPosIdx)))
/*Best*/.mapToObj(closeTaxonIdx -> new Tuple<>(dist(inputTaxonGenotypes, ldGenoTable.genotypeAllSites(closeTaxonIdx), 10)[0], genotypeTable.genotype(closeTaxonIdx, targetPosIdx))) //calculate the distance
/*Best*/ // .mapToObj(closeTaxonIdx -> new Tuple<>(LDKNNiImputationPluginEd.distance(inputTaxonGenotypes, ldGenoTable.genotypeAllSites(closeTaxonIdx), 10)[0], genotypeTable.genotype(closeTaxonIdx, targetPosIdx)))
.filter(distanceTaxon -> !Double.isNaN(distanceTaxon.x)) //skip is too few sites (<10 results in NaN)
.collect(Collectors.toCollection(() -> MinMaxPriorityQueue.maximumSize(numberOfTaxa).create()));
final Multimap distGenoMap = ArrayListMultimap.create();
topTaxa.stream().forEach(distGeno -> distGenoMap.put(distGeno.x, distGeno.y));
return distGenoMap;
}
private Multimap getHighLDMap(GenotypeTable genotypeTable, int numberOfSNPs) {
Multimap highLDMap = ArrayListMultimap.create();
final int numberOfSites = genotypeTable.numberOfSites();
LongAdder sites1Kdone=new LongAdder();
IntStream.range(0, genotypeTable.numberOfSites()).parallel()
.forEach(posIndex ->
{
MinMaxPriorityQueue highestLD = MinMaxPriorityQueue.orderedBy(LDResult.byR2Ordering.reverse())
.maximumSize(numberOfSNPs).create();
for (int site2 = 0; site2 < numberOfSites; site2++) {
if (posIndex == site2) {
continue;
}
if(maxDistance()>-1 && Math.abs(genotypeTable.chromosomalPosition(posIndex)-genotypeTable.chromosomalPosition(site2))>maxDistance()) {
continue;
}
LDResult ld = LinkageDisequilibrium.calculateBitLDForHaplotype(false, 20, genotypeTable, posIndex, site2);
if (Double.isNaN(ld.r2())) {
continue;
}
highestLD.add(ld);
}
List positionList = new ArrayList<>();
for (LDResult result : highestLD) {
positionList.add(genotypeTable.positions().get(result.site2()));
}
highLDMap.putAll(genotypeTable.positions().get(posIndex), positionList);
if((posIndex+1)%1000==0) {
sites1Kdone.add(1000);
fireProgress((int)(33*sites1Kdone.longValue())/numberOfSites);
System.out.println(sites1Kdone.longValue());
}
});
return highLDMap;
}
/**
* Imputes to the most common genotype weighted by distance
* @param distGeno Multimap of the closest genotypes and their distance
* @param useLDSites Number of high LD sites used.
* @return The imputed genotype
*/
static byte impute(Multimap distGeno, int useLDSites) {
// useLDSites is used to scale distance so is similar to DMs original implementation.
// Seems to have at most a small effect on accuracy. Could be removed?
// Create an array to store the weighted counts of each genotype
double[] weightedCount = new double[256];
// For each distance to genotype / genotype pair update the weighted counts
distGeno.entries().forEach(entry -> {
// +128 is because bytes have values from -128..127 but we want 0..255 for array indexes
weightedCount[entry.getValue() + 128] += 1.0 / (1.0 + useLDSites * entry.getKey());
});
// Find the best genotype - the one with the maximum rate
int bestGeno = 0;
double bestWeightedCount = weightedCount[0];
for (int i = 1; i < 256; i++) {
if (weightedCount[i] > bestWeightedCount) {
bestWeightedCount = weightedCount[i];
bestGeno = i;
}
}
//Return the best genotype. -128 is for the same reason we added it above.
return (byte) (bestGeno - 128);
}
/*
Alternative approach to determine the best genotype
This is an allele based approaches. Provides a weighting of each allele, and Heterozygous is called currently
if the second allele has the count more than 1/2 the most common.
Provides roughly the same results as the genotype approach. An ensemble is best but lower call rate.
Both approaches have value.
*/
// public static byte impute(Multimap distGeno, int useLDSites) {
// // Create an array to store the weighted counts
// TByteDoubleMap alleleWeight=new TByteDoubleHashMap(16);
//
// // For each distance to genotype / genotype pair update the weighted counts
// distGeno.entries().forEach(entry -> {
// byte[] alleles= GenotypeTableUtils.getDiploidValues(entry.getValue());
// double weight= 1.0 / (1.0 + useLDSites * entry.getKey());
// alleleWeight.adjustOrPutValue(alleles[0],weight,weight);
// alleleWeight.adjustOrPutValue(alleles[1],weight,weight);
// });
//
// double[] weights= alleleWeight.values();
// Arrays.sort(weights);
//
// if(weights.length==1) {
// return GenotypeTableUtils.getUnphasedDiploidValue(alleleWeight.keys()[0], alleleWeight.keys()[0]);
// } else if(weights[weights.length-1]>(2*weights[weights.length-2])) {
// alleleWeight.retainEntries((a,w) -> w>=weights[weights.length-1]);
// byte[] alleles=alleleWeight.keys();
// return GenotypeTableUtils.getUnphasedDiploidValue(alleles[0], alleles[0]);
// } else {
// alleleWeight.retainEntries((a,w) -> w>=weights[weights.length-2]);
// byte[] alleles=alleleWeight.keys();
// return GenotypeTableUtils.getUnphasedDiploidValue(alleles[0], alleles[1]);
// }
// }
@Override
public String getCitation() {
return "Daniel Money, Kyle Gardner, Heidi Schwaninger, Gan-Yuan Zhong, Sean Myles. (In Review) " +
" LinkImpute: fast and accurate genotype imputation for non-model organisms";
}
@Override
public ImageIcon getIcon() {
return null;
}
@Override
public String getButtonName() {
return "LD KNNi Imputation";
}
@Override
public String getToolTipText() {
return "LD KNNi Imputation";
}
// The following getters and setters were auto-generated.
// Please use this method to re-generate.
//
public static void main(String[] args) {
GeneratePluginCode.generate(LDKNNiImputationPlugin.class);
}
/**
* Convenience method to run plugin with one return object.
*/
public GenotypeTable runPlugin(DataSet input) {
return (GenotypeTable) performFunction(input).getData(0).getData();
}
/**
* Maximum number of sites in high LD to use in imputation
*
* @return High LD Sites
*/
public Integer highLDSSites() {
return highLDSSites.value();
}
/**
* Set High LD Sites. Maximum number of sites in high
* LD to use in imputation
*
* @param value High LD Sites
*
* @return this plugin
*/
public LDKNNiImputationPlugin highLDSSites(Integer value) {
highLDSSites = new PluginParameter<>(highLDSSites, value);
return this;
}
/**
* Maximum number of neighbours to use in imputation
*
* @return Number of nearest neighbors
*/
public Integer knnTaxa() {
return knnTaxa.value();
}
/**
* Set Number of nearest neighbors. Maximum number of
* neighbours to use in imputation
*
* @param value Number of nearest neighbors
*
* @return this plugin
*/
public LDKNNiImputationPlugin knnTaxa(Integer value) {
knnTaxa = new PluginParameter<>(knnTaxa, value);
return this;
}
/**
* Maximum physical distance between sites to look for
* LD (-1 for no distance cutoff - unlinked chromosomes
* will be tested)
*
* @return Max distance between site to find LD
*/
public Integer maxDistance() {
return maxDistance.value();
}
/**
* Set Max distance between site to find LD. Maximum physical
* distance between sites to look for LD (-1 for no distance
* cutoff - unlinked chromosomes will be tested)
*
* @param value Max distance between site to find LD
*
* @return this plugin
*/
public LDKNNiImputationPlugin maxDistance(Integer value) {
maxDistance = new PluginParameter<>(maxDistance, value);
return this;
}
/**
Alternative to current IBS distance measure
AA <> AA = 0
Aa <> Aa = 0 distance (normal IBS distance this is 0.5)
AA <> aa = 1 distance
*/
//TODO Terry revisit where this should go
//TAS-787
public static double[] dist(byte[] b1, byte[] b2, int min) {
int distance = 0;
int count = 0;
for (int i = 0; i < b1.length; i++) {
//Make sure hets are represented the same way
byte p1 = getUnphasedSortedDiploidValue(b1[i]);
byte p2 = getUnphasedSortedDiploidValue(b2[i]);
//Ignore the case where either genotype is unknown
if ((p1 != GenotypeTable.UNKNOWN_DIPLOID_ALLELE) && (p2 != GenotypeTable.UNKNOWN_DIPLOID_ALLELE)) {
// count counts how many snps we've actually used to scale the
// distance with since some snps will be unknown
count++;
//If the genotypes are unknown then we need to increase the distance
if (p1 != p2) {
//If either genotype is a het we must be either AA <> Aa or aa <> Aa so add one
if (GenotypeTableUtils.isHeterozygous(p1) ||
GenotypeTableUtils.isHeterozygous(p2)) {
distance += 1;
//else we must be aa <> AA so add two
} else {
distance += 2;
}
}
}
}
// If we haven't found enough snps to include in the calculation return NaN
if (count < min) {
return new double[]{Double.NaN,count};
}
//Else return the scaled distance
else {
return new double[]{((double) distance / (double) (2 * count)),count};
}
}
}
© 2015 - 2025 Weber Informatics LLC | Privacy Policy