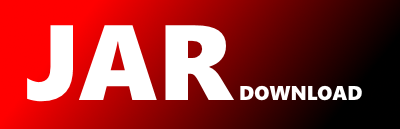
net.maizegenetics.analysis.numericaltransform.kNearestNeighbors Maven / Gradle / Ivy
Go to download
Show more of this group Show more artifacts with this name
Show all versions of tassel Show documentation
Show all versions of tassel Show documentation
TASSEL is a software package to evaluate traits associations, evolutionary patterns, and linkage
disequilibrium.
package net.maizegenetics.analysis.numericaltransform;
import com.google.common.collect.MinMaxPriorityQueue;
import java.util.AbstractMap;
import java.util.HashMap;
import java.util.Iterator;
import java.util.Map;
/**
* Imputation of the missing data by k-nearest neighbors.
*
* @author Janu Verma
* @author Terry Casstevens
*
*/
public class kNearestNeighbors {
private kNearestNeighbors() {
// utility class
}
/**
* Impute all the missing values.
*
* @param data matrix
* @param k - number of nearest neighbors to be computed.
* @param isManhattan - if true Manhattan distance will be used.
* @param isCosine - if true Cosine similarity will be used.
* @return imputed data matrix
*/
public static double[][] impute(double[][] data, int k, boolean isManhattan, boolean isCosine) {
int rows = data.length;
int cols = data[0].length;
double[][] result = new double[rows][cols];
for (int i = 0; i < rows; i++) {
double[][] neighbors = null;
boolean neighborsCalculated = false;
for (int j = 0; j < cols; j++) {
if (!Double.isNaN(data[i][j])) {
result[i][j] = data[i][j];
} else {
if (isCosine) {
neighbors = cosineSimRank(data, i, j, k);
result[i][j] = calcKNN(data, neighbors, j);
} else {
if (!neighborsCalculated) {
neighbors = KNearestNeighbor(data, i, k, isManhattan);
neighborsCalculated = true;
}
result[i][j] = calcKNN(data, neighbors, j);
}
}
}
}
return result;
}
/**
* Compute the fill-in value.
*
* @param data matrix
* @param neighbors neighbors
* @param col - col containing the missing value
* @return value to be filled in for the missing data point.
*/
private static double calcKNN(double[][] data, double[][] neighbors, int col) {
double num = 0.0;
int numberOfNonMissingValues = 0;
for (double[] neighbor : neighbors) {
if (!Double.isNaN(neighbor[col])) {
num += neighbor[col];
numberOfNonMissingValues++;
}
}
if (numberOfNonMissingValues == 0) {
return columnMean(data, col);
}
return (num / numberOfNonMissingValues);
}
/**
* @param data a matrix
* @param col the column of the matrix for which the mean should be computed
* @return the mean of the column, ignoring missing values
*/
public static double columnMean(double[][] data, int col) {
double sum = 0;
double count = 0;
for (double[] row : data) {
if (!Double.isNaN(row[col])) {
sum += row[col];
count++;
}
}
if (count == 0) {
return Double.NaN;
}
return sum / count;
}
/**
* Computes the cosine similarity of two vectors.
*
* @param data1 array
* @param data2 array
* @return angle between the two vectors.
*/
public static double cosine(double data1[], double data2[]) {
int l1 = data1.length;
int l2 = data2.length;
double result = 0.0;
for (int i = 0; i < l1; i++) {
if ((!Double.isNaN(data1[i])) && (!Double.isNaN(data2[i]))) {
result += (data1[i] * data2[i]);
}
}
double norm1 = 0.0;
for (int i = 0; i < l1; i++) {
if (!Double.isNaN(data1[i])) {
norm1 += Math.pow(data1[i], 2);
}
}
double norm2 = 0.0;
for (int j = 0; j < l2; j++) {
if (!Double.isNaN(data2[j])) {
norm2 += Math.pow(data2[j], 2);
}
}
double normProduct = norm1 * norm2;
return result / normProduct;
}
/**
* Rank all the rows according to their cosine similarity with the given
* row.
*
* @param data matrix
* @param row
* @param col
* @param k
* @return matrix whose rows are ordered according to their similarity with
* given row.
*/
public static double[][] cosineSimRank(double data[][], int row, int col, int k) {
int nRows = data.length;
int nCols = data[0].length;
double[] query = data[row];
double[][] neighbors = new double[k][];
Map distances = new HashMap<>();
for (int i = 0; i < nRows; i++) {
if (i != col) {
double[] alpha = data[i];
distances.put(i, cosine(query, alpha));
}
}
for (int n = 0; n < k; n++) {
double longestDistance = -10000.0;
int IndexS = 0;
Iterator it = distances.entrySet().iterator();
while (it.hasNext()) {
Map.Entry distance = (Map.Entry) it.next();
if (distance.getValue() > longestDistance) {
longestDistance = distance.getValue();
IndexS = distance.getKey();
}
}
neighbors[n] = data[IndexS];
distances.remove(IndexS);
}
return neighbors;
}
/**
* Compute Manhattan or Eulcidean distance between two vectors.
*
* @param data1 array
* @param data2 array
* @param isManhattan - true for Manhattan distance choice.
* @return value of the distance between two vectors.
*/
public static double distance(double data1[], double data2[], boolean isManhattan) {
int l1 = data1.length;
double result = 0.0;
double count = l1;
if (isManhattan) {
for (int i = 0; i < l1; i++) {
double diff = data1[i] - data2[i];
if (Double.isNaN(diff)) {
count -= 1.0;
} else {
result += Math.abs(diff);
}
}
} else {
for (int i = 0; i < l1; i++) {
double diff = data1[i] - data2[i];
if (Double.isNaN(diff)) {
count -= 1.0;
} else {
result += diff * diff;
}
}
}
return result / count;
}
/**
* Rank the rows based on their distance from the given row.
*
* @param data matrix
* @param row
* @param k
* @param isManhattan
* @return matrix
*/
public static double[][] KNearestNeighbor(double data[][], int row, int k, boolean isManhattan) {
int nRows = data.length;
MinMaxPriorityQueue> distances
= MinMaxPriorityQueue.orderedBy((Map.Entry o1, Map.Entry o2) -> {
return Double.compare(o1.getKey(), o2.getKey());
}).maximumSize(k).create();
double highestLowest = -1.0;
for (int i = 0; i < nRows; i++) {
double current = distance(data[row], data[i], isManhattan);
if ((distances.size() < k) || (current < highestLowest)) {
distances.add(new AbstractMap.SimpleEntry<>(current, data[i]));
highestLowest = distances.peekLast().getKey();
}
}
double[][] neighbors = new double[k][];
for (int n = 0; n < k; n++) {
neighbors[n] = distances.poll().getValue();
}
return neighbors;
}
}
© 2015 - 2025 Weber Informatics LLC | Privacy Policy