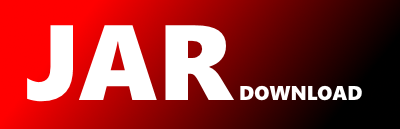
net.maizegenetics.stats.linearmodels.LinearModelForStepwiseRegression Maven / Gradle / Ivy
Go to download
Show more of this group Show more artifacts with this name
Show all versions of tassel Show documentation
Show all versions of tassel Show documentation
TASSEL is a software package to evaluate traits associations, evolutionary patterns, and linkage
disequilibrium.
package net.maizegenetics.stats.linearmodels;
import java.util.ArrayList;
import java.util.Arrays;
import net.maizegenetics.matrixalgebra.Matrix.DoubleMatrix;
import net.maizegenetics.matrixalgebra.Matrix.DoubleMatrixFactory;
import net.maizegenetics.util.SimpleTableReport;
public class LinearModelForStepwiseRegression {
ArrayList modelEffects;
int numberOfRequiredEffects = 1;
double[] data;
double enterLimit = 1e-3;
double exitLimit = 1e-3;
DoubleMatrix[][] xtxmatrices;
DoubleMatrix[] xtymatrices;
SweepFastLinearModel lm;
PartitionedLinearModel plm;
public LinearModelForStepwiseRegression(ArrayList requiredEffects, double[] data) {
modelEffects = requiredEffects;
numberOfRequiredEffects = modelEffects.size();
this.data = data;
xtxmatrices = new DoubleMatrix[numberOfRequiredEffects][numberOfRequiredEffects];
xtymatrices = new DoubleMatrix[numberOfRequiredEffects];
for (int i = 0; i < numberOfRequiredEffects; i++) {
xtymatrices[i] = requiredEffects.get(i).getXty(data);
xtxmatrices[i][i] = requiredEffects.get(i).getXtX();
for (int j = i + 1; j < numberOfRequiredEffects; j++) {
xtxmatrices[i][j] = ModelEffectUtils.getXtY(requiredEffects.get(i), requiredEffects.get(j));
}
}
lm = new SweepFastLinearModel(requiredEffects, data);
double ss = lm.getResiduals().crossproduct().get(0, 0);
double[] ssdf = lm.getResidualSSdf();
plm = new PartitionedLinearModel(modelEffects, lm);
}
public void addEffect(ModelEffect me) {
modelEffects.add(me);
int newdim = xtxmatrices.length + 1;
DoubleMatrix[][] oldxtx = xtxmatrices;
DoubleMatrix[] oldxty = xtymatrices;
xtxmatrices = new DoubleMatrix[newdim][newdim];
xtymatrices = new DoubleMatrix[newdim];
for (int i = 0; i < newdim - 1; i++) {
xtymatrices[i] = oldxty[i];
for (int j = i; j < newdim - 1; j++) {
xtxmatrices[i][j] = oldxtx[i][j];
}
}
xtxmatrices[newdim - 1][newdim - 1] = me.getXtX();
xtymatrices[newdim - 1] = me.getXty(data);
for (int i = 0; i < newdim - 1; i++) {
xtxmatrices[i][newdim - 1] = ModelEffectUtils.getXtY(modelEffects.get(i), me);
}
lm = new SweepFastLinearModel(modelEffects, xtxmatrices, xtymatrices, data);
double ss = lm.getResiduals().crossproduct().get(0, 0);
double[] ssdf = lm.getResidualSSdf();
plm = new PartitionedLinearModel(modelEffects, lm);
}
public double[] testNewEffect(ModelEffect me) {
//returns F, p-value for test
//see if there is a term that can be added using a partitioned model
plm.testNewModelEffect(me);
return plm.getFp();
}
public double testNewEffect(double[] covariate) {
return plm.testNewModelEffect(covariate);
}
public double[] getFpFromModelSS(double modelss) {
plm.setModelSS(modelss);
return plm.getFp();
}
public ModelEffect backwardStep() {
int numberOfModelEffects = modelEffects.size();
if (numberOfModelEffects - numberOfRequiredEffects > 1) {
double maxp = -1;
double[] errorSSdf = lm.getResidualSSdf();
double errorms = errorSSdf[0] / errorSSdf[1];
int maxEffectnumber = -1;
for (int i = numberOfRequiredEffects; i < numberOfModelEffects; i++) {
double[] ssdf = lm.getMarginalSSdf(i);
double F = ssdf[0] / ssdf[1] / errorms;
double p = -1;
try { p = LinearModelUtils.Ftest(F, ssdf[1], errorSSdf[1]); }
catch(Exception e) {
System.err.println("Error calculating p value at effect = " + i);
}
if (p > maxp) {
maxp = p;
maxEffectnumber = i;
}
}
if (maxp > exitLimit) {
return removeTerm(maxEffectnumber);
}
}
return null;
}
public ModelEffect removeTerm(int termNumber) {
//remove the effect from the matrices
int olddim = xtxmatrices.length;
int newdim = olddim -1;
DoubleMatrix[][] oldxtx = xtxmatrices;
DoubleMatrix[] oldxty = xtymatrices;
xtxmatrices = new DoubleMatrix[newdim][newdim];
xtymatrices = new DoubleMatrix[newdim];
for (int i = 0; i < newdim; i++) {
int ii = i;
if (i >= termNumber) ii++;
xtymatrices[i] = oldxty[ii];
for (int j = i; j < newdim; j++) {
int jj = j;
if (j >= termNumber) jj++;
xtxmatrices[i][j] = oldxtx[ii][jj];
}
}
//recalculate the model
ModelEffect removedEffect = modelEffects.remove(termNumber);
lm = new SweepFastLinearModel(modelEffects, xtxmatrices, xtymatrices, data);
plm = new PartitionedLinearModel(modelEffects, lm);
return removedEffect;
}
public DoubleMatrix getyhat() {
double[] beta = lm.getBeta();
int numberOfEffects = modelEffects.size();
int start = 0;
DoubleMatrix yhat = DoubleMatrixFactory.DEFAULT.make(data.length, 1, 0);
for (int i = 0; i < numberOfEffects; i++) {
ModelEffect me = modelEffects.get(i);
int nLevels = me.getNumberOfLevels();
yhat.plusEquals(me.getyhat(Arrays.copyOfRange(beta, start, start + nLevels)));
start += nLevels;
}
return yhat;
}
public void changeData(double[] newdata) {
data = newdata;
int numberOfEffects = modelEffects.size();
for (int i = 0; i < numberOfEffects; i++) {
ModelEffect me = modelEffects.get(i);
xtymatrices[i] = me.getXty(newdata);
}
lm = new SweepFastLinearModel(modelEffects, xtxmatrices, xtymatrices, data);
plm = new PartitionedLinearModel(modelEffects, lm);
}
public SimpleTableReport outputResults(String title, String traitname) {
String[] heads = new String[]{"Trait","Term","SS","df", "MS", "F", "p", "Rsq"};
int numberOfEffects = modelEffects.size();
Object[][] results = new Object[numberOfEffects + 1][];
double errordf = lm.getResidualSSdf()[1];
double errorss = lm.getResidualSSdf()[0];
double modelss = lm.getModelcfmSSdf()[0];
double modeldf = lm.getModelcfmSSdf()[1];
double totalss = lm.getFullModelSSdf()[0] + errorss;
Object[] result;
for (int i = 1; i < numberOfEffects; i++) {
int col = 0;
result = new Object[heads.length];
double[] ssdf = lm.getMarginalSSdf(i);
result[col++] = traitname;
result[col++] = modelEffects.get(i).getID();
result[col++] = ssdf[0];
result[col++] = ssdf[1];
result[col++] = ssdf[0]/ssdf[1];
double F = ssdf[0] / ssdf[1] / errorss * errordf;
result[col++] = F;
try {result[col++] = LinearModelUtils.Ftest(F, ssdf[1], errordf);}
catch(Exception e) {result[col++] = Double.NaN;}
result[col++] = ssdf[0] / totalss;
results[i-1] = result;
}
result = new Object[heads.length];
int col = 0;
result[col++] = traitname;
result[col++] = "Model";
result[col++] = modelss;
result[col++] = modeldf;
result[col++] = modelss / modeldf;
double F = modelss / modeldf / errorss * errordf;
result[col++] = F;
try {result[col] = LinearModelUtils.Ftest(F, modeldf, errordf);}
catch(Exception e) {result[col] = Double.NaN;}
col++;
result[col++] = modelss / totalss;
results[numberOfEffects - 1] = result;
result = new Object[heads.length];
col = 0;
result[col++] = traitname;
result[col++] = "Error";
result[col++] = errorss;
result[col++] = errordf;
result[col++] = errorss / errordf;
result[col++] = " ";
result[col++] = " ";
result[col++] = " ";
results[numberOfEffects] = result;
return new SimpleTableReport(title, heads, results);
}
public SweepFastLinearModel getLinearModel() {
return lm;
}
public ArrayList getModelEffects() {
return modelEffects;
}
public double getEnterLimit() {
return enterLimit;
}
public void setEnterLimit(double enterLimit) {
this.enterLimit = enterLimit;
}
public double getExitLimit() {
return exitLimit;
}
public void setExitLimit(double exitLimit) {
this.exitLimit = exitLimit;
}
}
© 2015 - 2025 Weber Informatics LLC | Privacy Policy