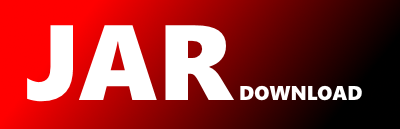
net.maizegenetics.stats.EMMA.EMMAforDoubleMatrix Maven / Gradle / Ivy
package net.maizegenetics.stats.EMMA;
import net.maizegenetics.matrixalgebra.Matrix.DoubleMatrix;
import net.maizegenetics.matrixalgebra.Matrix.DoubleMatrixFactory;
import net.maizegenetics.matrixalgebra.decomposition.EigenvalueDecomposition;
import net.maizegenetics.stats.linearmodels.LinearModelUtils;
import org.apache.logging.log4j.LogManager;
import org.apache.logging.log4j.Logger;
import java.util.ArrayList;
import java.util.Arrays;
import java.util.stream.IntStream;
/**
* Class for implementation of the EMMA algorithm often used in GWAS. Initially developer by
* Kang et al Genetics March 2008 178:1709-1723
*/
public class EMMAforDoubleMatrix {
private static final Logger myLogger = LogManager.getLogger(EMMAforDoubleMatrix.class);
protected DoubleMatrix y;
protected double[] lambda;
protected double[] eta2;
protected double c;
protected int N;
protected int q;
protected int Nran;
protected int dfMarker = 0;
protected DoubleMatrix Xoriginal = null;
// protected DoubleMatrix A;
protected DoubleMatrix X;
protected DoubleMatrix Zoriginal = null;
protected DoubleMatrix Z = null;
// protected DoubleMatrix transZ;
protected DoubleMatrix K;
protected EigenvalueDecomposition eig;
protected EigenvalueDecomposition eigA;
protected DoubleMatrix U;
protected DoubleMatrix invH;
protected DoubleMatrix invXHX;
protected DoubleMatrix beta;
protected DoubleMatrix Xbeta;
protected double ssModel;
protected double ssError;
protected double SST;
protected double Rsq;
protected int dfModel;
protected int dfError;
protected double delta;
protected double varResidual;
protected double varRandomEffect;
protected DoubleMatrix blup;
protected DoubleMatrix pred;
protected DoubleMatrix res;
protected DoubleMatrix pev;
protected double lnLikelihood;
protected boolean findDelta = true;
protected boolean calculatePEV = false;
protected double lowerlimit = 1e-5;
protected double upperlimit = 1e5;
protected int nregions = 100;
protected double convergence = 1e-10;
protected int maxiter = 50;
protected int subintervalCount = 0;
public EMMAforDoubleMatrix(DoubleMatrix y, DoubleMatrix fixed, DoubleMatrix kin, int nAlleles) {
this(y, fixed, kin, nAlleles, Double.NaN);
}
/**
* This constructor assumes that Z is the identity matrix for calculating blups, predicted values and residuals. If that is not true use
* the contstructor that explicity takes Z. This constructor treats A as ZKZ' so it can be used if blups and residuals are not needed.
* @param data
* @param fixed
* @param kin
* @param nAlleles
* @param delta
*/
public EMMAforDoubleMatrix(DoubleMatrix data, DoubleMatrix fixed, DoubleMatrix kin, int nAlleles, double delta) {
//throw an error if X is less than full column rank
dfModel = fixed.numberOfColumns();
int rank = fixed.columnRank();
if (rank < dfModel) throw new IllegalArgumentException("The fixed effect design matrix has less than full column rank. The analysis will not be run.");
if (!Double.isNaN(delta)) {
this.delta = delta;
findDelta = false;
}
y = data;
if (y.numberOfColumns() > 1 && y.numberOfRows() == 1) this.y = y.transpose();
N = y.numberOfRows();
X = fixed;
q = X.numberOfColumns();
// A = kin;
K = kin;
Nran = K.numberOfRows();
Z = DoubleMatrixFactory.DEFAULT.identity(Nran);
dfMarker = nAlleles - 1;
init();
}
/**
* This constructor should be used when Z is not the identity matrix. Z is needed to calculate blups and residuals.
* @param data
* @param fixed
* @param kin
* @param inZ
* @param nAlleles
* @param delta
*/
public EMMAforDoubleMatrix(DoubleMatrix data, DoubleMatrix fixed, DoubleMatrix kin, DoubleMatrix inZ, int nAlleles, double delta) {
dfModel = fixed.numberOfColumns();
int rank = fixed.columnRank();
if (rank < dfModel) throw new IllegalArgumentException("The fixed effect design matrix has less than full column rank. The analysis will not be run.");
if (!Double.isNaN(delta)) {
this.delta = delta;
findDelta = false;
}
y = data;
if (y.numberOfColumns() > 1 && y.numberOfRows() == 1) this.y = y.transpose();
N = y.numberOfRows();
X = fixed;
q = X.numberOfColumns();
Z = inZ;
K = kin;
// A = Z.mult(K).tcrossproduct(Z);
Nran = Z.numberOfRows();
dfMarker = nAlleles - 1;
init();
}
/**
* This constructor is designed for G-BLUP.
* Uses kinship matrix with phenotypes in same order.
* Phenotypes = NaN for prediction set.
* Assumes that Z is the identity matrix for calculating blups, predicted values and residuals. If that is not true use
* the constructor that explicity takes Z. This constructor treats A as ZKZ' so it can be used if blups and residuals are not needed.
* @param data A column double matrix of phenotypic values (number of individuals x 1 taxa ID column + number of traits)
* @param fixed
* @param kin
*/
public EMMAforDoubleMatrix(DoubleMatrix data, DoubleMatrix fixed, DoubleMatrix kin) {
//throw an error if X is less than full column rank
dfModel = fixed.numberOfColumns();
int rank = fixed.columnRank();
if (rank < dfModel) throw new IllegalArgumentException("The fixed effect design matrix has less than full column rank. The analysis will not be run.");
if (data.numberOfColumns() > 1 && data.numberOfRows() == 1)
throw new IllegalArgumentException("The phenotype data must be a column matrix.");
//remove rows in data with missing phenotypic values from Y and Z
K = kin;
Nran = K.numberOfRows();
//int nonmissingY = (int) Arrays.stream(data.to1DArray()).filter(d -> ! Double.isNaN(d)).count();
//int[] nonmissingIndex = new int[nonmissingY];
int[] nonmissingIndex = IntStream.range(0, Nran).filter(i -> ! Double.isNaN(data.get(i,0))).toArray();
y = data.getSelection(nonmissingIndex, null);
Zoriginal = DoubleMatrixFactory.DEFAULT.identity(Nran);
Z = Zoriginal.getSelection(nonmissingIndex, null);
N = y.numberOfRows();
Xoriginal = fixed;
X = fixed.getSelection(nonmissingIndex, null);
q = X.numberOfColumns();
init();
}
protected void init() {
int nreml = N - q;
c = nreml * Math.log(nreml / 2 / Math.PI) - nreml;
lambda = new double[nreml];
//find the eigenvalues of A
DoubleMatrix A = Z.mult(K).tcrossproduct(Z);
eigA = A.getEigenvalueDecomposition();
double[] eigenvalA = eigA.getEigenvalues();
int n = eigenvalA.length;
double min = eigenvalA[0];
for (int i = 1; i < n; i++) min = Math.min(min, eigenvalA[i]);
double bend = 0.0;
if (min < 0.01) bend = -1 * min + 0.5;
//S = I - X inv(X'X) X'
//X is assumed to be of full column rank, i.e. X'X is non-singular
DoubleMatrix[] XtXGM = X.getXtXGM();
DoubleMatrix XtX = XtXGM[0];
DoubleMatrix S = XtXGM[2];
DoubleMatrix G = XtXGM[1];
//determine the s
//add bend to the diagonal of A
//this is necessary to get correct decomposition of SAS
n = A.numberOfRows();
for (int i = 0; i < n; i++) A.set(i, i, A.get(i, i) + bend);
DoubleMatrix SAS = S.mult(A.mult(S));
//decompose SAS
eig = SAS.getEigenvalueDecomposition();
//which are the zero eigenvalues?
double[] eigenval = eig.getEigenvalues();
int[] ndx = getSortedIndexofAbsoluteValues(eigenval);
int[] eigndx = new int[nreml];
for (int i = 0; i < nreml; i++) eigndx[i] = ndx[i];
//sort V to get U
DoubleMatrix V = eig.getEigenvectors();
U = V.getSelection(null, ndx);
//derive lambda
for (int i = 0; i < nreml; i++) lambda[i] = eigenval[eigndx[i]] - bend;
}
private int[] getSortedIndexofAbsoluteValues(double[] values) {
int n = values.length;
int[] index = new int[n];
class Pair implements Comparable {
int order;
double absvalue;
Pair(int order, double value){
this.order = order;
this.absvalue = Math.abs(value);
}
@Override
public int compareTo(Pair other) {
if (absvalue < other.absvalue) return 1;
if (absvalue > other.absvalue) return -1;
return 0;
}
}
Pair[] valuePairs = new Pair[n];
for (int i = 0; i < n; i++) {
valuePairs[i] = new Pair(i, values[i]);
}
Arrays.sort(valuePairs);
for (int i = 0; i < n; i++) index[i] = valuePairs[i].order;
return index;
}
public void solve() {
//calculate eta squared
DoubleMatrix eta = U.crossproduct(y);
int nrows = eta.numberOfRows();
eta2 = new double[nrows];
for (int i = 0; i < nrows; i++) eta2[i] = eta.get(i, 0) * eta.get(i, 0);
if (findDelta) {
double[] interval = new double[]{lowerlimit, upperlimit};
delta = findDeltaInInterval(interval);
}
lnLikelihood = lnlk(delta);
invH = inverseH(delta);
beta = calculateBeta();
double genvar = getGenvar(beta);
dfModel = q - 1;
dfError = N - q;
varResidual = genvar * delta;
varRandomEffect = genvar;
}
public void calculateBlupsPredictedResiduals() {
calculateBLUP();
pred = calculatePred();
res = calculateRes();
}
public void calculateBlupsPredicted() {
calculateBLUP();
pred = calculatePred();
}
private double findDeltaInInterval(double[] interval) {
double[][] d = scanlnlk(interval[0], interval[1]);
double[][] sgnchange = findSignChanges(d);
int nchanges = sgnchange.length;
double[] bestd = new double[]{Double.NaN, Double.NaN, Double.NaN};
int n = d.length;
//find the element of d with maximum ln Likelihood (bestd)
for (int i = 0; i < n; i++) {
if (Double.isNaN(bestd[1])) bestd = d[i];
else if (!Double.isNaN(d[i][1]) && d[i][1] > bestd[1]) bestd = d[i];
}
double bestdelta = bestd[0];
double lkDelta = bestd[1];
for (int i = 0; i < nchanges; i++) {
double newdelta = findMaximum(sgnchange[i]);
if (!Double.isNaN(newdelta)) {
double newlk = lnlk(newdelta);
if (!Double.isNaN(newlk) && newlk > lkDelta) {
bestdelta = newdelta;
lkDelta = newlk;
}
}
}
return bestdelta;
}
private double lnlk(double delta) {
double term1 = 0;
double term2 = 0;
int n = N - q;
for (int i = 0; i < n; i++) {
double val = (lambda[i] + delta);
if (val < 0) return Double.NaN;
term1 += eta2[i] / val;
term2 += Math.log(val);
}
return (c - n * Math.log(term1) - term2) / 2;
}
private double d1lnlk(double delta) {
double term1 = 0;
double term2 = 0;
double term3 = 0;
int n = N - q;
for (int i = 0; i < n; i++) {
double val = 1 / (lambda[i] + delta);
double val2 = eta2[i] * val;
term1 += val2;
term2 += val2 * val;
term3 += val;
}
return n * term2 / term1 / 2 - term3 / 2;
}
private double[][] scanlnlk(double lower, double upper) {
double[][] result = new double[nregions][3];
upper = Math.log10(upper);
lower = Math.log10(lower);
double incr = (upper - lower) / (nregions - 1);
for (int i = 0; i < nregions; i++) {
double delta = Math.pow(10.0, lower + i * incr);
result[i][0] = delta;
result[i][1] = lnlk(delta);
result[i][2] = d1lnlk(delta);
}
return result;
}
private double[][] findSignChanges(double[][] scan) {
ArrayList changes = new ArrayList();
int n = scan.length;
for (int i = 0; i < n - 1; i++) {
if (scan[i][2] > 0 && scan[i+1][2] <= 0 && !Double.isNaN(scan[i][1])) changes.add(new Double[]{scan[i][0], scan[i+1][0]});
}
n = changes.size();
double[][] result = new double[n][2];
for (int i = 0; i < n; i++) {
result[i][0] = changes.get(i)[0];
result[i][1] = changes.get(i)[1];
}
return result;
}
private double findMaximum(double[] interval) {
//uses Newton Raphson to find local maximum
//updates delta using delta' = delta - d1/d2
//where d1 is the first derivative of lnlk at delta and
//d2 is the second derivative of lnlk at delta
//the local maximum is expected to fall between delta and max
//if the algorithm finds a new delta outside this interval
//then the likelihood is not well behaved in this interval
//subdivide the interval and try again
double delta = interval[0];
boolean end = false;
int n = N - q;
int nIterations = 0;
while (!end && nIterations < maxiter) {
//A = sum[eta2/(lambda + delta)]
//B = sum[eta2/(lambda + delta)^2]
//C = sum[eta2/(lambda + delta)^3]
//D = sum[1/(lambda + delta)]
//E = sum[1/(lambda + delta)^2]
double A = 0;
double B = 0;
double C = 0;
double D = 0;
double E = 0;
for (int i = 0; i < n; i++) {
double val = lambda[i] + delta;
double val2 = val * val;
double val3 = val2 * val;
A += eta2[i]/val;
B += eta2[i]/val2;
C += eta2[i]/val3;
D += 1/val;
E += 1/val2;
}
double d1 = n*B/A - D;
if (Math.abs(d1) < convergence) end = true;
else {
double d2 = E + n*(B*B - 2*A*C)/A/A;
delta = delta - d1/d2;
}
if (delta < interval[0] || delta > interval[1]) {
subintervalCount++;
if (subintervalCount > 3) {
subintervalCount = 0;
return Double.NaN;
}
delta = findDeltaInInterval(interval);
end = true;
}
nIterations++;
}
subintervalCount = 0;
return delta;
}
private DoubleMatrix inverseH(double delta) {
DoubleMatrix V = eigA.getEigenvectors();
DoubleMatrix D = eigA.getEigenvalueMatrix();
int n = D.numberOfRows();
for (int i = 0; i < n; i++) D.set(i, i, 1 / (D.get(i, i) + delta));
return V.mult(D.tcrossproduct(V));
}
private DoubleMatrix calculateBeta() {
DoubleMatrix XtH = X.crossproduct(invH);
invXHX = XtH.mult(X).inverse();
return invXHX.mult(XtH.mult(y));
}
public void calculateBLUP(){
Xbeta = X.mult(beta);
DoubleMatrix YminusXbeta = y.minus(Xbeta);
DoubleMatrix KtransZ = K.mult(Z.transpose());
DoubleMatrix KtransZinvH = KtransZ.mult(invH);
blup = KtransZinvH.mult(YminusXbeta);
if (calculatePEV) {
DoubleMatrix KZHX = KtransZinvH.mult(X);
DoubleMatrix pevMatrix = K.copy();
pevMatrix = pevMatrix.minus(KtransZinvH.tcrossproduct(KtransZ));
pevMatrix = pevMatrix.minus(KZHX.mult(invXHX).tcrossproduct(KZHX));
int size = pevMatrix.numberOfRows();
pev = DoubleMatrixFactory.DEFAULT.make(size, 1);
for (int i = 0; i < size; i++) pev.set(i, 0, pevMatrix.get(i, i));
pev.scalarMultEquals(varRandomEffect);
}
}
private DoubleMatrix calculatePred(){
if (Xoriginal == null){
Xbeta = X.mult(beta);
DoubleMatrix Zu = Z.mult(blup);
return Xbeta.plus(Zu);
}else{
Xbeta = Xoriginal.mult(beta);
//System.out.printf("Dimensions of Xbeta are %d,%d\n",Xbeta.numberOfRows(),Xbeta.numberOfColumns());
DoubleMatrix Zu = Zoriginal.mult(blup);
//System.out.printf("Dimensions of Zu are %d,%d\n",Zu.numberOfRows(),Zu.numberOfColumns());
return Xbeta.plus(Zu);
}
}
private DoubleMatrix calculateRes(){
return y.minus(pred);
}
private double getGenvar(DoubleMatrix beta) {
DoubleMatrix res = y.copy();
res.minusEquals(X.mult(beta));
return res.crossproduct(invH.mult(res)).get(0,0) / (N - q);
}
public int getDfMarker() {
return dfMarker;
}
public DoubleMatrix getBeta() {
return beta;
}
public int getDfModel() {
return dfModel;
}
public int getDfError() {
return dfError;
}
public double getDelta() {
return delta;
}
public DoubleMatrix getInvH() {
return invH;
}
public double getVarRes() {
return varResidual;
}
public double getVarRan() {
return varRandomEffect;
}
public DoubleMatrix getBlup() {
return blup;
}
public DoubleMatrix getPev() {
return pev;
}
public DoubleMatrix getPred() {
return pred;
}
public DoubleMatrix getRes() {
return res;
}
public double getLnLikelihood() {
return lnLikelihood;
}
/**
* @return For markers with 2 df, F.fullModel, p.fullModel, additive effect, Fadd, padd, dominance effect, Fdom, pdom
* For markers with other than 2 df, F and p for the full model only
*/
public double[] getMarkerFp() {
if (dfMarker < 1) return new double[]{Double.NaN, Double.NaN, Double.NaN};
int nparm = beta.numberOfRows();
int firstmarker = nparm - dfMarker;
DoubleMatrix M = DoubleMatrixFactory.DEFAULT.make(dfMarker, nparm);
for (int i = 0; i < dfMarker; i++) M.set(i, i + firstmarker, 1);
DoubleMatrix MB = M.mult(beta);
DoubleMatrix invMiM = M.mult(invXHX.tcrossproduct(M));
invMiM.invert();
double F = MB.crossproduct(invMiM.mult(MB)).get(0,0);
F /= varRandomEffect;
F /= dfMarker;
double p;
try {
p = LinearModelUtils.Ftest(F, dfMarker, N - q);
} catch (Exception e) {p = Double.NaN;}
if (dfMarker != 2) return new double[]{F,p};
//calculate add and dom effects and tests
//assumes the betas are for the two homozygous classes and that the het effect = 0;
//additive test
double Fadd, Fdom, padd, pdom;
M = DoubleMatrixFactory.DEFAULT.make(1, nparm, 0);
M.set(0, nparm - 2, 0.5);
M.set(0, nparm - 1, -0.5);
MB = M.mult(beta);
double addEffect = MB.get(0, 0);
try {
Fadd = addEffect * addEffect / (M.mult(invXHX.tcrossproduct(M))).get(0,0) / varRandomEffect;
} catch (Exception ex) {
Fadd = Double.NaN;
}
try {
padd = LinearModelUtils.Ftest(Fadd, 1, N - q);
} catch (Exception e) {
padd = Double.NaN;
}
//dominance test
M = DoubleMatrixFactory.DEFAULT.make(1, nparm, 0);
M.set(0, nparm - 2, -0.5);
M.set(0, nparm - 1, -0.5);
MB = M.mult(beta);
double domEffect = MB.get(0, 0);
try {
Fdom = domEffect * domEffect / (M.mult(invXHX.tcrossproduct(M))).get(0,0) / varRandomEffect;
} catch (Exception ex) {
Fdom = Double.NaN;
}
try {
pdom = LinearModelUtils.Ftest(Fdom, 1, N - q);
} catch (Exception e) {
pdom = Double.NaN;
}
return new double[]{F,p,addEffect,Fadd,padd,domEffect,Fdom,pdom};
}
public void solveWithNewData(DoubleMatrix y) {
this.y = y;
solve();
}
public void setCalculatePEV(boolean calculatePEV) {
this.calculatePEV = calculatePEV;
}
}
© 2015 - 2025 Weber Informatics LLC | Privacy Policy