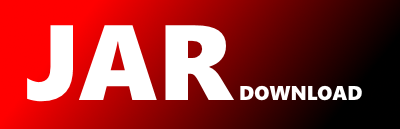
mikera.matrixx.decompose.impl.svd.SvdImplicitQr Maven / Gradle / Ivy
Go to download
Show more of this group Show more artifacts with this name
Show all versions of vectorz Show documentation
Show all versions of vectorz Show documentation
Fast double-precision vector and matrix maths library for Java, supporting N-dimensional numeric arrays.
/*
* Copyright (c) 2009-2013, Peter Abeles. All Rights Reserved.
*
* This file is part of Efficient Java Matrix Library (EJML).
*
* Licensed under the Apache License, Version 2.0 (the "License");
* you may not use this file except in compliance with the License.
* You may obtain a copy of the License at
*
* http://www.apache.org/licenses/LICENSE-2.0
*
* Unless required by applicable law or agreed to in writing, software
* distributed under the License is distributed on an "AS IS" BASIS,
* WITHOUT WARRANTIES OR CONDITIONS OF ANY KIND, either express or implied.
* See the License for the specific language governing permissions and
* limitations under the License.
*/
package mikera.matrixx.decompose.impl.svd;
import mikera.matrixx.AMatrix;
import mikera.matrixx.Matrix;
import mikera.matrixx.decompose.Bidiagonal;
import mikera.matrixx.decompose.IBidiagonalResult;
import mikera.vectorz.AVector;
import mikera.vectorz.Vector;
/**
*
* Computes the Singular value decomposition of a matrix using the implicit QR algorithm
* for singular value decomposition. It works by first by transforming the matrix
* to a bidiagonal A=U*B*VT form, then it implicitly computing the eigenvalues of the BTB matrix,
* which are the same as the singular values in the original A matrix.
*
*
*
* Based off of the description provided in:
*
* David S. Watkins, "Fundamentals of Matrix Computations," Second Edition. Page 404-411
*
*
* @author Peter Abeles
*/
public class SvdImplicitQr {
private int numRows;
private int numCols;
// dimensions of transposed matrix
private int numRowsT;
private int numColsT;
// if true then it can use the special Bidiagonal decomposition
// private boolean canUseTallBidiagonal;
// If U is not being computed and the input matrix is 'tall' then a special bidiagonal decomposition
// can be used which is faster.
private IBidiagonalResult bidiagResult;
private SvdImplicitQrAlgorithm qralg = new SvdImplicitQrAlgorithm();
double diag[];
double off[];
private Matrix Ut;
private Matrix Vt;
private double singularValues[];
private int numSingular;
// compute a compact SVD
private boolean compact;
// What is actually computed
// private boolean computeU;
// private boolean computeV;
// What the user requested to be computed
// If the transpose is computed instead then what is actually computed is swapped
// private boolean prefComputeU;
// private boolean prefComputeV;
// Should it compute the transpose instead
private boolean transposed;
// Either a copy of the input matrix or a copy of it transposed
private Matrix A_mod = Matrix.create(1,1);
public static SVDResult decompose(AMatrix A, boolean compact) {
SvdImplicitQr svd = new SvdImplicitQr(compact);
return svd._decompose(A);
}
/**
* Configures the class
*
* @param compact Compute a compact SVD
* @param computeU If true it will compute the U matrix
* @param computeV If true it will compute the V matrix
*/
/*Once BidiagonalDecomposition is implemented, use the commented out constructor
and add:
"@param canUseTallBidiagonal If true then it can choose to use a tall Bidiagonal decomposition to improve runtime performance."
to doc*/
// public SvdImplicitQr(boolean compact, boolean computeU, boolean computeV,
// boolean canUseTallBidiagonal )
public SvdImplicitQr(boolean compact) {
this.compact = compact;
// this.prefComputeU = computeU;
// this.prefComputeV = computeV;
// this.canUseTallBidiagonal = canUseTallBidiagonal;
}
public AVector getSingularValues() {
return Vector.wrap(singularValues);
}
public int numberOfSingularValues() {
return numSingular;
}
public boolean isCompact() {
return compact;
}
public AMatrix getU() {
// if( !prefComputeU )
// throw new IllegalArgumentException("As requested U was not computed.");
return Ut.getTranspose();
}
public AMatrix getV() {
// if( !prefComputeV )
// throw new IllegalArgumentException("As requested V was not computed.");
return Vt.getTranspose();
}
public AMatrix getS() {
int m = compact ? numSingular : numRows;
int n = compact ? numSingular : numCols;
Matrix S = Matrix.create(m,n);
for( int i = 0; i < numSingular; i++ ) {
S.unsafeSet(i,i, singularValues[i]);
}
return S;
}
public SVDResult _decompose(AMatrix _orig) {
// Creating a copy so that original matrix is not modified
Matrix orig = _orig.copy().toMatrix();
setup(orig);
if (bidiagonalization(orig)) {
return null;
}
if( computeUSV() )
return null;
// make sure all the singular values or positive
makeSingularPositive();
// if transposed undo the transposition
undoTranspose();
return new SVDResult(getU(), getS(), getV(), getSingularValues());
}
private boolean bidiagonalization(Matrix orig) {
// change the matrix to bidiagonal form
if( transposed ) {
A_mod = orig.getTransposeCopy().toMatrix();
} else {
A_mod = orig.copy().toMatrix();
}
bidiagResult = Bidiagonal.decompose(A_mod, compact);
return bidiagResult == null;
}
/**
* If the transpose was computed instead do some additional computations
*/
private void undoTranspose() {
if( transposed ) {
Matrix temp = Vt;
Vt = Ut;
Ut = temp;
}
}
/**
* Compute singular values and U and V at the same time
*/
private boolean computeUSV() {
diag = bidiagResult.getB().getBand(0).toDoubleArray();
off = bidiagResult.getB().getBand(1).toDoubleArray();
qralg.setMatrix(numRowsT,numColsT,diag,off);
// long pointA = System.currentTimeMillis();
// compute U and V matrices
// if( computeU )
Ut = bidiagResult.getU().getTranspose().toMatrix();
// if( computeV )
Vt = bidiagResult.getV().getTranspose().toMatrix();
qralg.setFastValues(false);
// if( computeU )
qralg.setUt(Ut);
// else
// qralg.setUt(null);
// if( computeV )
qralg.setVt(Vt);
// else
// qralg.setVt(null);
// long pointB = System.currentTimeMillis();
boolean ret = !qralg.process();
// long pointC = System.currentTimeMillis();
// System.out.println(" compute UV "+(pointB-pointA)+" QR = "+(pointC-pointB));
return ret;
}
private void setup(Matrix orig) {
transposed = orig.columnCount() > orig.rowCount();
// flag what should be computed and what should not be computed
if( transposed ) {
// computeU = prefComputeV;
// computeV = prefComputeU;
numRowsT = orig.columnCount();
numColsT = orig.rowCount();
} else {
// computeU = prefComputeU;
// computeV = prefComputeV;
numRowsT = orig.rowCount();
numColsT = orig.columnCount();
}
numRows = orig.rowCount();
numCols = orig.columnCount();
diag = new double[ numColsT ];
off = new double[ numColsT-1 ];
// if it is a tall matrix and U is not needed then there is faster decomposition algorithm
// if( canUseTallBidiagonal && numRows > numCols * 2 && !computeU ) {
// if( bidiag == null || !(bidiag instanceof BidiagonalDecompositionTall) ) {
// bidiag = new BidiagonalDecompositionTall();
// }
// } else if( bidiag == null || !(bidiag instanceof BidiagonalDecompositionRow) ) {
// bidiag = new BidiagonalDecompositionRow();
// }
// TODO: ^Choose between BidiagonalTall and BidiagonalRow once BidiagonalTall
// is implemented
}
/**
* With the QR algorithm it is possible for the found singular values to be negative. This
* makes them all positive by multiplying it by a diagonal matrix that has
*/
private void makeSingularPositive() {
numSingular = qralg.getNumberOfSingularValues();
singularValues = qralg.getSingularValues();
double[] UtData = Ut.asDoubleArray();
for( int i = 0; i < numSingular; i++ ) {
double val = qralg.getSingularValue(i);
if( val < 0 ) {
singularValues[i] = 0.0d - val;
// if( computeU ) {
// compute the results of multiplying it by an element of -1 at this location in
// a diagonal matrix.
int start = i* Ut.columnCount();
int stop = start+ Ut.columnCount();
for( int j = start; j < stop; j++ ) {
UtData[j] = 0.0d - UtData[j];
// }
}
} else {
singularValues[i] = val;
}
}
}
public int numRows() {
return numRows;
}
public int numCols() {
return numCols;
}
}
© 2015 - 2025 Weber Informatics LLC | Privacy Policy