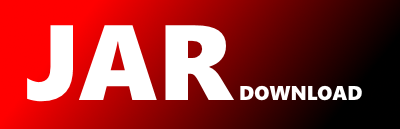
mikera.matrixx.decompose.impl.eigen.SymmetricQRAlgorithmDecomposition Maven / Gradle / Ivy
Go to download
Fast double-precision vector and matrix maths library for Java, supporting N-dimensional numeric arrays.
Please wait ...
© 2015 - 2025 Weber Informatics LLC | Privacy Policy