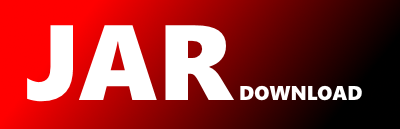
mulan.classifier.neural.BPMLL Maven / Gradle / Ivy
Go to download
Show more of this group Show more artifacts with this name
Show all versions of mulan Show documentation
Show all versions of mulan Show documentation
Mulan is an open-source Java library for learning from multi-label datasets.
The newest version!
/*
* This program is free software; you can redistribute it and/or modify
* it under the terms of the GNU General Public License as published by
* the Free Software Foundation; either version 2 of the License, or
* (at your option) any later version.
*
* This program is distributed in the hope that it will be useful,
* but WITHOUT ANY WARRANTY; without even the implied warranty of
* MERCHANTABILITY or FITNESS FOR A PARTICULAR PURPOSE. See the
* GNU General Public License for more details.
*
* You should have received a copy of the GNU General Public License
* along with this program; if not, write to the Free Software
* Foundation, Inc., 675 Mass Ave, Cambridge, MA 02139, USA.
*/
/*
* BPMLL.java
* Copyright (C) 2009-2012 Aristotle University of Thessaloniki, Greece
*/
package mulan.classifier.neural;
import java.util.*;
import mulan.classifier.InvalidDataException;
import mulan.classifier.MultiLabelLearnerBase;
import mulan.classifier.MultiLabelOutput;
import mulan.classifier.neural.model.ActivationTANH;
import mulan.classifier.neural.model.BasicNeuralNet;
import mulan.classifier.neural.model.NeuralNet;
import mulan.core.WekaException;
import mulan.data.DataUtils;
import mulan.data.InvalidDataFormatException;
import mulan.data.MultiLabelInstances;
import weka.core.Attribute;
import weka.core.Instance;
import weka.core.Instances;
import weka.core.TechnicalInformation;
import weka.core.TechnicalInformation.Field;
import weka.core.TechnicalInformation.Type;
import weka.filters.Filter;
import weka.filters.unsupervised.attribute.NominalToBinary;
/**
* The implementation of Back-Propagation Multi-Label Learning (BPMLL) learner. The learned model is stored in {@link NeuralNet} neural network. The models of the learner built by {@link BPMLLAlgorithm} from given training data set.
*
*
* BibTeX:
*
* @article{Zhang2006,
* author = {Zhang, M.L., Zhou, Z.H.},
* journal = {IEEE Transactions on Knowledge and Data Engineering},
* pages = {1338-1351},
* title = {Multi-label neural networks with applications to functional genomics and text categorization},
* volume = {18},
* year = {2006}
* }
*
*
*
* @see BPMLLAlgorithm
* @author Jozef Vilcek
* @version 2012.02.27
*/
public class BPMLL extends MultiLabelLearnerBase {
private static final long serialVersionUID = 2153814250172139021L;
private static final double NET_BIAS = 1;
private static final double ERROR_SMALL_CHANGE = 0.000001;
// filter used to convert nominal input attributes into binary-numeric
private NominalToBinary nominalToBinaryFilter;
// algorithm parameters
private int epochs = 100;
private final Long randomnessSeed;
private double weightsDecayCost = 0.00001;
private double learningRate = 0.05;
private int[] hiddenLayersTopology;
// members related to normalization or attributes
private boolean normalizeAttributes = true;
private NormalizationFilter normalizer;
private NeuralNet model;
private ThresholdFunction thresholdF;
/**
* Creates a new instance of {@link BPMLL} learner.
*/
public BPMLL() {
randomnessSeed = null;
}
/**
* Creates a new instance of {@link BPMLL} learner.
* @param randomnessSeed the seed value for pseudo-random generator
*/
public BPMLL(long randomnessSeed) {
this.randomnessSeed = randomnessSeed;
}
/**
* Sets the topology of hidden layers for neural network.
* The length of passed array defines number of hidden layers.
* The value at particular index of array defines number of neurons in that layer.
* If null
is specified, no hidden layers will be created.
*
* The network is created when learner is being built.
* The input and output layer is determined from input training data.
*
* @param hiddenLayers
* @throws IllegalArgumentException if any value in the array is less or equal to zero
*/
public void setHiddenLayers(int[] hiddenLayers) {
if (hiddenLayers != null) {
for (int value : hiddenLayers) {
if (value <= 0) {
throw new IllegalArgumentException("Invalid hidden layer topology definition. " +
"Number of neurons in hidden layer must be larger than zero.");
}
}
}
hiddenLayersTopology = hiddenLayers;
}
/**
* Gets an array defining topology of hidden layer of the underlying neural model.
*
* @return The method returns a copy of the array.
*/
public int[] getHiddenLayers() {
return hiddenLayersTopology == null ? hiddenLayersTopology : Arrays.copyOf(hiddenLayersTopology, hiddenLayersTopology.length);
}
/**
* Sets the learning rate. Must be greater than 0 and no more than 1.
* Default value is 0.05.
*
* @param learningRate the learning rate
* @throws IllegalArgumentException if passed value is invalid
*/
public void setLearningRate(double learningRate) {
if (learningRate <= 0 || learningRate > 1) {
throw new IllegalArgumentException("The learning rate must be greater than 0 and no more than 1. " +
"Entered value is : " + learningRate);
}
this.learningRate = learningRate;
}
/**
* Gets the learning rate. The default value is 0.05.
* @return learning rate
*/
public double getLearningRate() {
return learningRate;
}
/**
* Sets the regularization cost term for weights decay.
* Must be greater than 0 and no more than 1.
* Default value is 0.00001.
*
* @param weightsDecayCost the weights decay cost term
* @throws IllegalArgumentException if passed value is invalid
*/
public void setWeightsDecayRegularization(double weightsDecayCost) {
if (weightsDecayCost <= 0 || weightsDecayCost > 1) {
throw new IllegalArgumentException("The weights decay regularization cost " +
"term must be greater than 0 and no more than 1. " +
"The passed value is : " + weightsDecayCost);
}
this.weightsDecayCost = weightsDecayCost;
}
/**
* Gets a value of the regularization cost term for weights decay.
* @return regularization cost
*/
public double getWeightsDecayRegularization() {
return weightsDecayCost;
}
/**
* Sets the number of training epochs. Must be greater than 0.
* Default value is 100.
*
* @param epochs the number of training epochs
* @throws IllegalArgumentException if passed value is invalid
*/
public void setTrainingEpochs(int epochs) {
if (epochs <= 0) {
throw new IllegalArgumentException("The number of training epochs must be greater than zero. " +
"Entered value is : " + epochs);
}
this.epochs = epochs;
}
/**
* Gets number of training epochs.
* Default value is 100.
* @return training epochs
*/
public int getTrainingEpochs() {
return epochs;
}
/**
* Sets whether attributes of instances data (except label attributes) should
* be normalized prior to building the learner. Normalization is performed
* on numeric attributes to the range {-1,1}).
* When making prediction, attributes of passed input instance are also
* normalized prior to making prediction.
* Default is true (normalization of attributes takes place).
*
* @param normalize flag if normalization of attributes should be used
* @throws IllegalArgumentException if passed value is invalid
*/
public void setNormalizeAttributes(boolean normalize) {
normalizeAttributes = normalize;
}
/**
* Gets a value if normalization of nominal attributes should take place.
* Default value is true.
* @return a value if normalization of nominal attributes should take place
*/
public boolean getNormalizeAttributes() {
return normalizeAttributes;
}
protected void buildInternal(final MultiLabelInstances instances) throws Exception {
// delete filter if available from previous build, a new one will be created if necessary
nominalToBinaryFilter = null;
MultiLabelInstances trainInstances = instances.clone();
List trainData = prepareData(trainInstances);
int inputsDim = trainData.get(0).getInput().length;
model = buildNeuralNetwork(inputsDim);
BPMLLAlgorithm learnAlg = new BPMLLAlgorithm(model, weightsDecayCost);
int numInstances = trainData.size();
int processedInstances = 0;
double prevError = Double.MAX_VALUE;
double error = 0;
for (int epoch = 0; epoch < epochs; epoch++) {
Collections.shuffle(trainData, new Random(1));
for (int index = 0; index < numInstances; index++) {
DataPair trainPair = trainData.get(index);
double result = learnAlg.learn(trainPair.getInput(), trainPair.getOutput(), learningRate);
if (!Double.isNaN(result)) {
error += result;
processedInstances++;
}
}
if (getDebug()) {
if (epoch % 10 == 0) {
debug("Training epoch : " + epoch + " Model error : " + error / processedInstances);
}
}
double errorDiff = prevError - error;
if (errorDiff <= ERROR_SMALL_CHANGE * prevError) {
if (getDebug()) {
debug("Global training error does not decrease enough. Training terminated.");
}
break;
}
}
thresholdF = buildThresholdFunction(trainData);
}
public String globalInfo() {
return "The implementation of Back-Propagation Multi-Label Learning "
+ "(BPMLL) learner. The learned model is stored in "
+ "{@link NeuralNet} neural network. The models of the learner "
+ "built by {@link BPMLLAlgorithm} from given training data set.";
}
@Override
public TechnicalInformation getTechnicalInformation() {
TechnicalInformation technicalInfo = new TechnicalInformation(Type.ARTICLE);
technicalInfo.setValue(Field.AUTHOR, "Zhang, M.L., Zhou, Z.H.");
technicalInfo.setValue(Field.YEAR, "2006");
technicalInfo.setValue(Field.TITLE, "Multi-label neural networks with applications to functional genomics and text categorization");
technicalInfo.setValue(Field.JOURNAL, "IEEE Transactions on Knowledge and Data Engineering");
technicalInfo.setValue(Field.VOLUME, "18");
technicalInfo.setValue(Field.PAGES, "1338-1351");
return technicalInfo;
}
private ThresholdFunction buildThresholdFunction(List trainData) {
int numExamples = trainData.size();
double[][] idealLabels = new double[numExamples][numLabels];
double[][] modelConfidences = new double[numExamples][numLabels];
for (int example = 0; example < numExamples; example++) {
DataPair dataPair = trainData.get(example);
idealLabels[example] = dataPair.getOutput();
modelConfidences[example] = model.feedForward(dataPair.getInput());
}
return new ThresholdFunction(idealLabels, modelConfidences);
}
private NeuralNet buildNeuralNetwork(int inputsDim) {
int[] networkTopology;
if (hiddenLayersTopology == null) {
int hiddenUnits = Math.round(0.2f * inputsDim);
hiddenLayersTopology = new int[]{hiddenUnits};
networkTopology = new int[]{inputsDim, hiddenUnits, numLabels};
} else {
networkTopology = new int[hiddenLayersTopology.length + 2];
networkTopology[0] = inputsDim;
System.arraycopy(hiddenLayersTopology, 0, networkTopology, 1, hiddenLayersTopology.length);
networkTopology[networkTopology.length - 1] = numLabels;
}
NeuralNet aModel = new BasicNeuralNet(networkTopology, NET_BIAS, ActivationTANH.class, randomnessSeed == null ? null : new Random(randomnessSeed));
return aModel;
}
/**
* Prepares {@link MultiLabelInstances} data for the learning algorithm.
*
* The data are checked for correct format, label attributes
* are converted to bipolar values. Finally {@link Instance} instances are
* converted to {@link DataPair} instances, which will be used for the algorithm.
*/
private List prepareData(MultiLabelInstances mlData) {
Instances data = mlData.getDataSet();
data = checkAttributesFormat(data, mlData.getFeatureAttributes());
if (data == null) {
throw new InvalidDataException("Attributes are not in correct format. " +
"Input attributes (all but the label attributes) must be nominal or numeric.");
} else {
try {
mlData = mlData.reintegrateModifiedDataSet(data);
this.labelIndices = mlData.getLabelIndices();
} catch (InvalidDataFormatException e) {
throw new InvalidDataException("Failed to create a multilabel data set from modified instances.");
}
if (normalizeAttributes) {
normalizer = new NormalizationFilter(mlData, true, -0.8, 0.8);
}
return DataPair.createDataPairs(mlData, true);
}
}
/**
* Checks {@link Instances} data if attributes (all but the label attributes)
* are numeric or nominal. Nominal attributes are transformed to binary by use of
* {@link NominalToBinary} filter.
*
* @param dataSet instances data to be checked
* @param inputAttributes input/feature attributes which format need to be checked
* @return data set if it passed checks; otherwise null
*/
private Instances checkAttributesFormat(Instances dataSet, Set inputAttributes) {
StringBuilder nominalAttrRange = new StringBuilder();
String rangeDelimiter = ",";
for (Attribute attribute : inputAttributes) {
if (attribute.isNumeric() == false) {
if (attribute.isNominal()) {
nominalAttrRange.append((attribute.index() + 1) + rangeDelimiter);
} else {
// fail check if any other attribute type than nominal or numeric is used
return null;
}
}
}
// convert any nominal attributes to binary
if (nominalAttrRange.length() > 0) {
nominalAttrRange.deleteCharAt(nominalAttrRange.lastIndexOf(rangeDelimiter));
try {
nominalToBinaryFilter = new NominalToBinary();
nominalToBinaryFilter.setAttributeIndices(nominalAttrRange.toString());
nominalToBinaryFilter.setInputFormat(dataSet);
dataSet = Filter.useFilter(dataSet, nominalToBinaryFilter);
} catch (Exception exception) {
nominalToBinaryFilter = null;
if (getDebug()) {
debug("Failed to apply NominalToBinary filter to the input instances data. " +
"Error message: " + exception.getMessage());
}
throw new WekaException("Failed to apply NominalToBinary filter to the input instances data.", exception);
}
}
return dataSet;
}
public MultiLabelOutput makePredictionInternal(Instance instance) throws InvalidDataException {
Instance inputInstance = null;
if (nominalToBinaryFilter != null) {
try {
nominalToBinaryFilter.input(instance);
inputInstance = nominalToBinaryFilter.output();
inputInstance.setDataset(null);
} catch (Exception ex) {
throw new InvalidDataException("The input instance for prediction is invalid. " +
"Instance is not consistent with the data the model was built for.");
}
} else {
inputInstance = DataUtils.createInstance(instance, instance.weight(), instance.toDoubleArray());
}
int numAttributes = inputInstance.numAttributes();
if (numAttributes < model.getNetInputSize()) {
throw new InvalidDataException("Input instance do not have enough attributes " +
"to be processed by the model. Instance is not consistent with the data the model was built for.");
}
// if instance has more attributes than model input, we assume that true outputs
// are there, so we remove them
List someLabelIndices = new ArrayList();
boolean labelsAreThere = false;
if (numAttributes > model.getNetInputSize()) {
for (int index : this.labelIndices) {
someLabelIndices.add(index);
}
labelsAreThere = true;
}
if (normalizeAttributes) {
normalizer.normalize(inputInstance);
}
int inputDim = model.getNetInputSize();
double[] inputPattern = new double[inputDim];
int indexCounter = 0;
for (int attrIndex = 0; attrIndex < numAttributes; attrIndex++) {
if (labelsAreThere && someLabelIndices.contains(attrIndex)) {
continue;
}
inputPattern[indexCounter] = inputInstance.value(attrIndex);
indexCounter++;
}
double[] labelConfidences = model.feedForward(inputPattern);
double threshold = thresholdF.computeThreshold(labelConfidences);
boolean[] labelPredictions = new boolean[numLabels];
Arrays.fill(labelPredictions, false);
for (int labelIndex = 0; labelIndex < numLabels; labelIndex++) {
if (labelConfidences[labelIndex] > threshold) {
labelPredictions[labelIndex] = true;
}
// translate from bipolar output to binary
labelConfidences[labelIndex] = (labelConfidences[labelIndex] + 1) / 2;
}
MultiLabelOutput mlo = new MultiLabelOutput(labelPredictions, labelConfidences);
return mlo;
}
}
© 2015 - 2025 Weber Informatics LLC | Privacy Policy