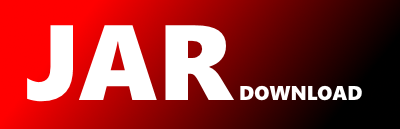
mulan.evaluation.measure.MacroAUC Maven / Gradle / Ivy
Go to download
Show more of this group Show more artifacts with this name
Show all versions of mulan Show documentation
Show all versions of mulan Show documentation
Mulan is an open-source Java library for learning from multi-label datasets.
The newest version!
/*
* This program is free software; you can redistribute it and/or modify
* it under the terms of the GNU General Public License as published by
* the Free Software Foundation; either version 2 of the License, or
* (at your option) any later version.
*
* This program is distributed in the hope that it will be useful,
* but WITHOUT ANY WARRANTY; without even the implied warranty of
* MERCHANTABILITY or FITNESS FOR A PARTICULAR PURPOSE. See the
* GNU General Public License for more details.
*
* You should have received a copy of the GNU General Public License
* along with this program; if not, write to the Free Software
* Foundation, Inc., 675 Mass Ave, Cambridge, MA 02139, USA.
*/
/*
* MacroAUC.java
* Copyright (C) 2009-2012 Aristotle University of Thessaloniki, Greece
*/
package mulan.evaluation.measure;
import weka.classifiers.evaluation.ThresholdCurve;
import weka.core.Instances;
import weka.core.Utils;
/**
* Implementation of the macro-averaged AUC measure.
*
* @author Grigorios Tsoumakas
* @version 2010.12.10
*/
public class MacroAUC extends LabelBasedAUC implements MacroAverageMeasure {
/**
* Creates a new instance of this class
*
* @param numOfLabels the number of labels
*/
public MacroAUC(int numOfLabels) {
super(numOfLabels);
}
public String getName() {
return "Macro-averaged AUC";
}
public double getValue() {
double[] labelAUC = new double[numOfLabels];
for (int i = 0; i < numOfLabels; i++) {
ThresholdCurve tc = new ThresholdCurve();
Instances result = tc.getCurve(m_Predictions[i], 1);
labelAUC[i] = ThresholdCurve.getROCArea(result);
}
return Utils.mean(labelAUC);
}
/**
* Returns the AUC for a particular label
*
* @param labelIndex the index of the label
* @return the AUC for that label
*/
public double getValue(int labelIndex) {
ThresholdCurve tc = new ThresholdCurve();
Instances result = tc.getCurve(m_Predictions[labelIndex], 1);
return ThresholdCurve.getROCArea(result);
}
}
© 2015 - 2025 Weber Informatics LLC | Privacy Policy