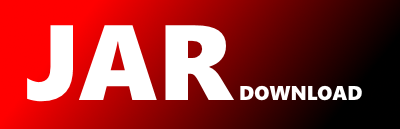
meka.classifiers.multitarget.meta.MultiSearch Maven / Gradle / Ivy
Go to download
Show more of this group Show more artifacts with this name
Show all versions of meka Show documentation
Show all versions of meka Show documentation
The MEKA project provides an open source implementation of methods for multi-label classification and evaluation. It is based on the WEKA Machine Learning Toolkit. Several benchmark methods are also included, as well as the pruned sets and classifier chains methods, other methods from the scientific literature, and a wrapper to the MULAN framework.
The newest version!
/*
* This program is free software: you can redistribute it and/or modify
* it under the terms of the GNU General Public License as published by
* the Free Software Foundation, either version 3 of the License, or
* (at your option) any later version.
*
* This program is distributed in the hope that it will be useful,
* but WITHOUT ANY WARRANTY; without even the implied warranty of
* MERCHANTABILITY or FITNESS FOR A PARTICULAR PURPOSE. See the
* GNU General Public License for more details.
*
* You should have received a copy of the GNU General Public License
* along with this program. If not, see .
*/
/*
* MultiSearch.java
* Copyright (C) 2008-2017 University of Waikato, Hamilton, New Zealand
*/
package meka.classifiers.multitarget.meta;
import meka.classifiers.AbstractMultiSearch;
import meka.classifiers.multilabel.MultiLabelClassifier;
import meka.classifiers.multilabel.ProblemTransformationMethod;
import meka.classifiers.multitarget.MultiTargetClassifier;
import meka.classifiers.multitarget.RAkELd;
import weka.classifiers.Classifier;
import weka.core.RevisionUtils;
import weka.core.SerializedObject;
import weka.core.setupgenerator.AbstractParameter;
import weka.core.setupgenerator.MathParameter;
/**
* Performs a search of an arbitrary number of parameters of a classifier and chooses the best combination found.
* The properties being explored are totally up to the user.
*
* E.g., if you have a FilteredClassifier selected as base classifier, sporting a PLSFilter and you want to explore the number of PLS components, then your property will be made up of the following components:
* - filter: referring to the FilteredClassifier's property (= PLSFilter)
* - numComponents: the actual property of the PLSFilter that we want to modify
* And assembled, the property looks like this:
* filter.numComponents
*
*
* The best classifier setup can be accessed after the buildClassifier call via the getBestClassifier method.
*
* The trace of setups evaluated can be accessed after the buildClassifier call as well, using the following methods:
* - getTrace()
* - getTraceSize()
* - getTraceValue(int)
* - getTraceFolds(int)
* - getTraceClassifierAsCli(int)
* - getTraceParameterSettings(int)
*
* Using the weka.core.setupgenerator.ParameterGroup parameter, it is possible to group dependent parameters. In this case, all top-level parameters must be of type weka.core.setupgenerator.ParameterGroup.
*
*
* Valid options are:
*
*
-E <ACC|JIDX|HSCORE|EM|JDIST|HLOSS|ZOLOSS|HARSCORE|OE|RLOSS|AVGPREC|LOGLOSSL|LOGLOSSD|F1MICRO|F1MACROEX|F1MACROLBL|AUPRC|AUROC|LCARD|LDIST>
* Determines the parameter used for evaluation:
* ACC = Accuracy
* JIDX = Jaccard index
* HSCORE = Hamming score
* EM = Exact match
* JDIST = Jaccard distance
* HLOSS = Hamming loss
* ZOLOSS = ZeroOne loss
* HARSCORE = Harmonic score
* OE = One error
* RLOSS = Rank loss
* AVGPREC = Avg precision
* LOGLOSSL = Log Loss (lim. L)
* LOGLOSSD = Log Loss (lim. D)
* F1MICRO = F1 (micro averaged)
* F1MACROEX = F1 (macro averaged by example)
* F1MACROLBL = F1 (macro averaged by label)
* AUPRC = AUPRC (macro averaged)
* AUROC = AUROC (macro averaged)
* LCARD = Label cardinality (predicted)
* LDIST = Levenshtein distance
* (default: ACC)
*
* -search "<classname options>"
* A property search setup.
*
*
* -algorithm "<classname options>"
* A search algorithm.
*
*
* -log-file <filename>
* The log file to log the messages to.
* (default: none)
*
* -S <num>
* Random number seed.
* (default 1)
*
* -W
* Full name of base classifier.
* (default: meka.classifiers.multitarget.RAkELd)
*
* -output-debug-info
* If set, classifier is run in debug mode and
* may output additional info to the console
*
* -do-not-check-capabilities
* If set, classifier capabilities are not checked before classifier is built
* (use with caution).
*
* -num-decimal-places
* The number of decimal places for the output of numbers in the model (default 2).
*
* -batch-size
* The desired batch size for batch prediction (default 100).
*
*
* Options specific to classifier meka.classifiers.multitarget.RAkELd:
*
*
* -k <num>
* The number of labels in each partition -- should be 1 <= k < (L/2) where L is the total number of labels.
*
* -P <value>
* Sets the pruning value, defining an infrequent labelset as one which occurs <= P times in the data (P = 0 defaults to LC).
* default: 0 (LC)
*
* -N <value>
* Sets the (maximum) number of frequent labelsets to subsample from the infrequent labelsets.
* default: 0 (none)
* n N = n
* -n N = n, or 0 if LCard(D) >= 2
* n-m N = random(n,m)
*
* -S <value>
* The seed value for randomization
* default: 0
*
* -W
* Full name of base classifier.
* (default: weka.classifiers.trees.J48)
*
* -output-debug-info
* If set, classifier is run in debug mode and
* may output additional info to the console
*
* -do-not-check-capabilities
* If set, classifier capabilities are not checked before classifier is built
* (use with caution).
*
* -num-decimal-places
* The number of decimal places for the output of numbers in the model (default 2).
*
* -batch-size
* The desired batch size for batch prediction (default 100).
*
*
* Options specific to classifier weka.classifiers.trees.J48:
*
*
* -U
* Use unpruned tree.
*
* -O
* Do not collapse tree.
*
* -C <pruning confidence>
* Set confidence threshold for pruning.
* (default 0.25)
*
* -M <minimum number of instances>
* Set minimum number of instances per leaf.
* (default 2)
*
* -R
* Use reduced error pruning.
*
* -N <number of folds>
* Set number of folds for reduced error
* pruning. One fold is used as pruning set.
* (default 3)
*
* -B
* Use binary splits only.
*
* -S
* Do not perform subtree raising.
*
* -L
* Do not clean up after the tree has been built.
*
* -A
* Laplace smoothing for predicted probabilities.
*
* -J
* Do not use MDL correction for info gain on numeric attributes.
*
* -Q <seed>
* Seed for random data shuffling (default 1).
*
* -doNotMakeSplitPointActualValue
* Do not make split point actual value.
*
* -output-debug-info
* If set, classifier is run in debug mode and
* may output additional info to the console
*
* -do-not-check-capabilities
* If set, classifier capabilities are not checked before classifier is built
* (use with caution).
*
* -num-decimal-places
* The number of decimal places for the output of numbers in the model (default 2).
*
* -batch-size
* The desired batch size for batch prediction (default 100).
*
*
* @author fracpete (fracpete at waikato dot ac dot nz)
* @version $Revision: 4521 $
*/
public class MultiSearch
extends AbstractMultiSearch
implements MultiLabelClassifier, MultiTargetClassifier {
/** for serialization. */
private static final long serialVersionUID = -5129316523575906233L;
/**
* Returns the default classifier to use.
*
* @return the default classifier
*/
protected Classifier defaultClassifier() {
return new RAkELd();
}
/**
* Returns the default search parameters.
*
* @return the parameters
*/
protected AbstractParameter[] defaultSearchParameters() {
AbstractParameter[] result;
MathParameter param;
result = new AbstractParameter[1];
param = new MathParameter();
param.setProperty("K");
param.setMin(1);
param.setMax(3);
param.setStep(1);
param.setBase(10);
param.setExpression("I");
result[0] = param;
try {
result = (AbstractParameter[]) new SerializedObject(result).getObject();
}
catch (Exception e) {
result = new AbstractParameter[0];
System.err.println("Failed to create copy of default parameters!");
e.printStackTrace();
}
return result;
}
/**
* Set the base learner.
*
* @param newClassifier the classifier to use.
*/
@Override
public void setClassifier(Classifier newClassifier) {
if (!(newClassifier instanceof MultiTargetClassifier))
throw new IllegalStateException(
"Base classifier must implement " + MultiTargetClassifier.class.getName()
+ ", provided: " + newClassifier.getClass().getName());
super.setClassifier(newClassifier);
}
/**
* Returns the revision string.
*
* @return the revision
*/
@Override
public String getRevision() {
return RevisionUtils.extract("$Revision: 4521 $");
}
/**
* Main method for running this classifier from commandline.
*
* @param args the options
*/
public static void main(String[] args) {
ProblemTransformationMethod.evaluation(new MultiSearch(), args);
}
}
© 2015 - 2025 Weber Informatics LLC | Privacy Policy