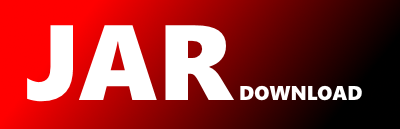
rbms.DBM Maven / Gradle / Ivy
Go to download
Show more of this group Show more artifacts with this name
Show all versions of meka Show documentation
Show all versions of meka Show documentation
The MEKA project provides an open source implementation of methods for multi-label classification and evaluation. It is based on the WEKA Machine Learning Toolkit. Several benchmark methods are also included, as well as the pruned sets and classifier chains methods, other methods from the scientific literature, and a wrapper to the MULAN framework.
The newest version!
/*
* This program is free software: you can redistribute it and/or modify
* it under the terms of the GNU General Public License as published by
* the Free Software Foundation, either version 3 of the License, or
* (at your option) any later version.
*
* This program is distributed in the hope that it will be useful,
* but WITHOUT ANY WARRANTY; without even the implied warranty of
* MERCHANTABILITY or FITNESS FOR A PARTICULAR PURPOSE. See the
* GNU General Public License for more details.
*
* You should have received a copy of the GNU General Public License
* along with this program. If not, see .
*/
package rbms;
import Jama.Matrix;
import java.util.*;
/**
* DBM - Stacked Restricted Boltzmann Machines.
*
* Like RBM, but with multiple layers (trained greedily).
* Default: N = 2 layers (both with H hidden units).
*
*
* ============== TRAINING =====================
* DBM dbm = new DBM();
* dbm.setOptions("-E 100 -H 10 -r 0.1 -m 0.8");
* ...
*
*
* @see RBM
* @author Jesse Read
* @version April 2013
*/
public class DBM extends RBM {
protected RBM rbm[] = null;
protected int h[] = null; // all layers
/**
* DBM - Create a DBM with 'options' (we use WEKA-style option processing).
*/
public DBM(String options[]) throws Exception {
super.setOptions(options);
}
public RBM[] getRBMs() {
return rbm;
}
@Override
public double[] prob_z(double z[]) {
if (rbm == null)
return null;
for(int i = 0; i < h.length; i++) {
z = rbm[i].prob_z(z); // input = rbm(input)
}
return z;
}
@Override
public double[][] prob_Z(double X_[][]) {
if (rbm == null)
return null;
for(int i = 0; i < h.length; i++) {
X_ = rbm[i].prob_Z(X_); // input = rbm(input)
}
return X_;
}
/*
// forward propagation
public double[][][] prop_Z_downs(double X_[][]) throws Exception {
double Z[][][] = new double[m_N+1][][];
Z[0] = X_;
for(int i = 0; i < m_N; i++) {
Z[i+1] = rbm[i].prop_Z_down(Z[i]); // input = rbm(input)
}
return Z;
}
*/
/** Set hidden layers specification */
public void setH(int h[]) {
this.h = h;
}
/**
* SetH - for a discriminative DBM (where the last h == L)
* @param H hidden layers
* @param L output linear layer
* @param N number of hidden layers
*/
public void setH(int H, int L, int N) {
int h[] = new int[N];
for(int i = 0; i < N-1; i++) {
h[i] = H;
}
h[N-1] = L;
this.h = h;
}
/**
* SetH -
* @param H hidden layers
* @param N number of hidden layers
*/
public void setH(int H, int N) {
int h[] = new int[N];
for(int i = 0; i < N; i++) {
h[i] = H;
}
this.h = h;
}
@Override
public void setH(int H) {
// default
setH(H,2);
}
/* not sure if will use this?
private int[] geth(int H, int N) {
int h[] = new int[N];
if (H > 0) {
// first layer is h
h[0] = H;
// each layer above is half as big
for(int i = 1; i < N; i++) {
h[i] = h[i-1] / 2;
}
}
else {
// output layer is -H
h[N-1] = Math.abs(H);
// each layer below is 4 times bigger
for(int i = N-2; i>=0; i--) {
h[i] = h[i+1] * 4;
}
}
return h;
}
*/
@Override
// should an RBM be a DBM of one layer?
public Matrix[] getWs() {
Matrix W[] = new Matrix[rbm.length];
for(int i = 0; i < W.length; i++) {
W[i] = this.rbm[i].getW();
}
return W;
}
@Override
public double train(double X_[][]) throws Exception {
return train(X_,0);
}
@Override
public double train(double X_[][], int batchSize) throws Exception {
int N = h.length;
this.rbm = new RBM[N];
// Greedily train RBMs, get Z off the top
for(int i = 0; i < N; i++) {
rbm[i] = new RBM(this.getOptions()); // same options as this instantiation
rbm[i].setH(h[i]); // but different number of hidden units
if (batchSize == 0)
rbm[i].train(X_);
else
rbm[i].train(X_,batchSize);
X_ = rbm[i].prob_Z(X_); // input = rbm(input)
}
return 1.0;
}
@Override
public void update(Matrix X) {
for(int i = 0; i < this.h.length; i++) {
rbm[i].update(X);
try {
X = rbm[i].prob_Z(X);
} catch(Exception e) {
System.err.println("AHH!!");
e.printStackTrace();
}
}
}
@Override
public void update(Matrix X, double s) {
for(int i = 0; i < this.h.length; i++) {
rbm[i].update(X,s);
try {
X = rbm[i].prob_Z(X);
} catch(Exception e) {
System.err.println("AHH!!");
e.printStackTrace();
}
}
}
@Override
public void update(double X_[][]) {
for(int i = 0; i < this.h.length; i++) {
rbm[i].update(X_);
try {
X_ = rbm[i].prob_Z(X_); // input = rbm(input)
} catch(Exception e) {
System.err.println("AHH!!");
e.printStackTrace();
}
}
}
}
© 2015 - 2025 Weber Informatics LLC | Privacy Policy