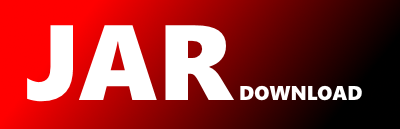
rbms.RBM Maven / Gradle / Ivy
Go to download
Show more of this group Show more artifacts with this name
Show all versions of meka Show documentation
Show all versions of meka Show documentation
The MEKA project provides an open source implementation of methods for multi-label classification and evaluation. It is based on the WEKA Machine Learning Toolkit. Several benchmark methods are also included, as well as the pruned sets and classifier chains methods, other methods from the scientific literature, and a wrapper to the MULAN framework.
The newest version!
/*
* This program is free software: you can redistribute it and/or modify
* it under the terms of the GNU General Public License as published by
* the Free Software Foundation, either version 3 of the License, or
* (at your option) any later version.
*
* This program is distributed in the hope that it will be useful,
* but WITHOUT ANY WARRANTY; without even the implied warranty of
* MERCHANTABILITY or FITNESS FOR A PARTICULAR PURPOSE. See the
* GNU General Public License for more details.
*
* You should have received a copy of the GNU General Public License
* along with this program. If not, see .
*/
package rbms;
import Jama.Matrix;
import meka.core.MatrixUtils;
import weka.core.*;
import java.util.*;
/**
* RBM.java - Restricted Boltzmann Machine.
* Using Contrastive Divergence.
*
* You have inputs X; You want to output to hidden units Z.
* To do this, you learn weight matrix W, where Z ~ sigma(W'X).
*
*
* ============== TRAINING (batches of 10) =====
* RBM rbm = new RBM();
* rbm.setOptions("-E 100 -H 10 -r 0.1 -m 0.8"); // to build 10 hidden units, over 100 epochs, with learning rate 0.1, momentum 0.8
* rbm.train(X,10); // train in batches of 10
* Z = rbm.getZ(X); // get output
* ============== UPDATING (one epoch) =========
* rbm.update(xnew);
* ============== TESTING (single input) =======
* z = rbm.getz(xnew);
*
* Note: should be binary for hidden states, can be probabilities for visible states.
*
* @author Jesse Read ([email protected])
* @version April 2013
*/
public class RBM {
protected double LEARNING_RATE = 0.1; // set between v small and 0.1 in [0,1]
protected double MOMENTUM = 0.1; // set between 0.1 and 0.9 in [0,1]
protected double COST = 0.0002 * LEARNING_RATE; // set between v small to ~ 0.001 * LEARNING_RATE The rate at which to degrade connection weights to penalize large weights
protected int m_E = 1000;
protected int m_H = 10;
private boolean m_V = false; // cut out of var(10) < 0.0001
private int batch_size = 0; // @todo implement this option (with CLI option)
protected Matrix W = null; // the weight matrix
protected Matrix dW_ = null; // used for momentum
protected Random m_R = new Random(0); // for random init. of matrices and sampling
/**
* RBM - Create an RBM with default options.
*/
public RBM() {
}
/**
* RBM - Create an RBM with 'options' (using WEKA-style option processing).
*/
public RBM(String options[]) throws Exception {
this.setOptions(options);
}
/**
* Set Options - WEKA-style option processing.
*/
public void setOptions(String[] options) throws Exception {
try {
setH(Integer.parseInt(Utils.getOption('H',options)));
setE(Integer.parseInt(Utils.getOption('E',options)));
setLearningRate(Double.parseDouble(Utils.getOption('r',options)));
setMomentum(Double.parseDouble(Utils.getOption('m',options)));
} catch(Exception e) {
System.err.println("Missing option!");
e.printStackTrace();
System.exit(1);
}
//super.setOptions(options);
}
/**
* GetOptions - WEKA-style option processing.
*/
public String[] getOptions() throws Exception {
ArrayList result;
result = new ArrayList(); //Arrays.asList(super.getOptions()));
result.add("-r");
result.add(String.valueOf(LEARNING_RATE));
result.add("-m");
result.add(String.valueOf(MOMENTUM));
result.add("-E");
result.add(String.valueOf(getE()));
result.add("-H");
result.add(String.valueOf(getH()));
return result.toArray(new String[result.size()]);
}
/**
* Hidden Activation Probability - returns P(z|x) where p(z[i]==1|x) for each element.
* A Bias is added (and removed) automatically.
* @param x_ x (without bias)
* @return z (without bias)
*/
public double[] prob_z(double x_[]) {
Matrix x = new Matrix(MatrixUtils.addBias(x_),1);
double z[] = MatrixUtils.sigma(x.times(W).getArray()[0]);
return MatrixUtils.removeBias(z);
}
/**
* Hidden Activation Probability - returns P(Z|X).
* A Bias column added (and removed) automatically.
* @param X_ X (without bias)
* @return P(Z|X)
*/
public double[][] prob_Z(double X_[][]) {
Matrix X = new Matrix(MatrixUtils.addBias(X_));
return MatrixUtils.removeBias(prob_Z(X).getArray());
}
/**
* Hidden Activation Probability - returns P(Z|X).
* A bias column is assumed to be included.
* @param X X (bias included)
* @return P(Z|X)
*/
public Matrix prob_Z(Matrix X) {
Matrix P_Z = MatrixUtils.sigma( X.times(W) ); // (this is the activation function)
MatrixUtils.fillCol(P_Z.getArray(), 0, 1.0); // fix bias ... set first col to 1.0
return P_Z;
}
/**
* Hidden Activation Value.
* A bias column added (and removed) automatically.
* @param X_ X (without bias)
* @return 1 if P(Z|X) greater than 0.5
*/
public double[][] propUp(double X_[][]) {
return MatrixUtils.threshold(prob_Z(X_),0.5); // ... or just go down
}
/**
* Sample Hidden Value - returns Z ~ P(Z|X).
* A bias column is assumed to be included.
* @param X X (bias included)
* @return Z ~ P(Z|X)
*/
public Matrix sample_Z(Matrix X) {
Matrix P_Z = prob_Z(X);
return MatrixUtils.sample(P_Z, m_R);
}
/**
* Sample Hidden Value - returns z[i] ~ p(z[i]==1|x) for each i-th element.
* A bias column added (and removed) automatically.
* @param x_ x (without bias)
* @return z ~ P(z|x) (without bias)
*/
public double[] sample_z(double x_[]) {
double p[] = prob_z(x_);
return MatrixUtils.sample(p, m_R);
}
/**
* Sample Visible - returns x[j] ~ p(x[j]==1|z) for each j-th element.
* A bias is added (and removed) automatically.
* @param z_ z (without bias)
* @return x ~ P(x|z) (without bias)
*/
public double[] sample_x(double z_[]) {
double p_x[] = prob_x(z_);
return MatrixUtils.sample(p_x, m_R);
}
/**
* Sample Visible - returns X ~ P(X|Z).
* A bias column is assumed to be included.
* @param Z Z (bias included)
* @return X ~ P(X|Z)
*/
public Matrix sample_X(Matrix Z) {
Matrix P_X = prob_X(Z);
return MatrixUtils.sample(P_X, m_R);
}
/**
* Visible Activation Probability - returns P(x|z) where p(x[j]==1|z) for each j-th element.
* A bias is added (and removed) automatically.
* @param z_ z (without bias)
* @return x (without bias)
*/
public double[] prob_x(double z_[]) {
Matrix z = new Matrix(MatrixUtils.addBias(z_),1);
double x[] = MatrixUtils.sigma(z.times(W.transpose()).getArray()[0]);
return MatrixUtils.removeBias(x);
}
/**
* Visible Activation Probability - returns P(X|Z).
* A bias column is assumed to be included.
* @param Z z (bias included)
* @return P(X|Z)
*/
public Matrix prob_X(Matrix Z) {
Matrix X = new Matrix(MatrixUtils.sigma(Z.times(W.transpose()).getArray())); // (this is the activation function)
MatrixUtils.fillCol(X.getArray(), 0, 1.0); // fix bias - set first col to 1.0
return X;
}
/**
* Make W matrix of dimensions d+1 and h+1 (+1 for biases).
* Initialized from ~N(0,0.2) (seems to work better than ~N(0.0.01)) -- except biases (set to 0)
* @param d number of rows (visible units)
* @param h number of columns (hidden units)
* @param r for getting random rumbers
* @return W
*/
public static Matrix makeW(int d, int h, Random r) {
double W_[][] = MatrixUtils.multiply(MatrixUtils.randn(d + 1, h + 1, r),0.20); // ~ N(0.0,0.01)
MatrixUtils.fillRow(W_, 0, 0.0); // set the first row to 0 for bias
MatrixUtils.fillCol(W_, 0, 0.0); // set the first col to 0 for bias
return new Matrix(W_);
}
protected Matrix makeW(int d, int h) {
return makeW(d,h,m_R);
}
/**
* Initialize W, and make _dW (for momentum) of the same dimensions.
* @param X_ X (only to know d = X_[0].length)
*/
private void initWeights(double X_[][]) {
initWeights(X_[0].length, m_H);
}
/**
* Initialize W, and make _dW (for momentum) of the same dimensions.
* @param d number of visible units
* @param h number of hidden units
*/
private void initWeights(int d, int h) {
this.W = makeW(d,h);
this.dW_ = new Matrix(W.getRowDimension(),W.getColumnDimension()); // for momentum
}
/**
* Initialize W, and make _dW (for momentum) of the same dimensions.
* @param d number of visible units
*/
public void initWeights(int d) {
initWeights(d,m_H);
}
/**
* Update - Carry out one epoch of CD, update W.
* We use dW_ to manage momentum.
*
* TODO weight decay SHOULD NOT BE APPLIED TO BIASES
* @param X X
*/
public void update(Matrix X) {
Matrix CD = epoch(X);
Matrix dW = (CD.minusEquals(this.W.times(COST))).timesEquals(LEARNING_RATE); // with COST
this.W.plusEquals(dW.plus(this.dW_.timesEquals(MOMENTUM))); // with MOMENTUM.
this.dW_ = dW; // for the next update
}
/**
* Update - Carry out one epoch of CD, update W.
*
* TODO combine with above fn.
* @param X X
* @param s multiply the gradient by this scalar
*/
public void update(Matrix X, double s) {
Matrix CD = epoch(X);
Matrix dW = (CD.minusEquals(this.W.times(COST))).timesEquals(LEARNING_RATE); // with COST
dW = dW.times(s); // *scaling factor
this.W.plusEquals(dW.plus(this.dW_.timesEquals(MOMENTUM))); // with MOMENTUM.
this.dW_ = dW; // for the next update
}
/**
* Update - On raw data (with no bias column)
* @param X_ raw double[][] data (with no bias column)
*/
public void update(double X_[][]) {
Matrix X = new Matrix(MatrixUtils.addBias(X_));
update(X);
}
/**
* Update - On raw data (with no bias column)
* @param x_ raw double[] data (with no bias column)
*/
public void update(double x_[]) {
update(new double[][]{x_});
}
/**
* Update - On raw data (with no bias column)
* @param x_ raw double[] data (with no bias column)
* @param s multiply the gradient by this scalar
*/
public void update(double x_[], double s) {
Matrix X = new Matrix(MatrixUtils.addBias(new double[][]{x_}));
update(X,s);
}
/**
* Train - Setup and train the RBM on X, over m_E epochs.
* @param X_ X
* @return the error (@TODO unnecessary)
*/
public double train(double X_[][]) throws Exception {
initWeights(X_);
Matrix X = new Matrix(MatrixUtils.addBias(X_));
double _error = Double.MAX_VALUE; // prev error , necessary only when using m_V
// TRAIN FOR m_E EPOCHS.
for(int e = 0; e < m_E; e++) {
// BREAK OUT IF THE GRADIENT IS POSITIVE
if (m_V) {
double err_now = calculateError(X); // Retrieve error
if (_error < err_now) {
System.out.println("broken out @"+e);
break;
}
_error = err_now;
}
/*
* The update
*/
update(X);
}
return _error;
}
/**
* Train - Setup and batch-train the RBM on X.
*
* TODO, above function train(X_) could really be trained with train(X_,N), so, should share code with train(X)
*
* TODO, divide gradient by the size of the batch! (doing already? .. no)
* @param X_ X
* @param batchSize the batch size
*/
public double train(double X_[][], int batchSize) throws Exception {
initWeights(X_);
X_ = MatrixUtils.addBias(X_);
int N = X_.length; // N
if (batchSize == N)
return train(X_);
int N_n = (int)Math.ceil(N*1./batchSize);// Number of batches
Matrix X_n[] = new Matrix[N_n];
for(int n = 0,i = 0; n < N; n+=batchSize,i++) {
// @TODO, could save some small-time memory/speed here
X_n[i] = new Matrix(Arrays.copyOfRange(X_,n,Math.min(n+batchSize,N)));
}
for(int e = 0; e < m_E; e++) {
// @TODO could be random, see function below
for(Matrix X : X_n) {
update(X, 1./N_n);
}
}
return 1.0;
}
/**
* Train - Setup and batch-train the RBM on X, with some random sampling involved.
*
* TODO should share code with train(X)
* @param X_ X
* @param batchSize the batch size
* @param r the randomness
*/
public double train(double X_[][], int batchSize, Random r) throws Exception {
initWeights(X_);
X_ = MatrixUtils.addBias(X_);
int N = X_.length; // N
int N_n = (int)Math.ceil(N*1./batchSize);// Number of batches
// @TODO select the batches randomly at each epoch
Matrix X_n[] = new Matrix[N_n];
for(int n = 0,i = 0; n < N; n+=batchSize,i++) {
X_n[i] = new Matrix(Arrays.copyOfRange(X_,n,Math.min(n+batchSize,N)));
}
for(int e = 0; e < m_E; e++) {
for(int i = 0; i < N_n; i++) {
update(X_n[r.nextInt(N_n)]);
}
}
return 1.0;
}
/**
* Calculate the Error right now.
*
* NOTE: this will take a few miliseconds longer than calculating directly in the epoch() loop (where we have to calculate X_down anyway).
*
* TODO rename this function
* @param X X
* @return The error
*/
public double calculateError(Matrix X) {
Matrix Z_up = prob_Z(X); // up @TODO replace with getZ(X,W), etc
Matrix X_down = prob_X(Z_up); // go down
// MSE
return MatrixUtils.meanSquaredError(X.getArray(), X_down.getArray()); // @note: this can take some milliseconds to calculate
}
/**
* Epoch - Run X through one epcho of CD of the RBM.
* @param X_0 The input matrix (includes bias column).
* @return the contrastive divergence (CD) for this epoch.
*
*
* x_0 = x
*
* for k = 0,...,K-1
* z_k = sample up
* x_k+1 = sample down
*
* e+ = pz|x_0
* e- = pz|x_K
*
* CD = e+ - e-
*
*
* Note: should be binary for hidden states, can be probabilities for visible states.
*/
public Matrix epoch(Matrix X_0) {
int N = X_0.getArray().length;
// POSITIVE
Matrix Z_0 = prob_Z(X_0); // sample up
Matrix E_pos = X_0.transpose().times(Z_0); // positive energy, H_1 * V_1
// NEGATIVE
Matrix X_1 = prob_X(Z_0); // go down -- can either sample down
//Matrix X_1 = Mat.threshold(prob_X(Z_0),0.5); // ... or just go down
Matrix pZ_1 = prob_Z(X_1); // go back up again
Matrix E_neg = X_1.transpose().times(pZ_1); // negative energy, P(Z_1) * X_1
// CALCULATE ERROR (Optional!)
//double _Err = Mat.meanSquaredError(X_0.getArray(),X_1.getArray()); // @note: this take some milliseconds to calculate
//System.out.println(""+_Err);
// CONTRASTIVE DIVERGENCE
Matrix CD = ((E_pos.minusEquals(E_neg)).times(1./N)); // CD = difference between energies
return CD;
}
// SAME AS ABOVE, BUT USES SAMPLING INSTEAD OF RAW PROBABILITIES. DOESN'T SEEM TO WORK AS WELL.
public Matrix sample_epoch(Matrix X_0) {
int N = X_0.getArray().length;
// POSITIVE
Matrix Z_0 = sample_Z(X_0); // sample up
Matrix E_pos = X_0.transpose().times(Z_0); // positive energy, H_1 * V_1
// NEGATIVE
Matrix X_1 = sample_X(Z_0); // go down -- can either sample down
//Matrix X_1 = Mat.threshold(prob_X(Z_0),0.5); // ... or just go down
Matrix pZ_1 = prob_Z(X_1); // go back up again
Matrix E_neg = X_1.transpose().times(pZ_1); // negative energy, P(Z_1) * X_1
// CALCULATE ERROR (Optional!)
double _Err = MatrixUtils.meanSquaredError(X_0.getArray(), X_1.getArray()); // @note: this take some milliseconds to calculate
System.out.println(""+_Err);
// CONTRASTIVE DIVERGENCE
Matrix CD = ((E_pos.minusEquals(E_neg)).times(1./N)); // CD = difference between energies
return CD;
}
/* *********************************************************************************
* Get / Set Parameters
**********************************************************************************/
public void setH(int h) {
m_H = h;
}
public int getH() {
return m_H;
}
/**
* SetE - set the number of epochs (if n is negative, it means max epochs).
*/
public void setE(int n) {
if (n < 0) {
m_V = true;
m_E = -n;
}
else {
m_E = n;
}
}
public int getE() {
return m_E;
}
public void setLearningRate(double r) {
LEARNING_RATE = r;
COST = 0.0002 * LEARNING_RATE;
}
public double getLearningRate() {
return LEARNING_RATE;
}
public void setMomentum(double m) {
MOMENTUM = m;
}
public double getMomentum() {
return MOMENTUM;
}
public void setSeed(int seed) {
m_R = new Random(seed);
}
/* *********************************************************************************
* Get Weight Matrix(es)
**********************************************************************************/
public Matrix[] getWs() {
return new Matrix[]{W};
}
public Matrix getW() {
return W;
}
/**
* ToString - return a String representation of the weight Matrix defining this RBM.
*/
public String toString() {
Matrix W = getW();
return MatrixUtils.toString(W);
}
/**
* Main - do some test routines.
*/
public static void main(String argv[]) throws Exception {
}
}
© 2015 - 2025 Weber Informatics LLC | Privacy Policy