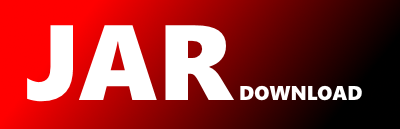
cern.colt.matrix.doc-files.allColt1.0.1ibm1.3LxPIII.txt Maven / Gradle / Ivy
Go to download
Show more of this group Show more artifacts with this name
Show all versions of parallelcolt Show documentation
Show all versions of parallelcolt Show documentation
Parallel Colt is a multithreaded version of Colt - a library for high performance scientific computing in Java. It contains efficient algorithms for data analysis, linear algebra, multi-dimensional arrays, Fourier transforms, statistics and histogramming.
The newest version!
Colt Matrix benchmark running on
java.vm.vendor IBM Corporation
java.vm.version 1.3.0
java.vm.name Classic VM
os.name Linux
os.version 2.2.12-20smp
os.arch x86
java.version 1.3.0
java.vendor IBM Corporation
java.vendor.url http://www.ibm.com/
Colt Version is Version 1.0.1.51 (Mon May 15 20:23:08 CEST 2000)
Please report problems to [email protected] or http://nicewww.cern.ch/~hoschek/colt/index.htm
Executing command = [dgemm, dense, 2, 2, 0.999, false, true, 5, 10, 25, 50, 100, 250, 500, 1000] ...
@x.x.x.x.x.x.x.x.*
Performance of Blas matrix-matrix mult dgemm(false, true, 1, A, B, 0, C)
type=dense
| size
| 5 10 25 50 100 250 500 1000
-----------------------------------------------------------------------
d 0.999 | 36.528 82.766 112.261 157.978 198.849 212.999 204.165 175.932
Run took a total of Time=37.602 secs. End of run.
Executing command = [dgemm, dense, 1, 2, 0.999, false, true, 5, 10, 25, 50, 100, 250, 500, 1000] ...
@x.x.x.x.x.x.x.x.*
Performance of Blas matrix-matrix mult dgemm(false, true, 1, A, B, 0, C)
type=dense
| size
| 5 10 25 50 100 250 500 1000
----------------------------------------------------------------------
d 0.999 | 36.213 74.518 102.758 105.095 119.012 116.333 102.124 87.409
Run took a total of Time=49.605 secs. End of run.
Executing command = [dgemm, rowCompressed, 1, 2, 0.01, false, false, 5, 10, 25, 50, 100, 250, 500, 1000] ...
@x.x.x.x.x.x.x.x.*
Performance of Blas matrix-matrix mult dgemm(false, false, 1, A, B, 0, C)
type=rowCompressed
| size
| 5 10 25 50 100 250 500 1000
-------------------------------------------------------------------------------------
d 0.01 | 32.525 138.719 703.833 1.845E+003 3.27E+003 2.848E+003 2.772E+003 2.951E+003
Run took a total of Time=32.202 secs. End of run.
Executing command = [dgemm, sparse, 1, 2, 0.01, false, false, 5, 10, 25, 50, 100, 250, 500, 1000] ...
@x.x.x.x.x.x.x.x.*
Performance of Blas matrix-matrix mult dgemm(false, false, 1, A, B, 0, C)
type=sparse
| size
| 5 10 25 50 100 250 500 1000
-------------------------------------------------------------------------------------
d 0.01 | 31.128 135.907 637.538 1.767E+003 3.15E+003 2.751E+003 2.817E+003 2.883E+003
Run took a total of Time=32.277 secs. End of run.
Executing command = [dgemv, dense, 2, 2, 0.01, false, 5, 10, 25, 50, 100, 250, 500, 1000, 2000] ...
@x.x.x.x.x.x.x.x.x.*
Performance of Blas matrix-vector mult dgemv(false, 1, A, B, 0, C)
type=dense
| size
| 5 10 25 50 100 250 500 1000 2000
---------------------------------------------------------------------------
d 0.01 | 20.828 52.811 103.611 104.354 109.498 101.451 67.187 66.341 54.554
Run took a total of Time=39.415 secs. End of run.
Executing command = [dgemv, dense, 1, 2, 0.01, false, 5, 10, 25, 50, 100, 250, 500, 1000, 2000] ...
@x.x.x.x.x.x.x.x.x.*
Performance of Blas matrix-vector mult dgemv(false, 1, A, B, 0, C)
type=dense
| size
| 5 10 25 50 100 250 500 1000 2000
-------------------------------------------------------------------------
d 0.01 | 30.574 65.79 114.877 101.596 111.633 47.494 31.702 31.778 31.793
Run took a total of Time=40.283 secs. End of run.
Executing command = [dgemv, rowCompressed, 1, 2, 0.01, false, 5, 10, 25, 50, 100, 250, 500, 1000, 2000] ...
@x.x.x.x.x.x.x.x.x.*
Performance of Blas matrix-vector mult dgemv(false, 1, A, B, 0, C)
type=rowCompressed
| size
| 5 10 25 50 100 250 500 1000 2000
------------------------------------------------------------------------------------------------
d 0.01 | 31.796 145.363 527.966 1.143E+003 2.33E+003 3.737E+003 4.776E+003 5.992E+003 3.448E+003
Run took a total of Time=41.138 secs. End of run.
Executing command = [dgemv, sparse, 1, 2, 0.01, false, 5, 10, 25, 50, 100, 250, 500, 1000, 2000] ...
@x.x.x.x.x.x.x.x.x.*
Performance of Blas matrix-vector mult dgemv(false, 1, A, B, 0, C)
type=sparse
| size
| 5 10 25 50 100 250 500 1000 2000
------------------------------------------------------------------------------
d 0.01 | 23.507 84.216 341.435 598.555 717.508 733.817 768.899 756.811 573.721
Run took a total of Time=38.784 secs. End of run.
Executing command = [assign, dense, 1, 2, 0.999, 5, 10, 25, 50, 100, 250, 500, 1000] ...
@x.x.x.x.x.x.x.x.*
Performance of A.assign(B) [Mops/sec]
type=dense
| size
| 5 10 25 50 100 250 500 1000
-----------------------------------------------------------------
d 0.999 | 79.408 164.319 309.899 35.58 44.551 20.849 21.326 21.78
Run took a total of Time=36.992 secs. End of run.
Executing command = [assignGetSet, dense, 1, 2, 0.999, 5, 10, 25, 50, 100, 250, 500, 1000] ...
@x.x.x.x.x.x.x.x.*
Performance of A.assign(B) via get and set [Mops/sec]
type=dense
| size
| 5 10 25 50 100 250 500 1000
--------------------------------------------------------
d 0.999 | 8.567 7.898 8.028 7.567 7.823 5.57 5.289 5.275
Run took a total of Time=32.556 secs. End of run.
Executing command = [assignGetSetQuick, dense, 1, 2, 0.999, 5, 10, 25, 50, 100, 250, 500, 1000] ...
@x.x.x.x.x.x.x.x.*
Performance of A.assign(B) via getQuick and setQuick [Mops/sec]
type=dense
| size
| 5 10 25 50 100 250 500 1000
------------------------------------------------------------
d 0.999 | 11.381 11.172 11.51 10.19 10.943 7.344 7.516 7.495
Run took a total of Time=32.857 secs. End of run.
Executing command = [elementwiseMult, dense, 1, 2, 0.999, 5, 10, 25, 50, 100, 250, 500, 1000] ...
@x.x.x.x.x.x.x.x.*
Performance of A.assign(F.mult(0.5)) via Blas [Mflops/sec]
type=dense
| size
| 5 10 25 50 100 250 500 1000
----------------------------------------------------------------
d 0.999 | 34.886 64.726 94.636 43.634 52.731 25.993 22.014 22.75
Run took a total of Time=37.829 secs. End of run.
Executing command = [elementwiseMultB, dense, 1, 2, 0.999, 5, 10, 25, 50, 100, 250, 500, 1000] ...
@x.x.x.x.x.x.x.x.*
Performance of A.assign(B,F.mult) via Blas [Mflops/sec]
type=dense
| size
| 5 10 25 50 100 250 500 1000
-----------------------------------------------------------------
d 0.999 | 44.274 55.451 60.475 35.507 26.752 14.646 14.925 14.599
Run took a total of Time=34.514 secs. End of run.
Executing command = [assignLog, dense, 1, 2, 0.999, 5, 10, 25, 50, 100, 250, 500, 1000] ...
@x.x.x.x.x.x.x.x.*
Performance of A[i,j] = log(A[i,j]) via Blas.assign(fun) [Mflops/sec]
type=dense
| size
| 5 10 25 50 100 250 500 1000
---------------------------------------------------------
d 0.999 | 4.697 4.703 4.777 4.694 4.804 4.333 4.171 4.204
Run took a total of Time=33.089 secs. End of run.
Executing command = [assignLog, dense, 2, 2, 0.999, 5, 10, 25, 50, 100, 250, 500, 1000] ...
@x.x.x.x.x.x.x.x.*
Performance of A[i,j] = log(A[i,j]) via Blas.assign(fun) [Mflops/sec]
type=dense
| size
| 5 10 25 50 100 250 500 1000
---------------------------------------------------------
d 0.999 | 4.351 4.727 4.881 4.775 4.894 7.295 6.991 7.246
Run took a total of Time=32.783 secs. End of run.
Executing command = [assignPlusMult, dense, 1, 2, 0.999, 5, 10, 25, 50, 100, 250, 500, 1000] ...
@x.x.x.x.x.x.x.x.*
Performance of A[i,j] = A[i,j] + s*B[i,j] via Blas.assign(fun) [Mflops/sec]
type=dense
| size
| 5 10 25 50 100 250 500 1000
-----------------------------------------------------------------
d 0.999 | 52.745 79.516 94.163 74.865 44.036 31.974 29.661 31.826
Run took a total of Time=34.536 secs. End of run.
Executing command = [SOR5, dense, 1, 2, 0.999, 5, 10, 25, 50, 100, 250, 500, 1000] ...
@x.x.x.x.x.x.x.x.*
Performance of A.zAssign8Neighbors(5 point function) [Mflops/sec]
type=dense
| size
| 5 10 25 50 100 250 500 1000
---------------------------------------------------------------
d 0.999 | 117.043 74.677 59.492 55.529 53.61 38.48 39.857 37.43
Run took a total of Time=32.801 secs. End of run.
Executing command = [SOR8, dense, 1, 2, 0.999, 5, 10, 25, 50, 100, 250, 500, 1000] ...
@x.x.x.x.x.x.x.x.*
Performance of A.zAssign8Neighbors(9 point function) [Mflops/sec]
type=dense
| size
| 5 10 25 50 100 250 500 1000
------------------------------------------------------------------
d 0.999 | 128.176 82.446 64.465 55.579 55.606 42.713 43.439 42.849
Run took a total of Time=32.433 secs. End of run.
Executing command = [LUDecompose, dense, 1, 2, 0.999, 5, 10, 25, 50, 100, 250, 500, 1000] ...
@x.x.x.x.x.x.x.x.*
Performance of LU.decompose(A) [Mflops/sec]
type=dense
| size
| 5 10 25 50 100 250 500 1000
----------------------------------------------------------------
d 0.999 | 3.482 10.428 27.323 51.485 67.178 88.586 35.401 30.844
Run took a total of Time=47.515 secs. End of run.
Executing command = [LUSolve, dense, 1, 2, 0.999, 5, 10, 25, 50, 100, 250, 500, 1000] ...
@x.x.x.x.x.x.x.x.*
Performance of LU.solve(A) [Mflops/sec]
type=dense
| size
| 5 10 25 50 100 250 500 1000
----------------------------------------------------------------
d 0.999 | 12.488 30.537 66.43 78.551 73.841 56.787 26.843 25.915
Run took a total of Time=134.412 secs. End of run.
Executing command = [pow, dense, 1, 2, 0.999, -1, 5, 10, 25, 50, 100, 250, 500, 1000] ...
@x.x.x.x.x.x.x.x.*
Performance of matrix to the power of an exponent pow(A,-1)
type=dense
| size
| 5 10 25 50 100 250 500 1000
----------------------------------------------------------------
d 0.999 | 5.407 15.041 41.556 60.984 70.523 63.082 30.962 28.746
Run took a total of Time=127.618 secs. End of run.
Command file name used: /afs/cern.ch/user/h/hoschek/coltb4/cmd2
To reproduce and compare results, here it's contents:
// matrix-matrix mult with 1 and with 2 CPUs
dgemm dense 2 2.0 0.999 false true 5 10 25 50 100 250 500 1000
dgemm dense 1 2.0 0.999 false true 5 10 25 50 100 250 500 1000
dgemm rowCompressed 1 2.0 0.01 false false 5 10 25 50 100 250 500 1000
dgemm sparse 1 2.0 0.01 false false 5 10 25 50 100 250 500 1000
// matrix-vector mult
dgemv dense 2 2.0 0.01 false 5 10 25 50 100 250 500 1000 2000
dgemv dense 1 2.0 0.01 false 5 10 25 50 100 250 500 1000 2000
dgemv rowCompressed 1 2.0 0.01 false 5 10 25 50 100 250 500 1000 2000
dgemv sparse 1 2.0 0.01 false 5 10 25 50 100 250 500 1000 2000
assign dense 1 2.0 0.999 5 10 25 50 100 250 500 1000
assignGetSet dense 1 2.0 0.999 5 10 25 50 100 250 500 1000
assignGetSetQuick dense 1 2.0 0.999 5 10 25 50 100 250 500 1000
// with 1 and with 2 CPUs
elementwiseMult dense 1 2.0 0.999 5 10 25 50 100 250 500 1000
elementwiseMultB dense 1 2.0 0.999 5 10 25 50 100 250 500 1000
assignLog dense 1 2.0 0.999 5 10 25 50 100 250 500 1000
assignLog dense 2 2.0 0.999 5 10 25 50 100 250 500 1000
assignPlusMult dense 1 2.0 0.999 5 10 25 50 100 250 500 1000
assignPlusMult dense 2 2.0 0.999 5 10 25 50 100 250 500 1000
SOR5 dense 1 2.0 0.999 5 10 25 50 100 250 500 1000
SOR8 dense 1 2.0 0.999 5 10 25 50 100 250 500 1000
LUDecompose dense 1 2.0 0.999 5 10 25 50 100 250 500 1000
LUSolve dense 1 2.0 0.999 5 10 25 50 100 250 500 1000
pow dense 1 2.0 0.999 -1 5 10 25 50 100 250 500 1000
© 2015 - 2025 Weber Informatics LLC | Privacy Policy