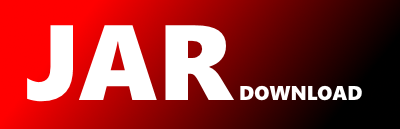
cern.colt.matrix.doc-files.out6 Maven / Gradle / Ivy
Go to download
Show more of this group Show more artifacts with this name
Show all versions of parallelcolt Show documentation
Show all versions of parallelcolt Show documentation
Parallel Colt is a multithreaded version of Colt - a library for high performance scientific computing in Java. It contains efficient algorithms for data analysis, linear algebra, multi-dimensional arrays, Fourier transforms, statistics and histogramming.
The newest version!
Colt Matrix benchmark running on
java.vm.vendor IBM Corporation
java.vm.version 1.3.0
java.vm.name Classic VM
os.name Linux
os.version 2.2.12-20smp
os.arch x86
java.version 1.3.0
java.vendor IBM Corporation
java.vendor.url http://www.ibm.com/
Colt Version is Version 1.0.1.51 (Mon May 15 20:23:08 CEST 2000)
Please report problems to [email protected] or http://nicewww.cern.ch/~hoschek/colt/index.htm
Executing command = [dgemm, dense, 2, 2, 0.999, false, true, 5, 10, 25, 50, 100, 250, 500, 1000] ...
@x.x.x.x.x.x.x.x.*
Performance of Blas matrix-matrix mult dgemm(false, true, 1, A, B, 0, C)
type=dense
| size
| 5 10 25 50 100 250 500 1000
-----------------------------------------------------------------------
d 0.999 | 36.528 82.766 112.261 157.978 198.849 212.999 204.165 175.932
Run took a total of Time=37.602 secs. End of run.
Executing command = [dgemm, dense, 1, 2, 0.999, false, true, 5, 10, 25, 50, 100, 250, 500, 1000] ...
@x.x.x.x.x.x.x.x.*
Performance of Blas matrix-matrix mult dgemm(false, true, 1, A, B, 0, C)
type=dense
| size
| 5 10 25 50 100 250 500 1000
----------------------------------------------------------------------
d 0.999 | 36.213 74.518 102.758 105.095 119.012 116.333 102.124 87.409
Run took a total of Time=49.605 secs. End of run.
Executing command = [dgemm, rowCompressed, 1, 2, 0.01, false, false, 5, 10, 25, 50, 100, 250, 500, 1000] ...
@x.x.x.x.x.x.x.x.*
Performance of Blas matrix-matrix mult dgemm(false, false, 1, A, B, 0, C)
type=rowCompressed
| size
| 5 10 25 50 100 250 500 1000
-------------------------------------------------------------------------------------
d 0.01 | 32.525 138.719 703.833 1.845E+003 3.27E+003 2.848E+003 2.772E+003 2.951E+003
Run took a total of Time=32.202 secs. End of run.
Executing command = [dgemm, sparse, 1, 2, 0.01, false, false, 5, 10, 25, 50, 100, 250, 500, 1000] ...
@x.x.x.x.x.x.x.x.*
Performance of Blas matrix-matrix mult dgemm(false, false, 1, A, B, 0, C)
type=sparse
| size
| 5 10 25 50 100 250 500 1000
-------------------------------------------------------------------------------------
d 0.01 | 31.128 135.907 637.538 1.767E+003 3.15E+003 2.751E+003 2.817E+003 2.883E+003
Run took a total of Time=32.277 secs. End of run.
Executing command = [dgemv, dense, 2, 2, 0.01, false, 5, 10, 25, 50, 100, 250, 500, 1000, 2000] ...
@x.x.x.x.x.x.x.x.x.*
Performance of Blas matrix-vector mult dgemv(false, 1, A, B, 0, C)
type=dense
| size
| 5 10 25 50 100 250 500 1000 2000
---------------------------------------------------------------------------
d 0.01 | 20.828 52.811 103.611 104.354 109.498 101.451 67.187 66.341 54.554
Run took a total of Time=39.415 secs. End of run.
Executing command = [dgemv, dense, 1, 2, 0.01, false, 5, 10, 25, 50, 100, 250, 500, 1000, 2000] ...
@x.x.x.x.x.x.x.x.x.*
Performance of Blas matrix-vector mult dgemv(false, 1, A, B, 0, C)
type=dense
| size
| 5 10 25 50 100 250 500 1000 2000
-------------------------------------------------------------------------
d 0.01 | 30.574 65.79 114.877 101.596 111.633 47.494 31.702 31.778 31.793
Run took a total of Time=40.283 secs. End of run.
Executing command = [dgemv, rowCompressed, 1, 2, 0.01, false, 5, 10, 25, 50, 100, 250, 500, 1000, 2000] ...
@x.x.x.x.x.x.x.x.x.*
Performance of Blas matrix-vector mult dgemv(false, 1, A, B, 0, C)
type=rowCompressed
| size
| 5 10 25 50 100 250 500 1000 2000
------------------------------------------------------------------------------------------------
d 0.01 | 31.796 145.363 527.966 1.143E+003 2.33E+003 3.737E+003 4.776E+003 5.992E+003 3.448E+003
Run took a total of Time=41.138 secs. End of run.
Executing command = [dgemv, sparse, 1, 2, 0.01, false, 5, 10, 25, 50, 100, 250, 500, 1000, 2000] ...
@x.x.x.x.x.x.x.x.x.*
Performance of Blas matrix-vector mult dgemv(false, 1, A, B, 0, C)
type=sparse
| size
| 5 10 25 50 100 250 500 1000 2000
------------------------------------------------------------------------------
d 0.01 | 23.507 84.216 341.435 598.555 717.508 733.817 768.899 756.811 573.721
Run took a total of Time=38.784 secs. End of run.
Executing command = [assign, dense, 1, 2, 0.999, 5, 10, 25, 50, 100, 250, 500, 1000] ...
@x.x.x.x.x.x.x.x.*
Performance of A.assign(B) [Mops/sec]
type=dense
| size
| 5 10 25 50 100 250 500 1000
-----------------------------------------------------------------
d 0.999 | 79.408 164.319 309.899 35.58 44.551 20.849 21.326 21.78
Run took a total of Time=36.992 secs. End of run.
Executing command = [assignGetSet, dense, 1, 2, 0.999, 5, 10, 25, 50, 100, 250, 500, 1000] ...
@x.x.x.x.x.x.x.x.*
Performance of A.assign(B) via get and set [Mops/sec]
type=dense
| size
| 5 10 25 50 100 250 500 1000
--------------------------------------------------------
d 0.999 | 8.567 7.898 8.028 7.567 7.823 5.57 5.289 5.275
Run took a total of Time=32.556 secs. End of run.
Executing command = [assignGetSetQuick, dense, 1, 2, 0.999, 5, 10, 25, 50, 100, 250, 500, 1000] ...
@x.x.x.x.x.x.x.x.*
Performance of A.assign(B) via getQuick and setQuick [Mops/sec]
type=dense
| size
| 5 10 25 50 100 250 500 1000
------------------------------------------------------------
d 0.999 | 11.381 11.172 11.51 10.19 10.943 7.344 7.516 7.495
Run took a total of Time=32.857 secs. End of run.
Executing command = [elementwiseMult, dense, 1, 2, 0.999, 5, 10, 25, 50, 100, 250, 500, 1000] ...
@x.x.x.x.x.x.x.x.*
Performance of A.assign(F.mult(0.5)) via Blas [Mflops/sec]
type=dense
| size
| 5 10 25 50 100 250 500 1000
----------------------------------------------------------------
d 0.999 | 34.886 64.726 94.636 43.634 52.731 25.993 22.014 22.75
Run took a total of Time=37.829 secs. End of run.
Executing command = [elementwiseMult, dense, 2, 2, 0.999, 5, 10, 25, 50, 100, 250, 500, 1000] ...
@x.x.x.x.x.x.x.
© 2015 - 2025 Weber Informatics LLC | Privacy Policy