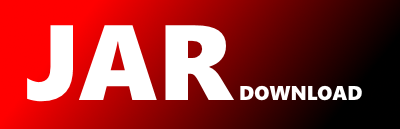
cern.colt.matrix.tdouble.algo.decomposition.SparseDoubleKLUDecomposition Maven / Gradle / Ivy
Go to download
Show more of this group Show more artifacts with this name
Show all versions of parallelcolt Show documentation
Show all versions of parallelcolt Show documentation
Parallel Colt is a multithreaded version of Colt - a library for high performance scientific computing in Java. It contains efficient algorithms for data analysis, linear algebra, multi-dimensional arrays, Fourier transforms, statistics and histogramming.
The newest version!
package cern.colt.matrix.tdouble.algo.decomposition;
import cern.colt.matrix.tdouble.DoubleMatrix1D;
import cern.colt.matrix.tdouble.DoubleMatrix2D;
import cern.colt.matrix.tdouble.algo.DoubleProperty;
import cern.colt.matrix.tdouble.impl.SparseCCDoubleMatrix2D;
import cern.colt.matrix.tdouble.impl.SparseRCDoubleMatrix2D;
import edu.emory.mathcs.csparsej.tdouble.Dcs_common.Dcs;
import edu.ufl.cise.klu.common.KLU_common;
import edu.ufl.cise.klu.common.KLU_numeric;
import edu.ufl.cise.klu.common.KLU_symbolic;
import static edu.ufl.cise.btf.tdouble.Dbtf_maxtrans.btf_maxtrans;
import static edu.ufl.cise.klu.tdouble.Dklu_analyze.klu_analyze;
import static edu.ufl.cise.klu.tdouble.Dklu_defaults.klu_defaults;
import static edu.ufl.cise.klu.tdouble.Dklu_factor.klu_factor;
import static edu.ufl.cise.klu.tdouble.Dklu_solve.klu_solve;
import static edu.ufl.cise.klu.tdouble.Dklu_extract.klu_extract;
/**
* LU decomposition implemented using JKLU.
*
* @author Piotr Wendykier ([email protected])
* @author Richard Lincoln ([email protected])
*/
public class SparseDoubleKLUDecomposition implements SparseDoubleLUDecomposition {
private KLU_symbolic S;
private KLU_numeric N;
private KLU_common Common;
private DoubleMatrix2D L;
private DoubleMatrix2D U;
private boolean rcMatrix = false;
private boolean isNonSingular = true;
/**
* Row and column dimension (square matrix).
*/
private int n;
/**
* Constructs and returns a new LU Decomposition object; The decomposed
* matrices can be retrieved via instance methods of the returned
* decomposition object.
*
* @param A
* Square matrix
* @param order
* ordering option (0 to 1); 0: AMD, 1: COLAMD
* @param checkIfSingular
* if true, then the singularity test (based on
* BTFJ) is performed.
* @param preOrder use BTF pre-ordering, or not
* @throws IllegalArgumentException
* if A is not square or is not sparse.
* @throws IllegalArgumentException
* if order is not in [0,1]
*/
public SparseDoubleKLUDecomposition(DoubleMatrix2D A, int order, boolean checkIfSingular, boolean preOrder) {
DoubleProperty.DEFAULT.checkSquare(A);
DoubleProperty.DEFAULT.checkSparse(A);
if (order < 0 || order > 3) {
throw new IllegalArgumentException("order must be a number between 0 and 3");
}
Common = new KLU_common();
klu_defaults(Common);
Common.ordering = order;
Common.btf = preOrder ? 1 : 0;
Dcs dcs;
if (A instanceof SparseRCDoubleMatrix2D) {
rcMatrix = true;
dcs = ((SparseRCDoubleMatrix2D) A).getColumnCompressed().elements();
} else {
dcs = (Dcs) A.elements();
}
n = A.rows();
int[] Ap = dcs.p, Ai = dcs.i;
double[] Ax = dcs.x;
S = klu_analyze(n, Ap, Ai, Common);
if (S == null) {
throw new IllegalArgumentException("Exception occured in klu_analyze()");
}
N = klu_factor(Ap, Ai, Ax, S, Common);
if (N == null) {
throw new IllegalArgumentException("Exception occured in klu_factor()");
}
if (checkIfSingular) {
/* check if matrix is singular */
int sprank = btf_maxtrans(n, n, Ap, Ai, Common.maxwork, new double[1], new int[n]) ;
if (sprank < n) {
isNonSingular = false;
}
}
}
public SparseDoubleKLUDecomposition(DoubleMatrix2D A, int order, boolean checkIfSingular) {
this(A, order, checkIfSingular, true);
}
/* (non-Javadoc)
* @see cern.colt.matrix.tdouble.algo.decomposition.SparseDoubleLUDecomposition#det()
*/
public double det() {
if (!isNonsingular())
return 0; // avoid rounding errors
int pivsign = 1;
for (int i = 0; i < n; i++) {
if (N.Pinv[i] != i) {
pivsign = -pivsign;
}
}
if (U == null) {
U = getU();
}
double det = pivsign;
for (int j = 0; j < n; j++) {
det *= U.getQuick(j, j);
}
return det;
}
/* (non-Javadoc)
* @see cern.colt.matrix.tdouble.algo.decomposition.SparseDoubleLUDecomposition#getL()
*/
public DoubleMatrix2D getL() {
if (L == null) {
int[] Lp = new int[N.lnz + 1];
int[] Li = new int[N.lnz];
double[] Lx = new double[N.lnz];
klu_extract(N, S, Lp, Li, Lx, null, null, null, null, null, null, null, null, null, null, Common);
L = new SparseCCDoubleMatrix2D(n, n, Li, Lp, Lx);
if (rcMatrix) {
L = ((SparseCCDoubleMatrix2D) L).getRowCompressed();
}
}
return L.copy();
}
/* (non-Javadoc)
* @see cern.colt.matrix.tdouble.algo.decomposition.SparseDoubleLUDecomposition#getPivot()
*/
public int[] getPivot() {
if (N.Pinv == null)
return null;
int[] pinv = new int[N.Pinv.length];
System.arraycopy(N.Pinv, 0, pinv, 0, pinv.length);
return pinv;
}
/* (non-Javadoc)
* @see cern.colt.matrix.tdouble.algo.decomposition.SparseDoubleLUDecomposition#getU()
*/
public DoubleMatrix2D getU() {
if (U == null) {
int[] Up = new int[N.unz + 1];
int[] Ui = new int[N.unz];
double[] Ux = new double[N.unz];
klu_extract(N, S, null, null, null, Up, Ui, Ux, null, null, null, null, null, null, null, Common);
U = new SparseCCDoubleMatrix2D(n, n, Ui, Up, Ux);
if (rcMatrix) {
U = ((SparseCCDoubleMatrix2D) U).getRowCompressed();
}
}
return U.copy();
}
/* (non-Javadoc)
* @see cern.colt.matrix.tdouble.algo.decomposition.SparseDoubleLUDecomposition#getSymbolicAnalysis()
*/
public KLU_symbolic getSymbolicAnalysis() {
KLU_symbolic S2 = new KLU_symbolic();
S2.symmetry = S.symmetry;
S2.est_flops = S.est_flops;
S2.lnz = S.lnz;
S2.unz = S.unz;
S2.Lnz = S.Lnz.clone();
S2.n = S.n;
S2.nz = S.nz;
S2.nzoff = S.nzoff;
S2.nblocks = S.nblocks;
S2.maxblock = S.maxblock;
S2.ordering = S.ordering;
S2.do_btf = S.do_btf;
S2.P = S.P.clone();
S2.Q = S.Q.clone();
S2.R = S.R.clone();
S2.structural_rank = S.structural_rank;
return S2;
}
/* (non-Javadoc)
* @see cern.colt.matrix.tdouble.algo.decomposition.SparseDoubleLUDecomposition#isNonsingular()
*/
public boolean isNonsingular() {
return isNonSingular;
}
/* (non-Javadoc)
* @see cern.colt.matrix.tdouble.algo.decomposition.SparseDoubleLUDecomposition#solve(cern.colt.matrix.tdouble.DoubleMatrix1D)
*/
public void solve(DoubleMatrix1D b) {
if (b.size() != n) {
throw new IllegalArgumentException("b.size() != A.rows()");
}
if (!isNonsingular()) {
throw new IllegalArgumentException("A is singular");
}
DoubleProperty.DEFAULT.checkDense(b);
double[] x;
if (b.isView()) {
x = (double[]) b.copy().elements();
} else {
x = (double[]) b.elements();
}
klu_solve(S, N, n, 1, x, 0, Common);
if (b.isView()) {
b.assign(x);
}
}
}
© 2015 - 2025 Weber Informatics LLC | Privacy Policy