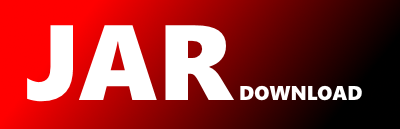
cern.colt.matrix.tdouble.algo.solver.DoubleHyBR Maven / Gradle / Ivy
Go to download
Show more of this group Show more artifacts with this name
Show all versions of parallelcolt Show documentation
Show all versions of parallelcolt Show documentation
Parallel Colt is a multithreaded version of Colt - a library for high performance scientific computing in Java. It contains efficient algorithms for data analysis, linear algebra, multi-dimensional arrays, Fourier transforms, statistics and histogramming.
The newest version!
/*
* Copyright (C) 2009 Piotr Wendykier
*
* This program is free software; you can redistribute it and/or modify it
* under the terms of the GNU Lesser General Public License as published by the
* Free Software Foundation; either version 2.1 of the License, or (at your
* option) any later version.
*
* This library is distributed in the hope that it will be useful, but WITHOUT
* ANY WARRANTY; without even the implied warranty of MERCHANTABILITY or
* FITNESS FOR A PARTICULAR PURPOSE. See the GNU Lesser General Public License
* for more details.
*
* You should have received a copy of the GNU Lesser General Public License
* along with this library; if not, write to the Free Software Foundation,
* Inc., 59 Temple Place, Suite 330, Boston, MA 02111-1307 USA
*/
package cern.colt.matrix.tdouble.algo.solver;
import optimization.DoubleFmin;
import optimization.DoubleFmin_methods;
import cern.colt.list.tdouble.DoubleArrayList;
import cern.colt.matrix.tdouble.DoubleFactory2D;
import cern.colt.matrix.tdouble.DoubleMatrix1D;
import cern.colt.matrix.tdouble.DoubleMatrix2D;
import cern.colt.matrix.tdouble.algo.DenseDoubleAlgebra;
import cern.colt.matrix.tdouble.algo.decomposition.DenseDoubleSingularValueDecomposition;
import cern.colt.matrix.tdouble.algo.solver.preconditioner.DoubleIdentity;
import cern.colt.matrix.tdouble.algo.solver.preconditioner.DoublePreconditioner;
import cern.colt.matrix.tdouble.impl.DenseColumnDoubleMatrix2D;
import cern.colt.matrix.tdouble.impl.DenseDoubleMatrix1D;
import cern.colt.matrix.tdouble.impl.DenseDoubleMatrix2D;
import cern.jet.math.tdouble.DoubleFunctions;
import cern.jet.stat.tdouble.DoubleDescriptive;
/**
* HyBR is a Hybrid Bidiagonalization Regularization method used for solving
* large-scale, ill-posed inverse problems of the form: b = A*x + noise The
* method combines an iterative Lanczos Bidiagonalization (LBD) Method with an
* SVD-based regularization method to stabilize the semiconvergence behavior
* that is characteristic of many ill-posed problems. The code is derived from
* RestoreTools: An Object Oriented Matlab Package for Image Restoration written
* by James G. Nagy and several of his students, including Julianne Chung,
* Katrina Palmer, Lisa Perrone, and Ryan Wright.
*
*
* References:
*
* [1] Paige and Saunders, "LSQR an algorithm for sparse linear equations an
* sparse least squares", ACM Trans. Math Software, 8 (1982), pp. 43-71.
*
*
* [2] Bjorck, Grimme and Van Dooren, "An implicit shift bidiagonalization
* algorithm for ill-posed systems", BIT 34 (11994), pp. 520-534.
*
*
* [3] Chung, Nagy and O'Leary, "A Weighted GCV Method for Lanczos Hybrid
* Regularization", Elec. Trans. Numer. Anal., 28 (2008), pp. 149--167.
*
*
*
* @author Piotr Wendykier ([email protected])
*
*/
public class DoubleHyBR extends AbstractDoubleIterativeSolver {
private HyBRInnerSolver innerSolver;
private HyBRRegularizationMethod regMethod;
private double regPar;
private double omega;
private boolean reorth;
private int begReg;
private double flatTol;
private boolean computeRnrm;
private static final DenseDoubleAlgebra alg = DenseDoubleAlgebra.DEFAULT;
private static final double FMIN_TOL = 1.0e-4;
/**
* Creates new instance of HyBR solver with default parameters:
*
* innerSolver = HyBR.InnerSolver.TIKHONOV
* regularizationMethod = HyBR.RegularizationMethod.ADAPTWGCV
* regularizationParameter = 0
* omega = 0
* reorthogonalize = false
* beginRegularization = 2
* flatTolerance = 1e-6
* computeRnrm = false;
*/
public DoubleHyBR() {
this(HyBRInnerSolver.TIKHONOV, HyBRRegularizationMethod.ADAPTWGCV, 0, 0, false, 2, 1e-6, false);
}
/**
* Creates new instance of HyBR solver.
*
* @param innerSolver
* solver for the inner problem
* @param regularizationMethod
* a method for choosing a regularization parameter
* @param regularizationParameter
* if regularizationMethod == HyBR.RegularizationMethod.NONE then
* the regularization parameter has to be specified here (value
* from the interval (0,1))
* @param omega
* regularizationMethod == HyBR.RegularizationMethod.WGCV then
* omega has to be specified here (must be nonnegative)
* @param reorthogonalize
* if thue then Lanczos subspaces are reorthogonalized
* @param beginRegularization
* begin regularization after this iteration (must be at least 2)
* @param flatTolerance
* tolerance for detecting flatness in the GCV curve as a
* stopping criteria (must be nonnegative)
*@param computeRnrm
* if true then the norm of relative residual is computed
*/
public DoubleHyBR(HyBRInnerSolver innerSolver, HyBRRegularizationMethod regularizationMethod,
double regularizationParameter, double omega, boolean reorthogonalize, int beginRegularization,
double flatTolerance, boolean computeRnrm) {
this.innerSolver = innerSolver;
this.regMethod = regularizationMethod;
if ((regularizationParameter < 0.0) || (regularizationParameter > 1.0)) {
throw new IllegalArgumentException("regularizationParameter must be a number between 0 and 1.");
}
this.regPar = regularizationParameter;
if (omega < 0.0) {
throw new IllegalArgumentException("omega must be a nonnegative number.");
}
this.omega = omega;
this.reorth = reorthogonalize;
if (beginRegularization < 2) {
throw new IllegalArgumentException("beginRegularization must be greater or equal 2");
}
this.begReg = beginRegularization;
if (flatTolerance < 0.0) {
throw new IllegalArgumentException("flatTolerance must be a nonnegative number.");
}
this.flatTol = flatTolerance;
this.computeRnrm = computeRnrm;
this.iter = new HyBRDoubleIterationMonitor();
}
public DoubleMatrix1D solve(DoubleMatrix2D A, DoubleMatrix1D b, DoubleMatrix1D x)
throws IterativeSolverDoubleNotConvergedException {
if (!(iter instanceof HyBRDoubleIterationMonitor)) {
this.iter = new HyBRDoubleIterationMonitor();
}
checkSizes(A, b, x);
int rows = A.rows();
int columns = A.columns();
boolean bump = false;
boolean warning = false;
double rnrm = -1.0;
int iterationsSave = 0;
double alpha = 0;
double alphaSave = 0;
double beta = 0;
HyBRInnerSolver inSolver = HyBRInnerSolver.NONE;
DoubleLBD lbd;
DoubleMatrix1D v;
DoubleMatrix1D work;
DoubleMatrix2D Ub, Vb;
DoubleMatrix1D f = null;
DoubleMatrix1D xSave = null;
double[] sv;
DoubleArrayList omegaList = new DoubleArrayList(new double[begReg - 2]);
DoubleArrayList GCV = new DoubleArrayList(new double[begReg - 2]);
DoubleMatrix2D U = new DenseDoubleMatrix2D(1, (int) b.size());
DoubleMatrix2D C = null;
DoubleMatrix2D V = null;
DenseDoubleSingularValueDecomposition svd;
if (computeRnrm) {
work = b.copy();
A.zMult(x, work, -1, 1, false);
rnrm = alg.norm2(work);
}
if (M instanceof DoubleIdentity) {
beta = alg.norm2(b);
U.viewRow(0).assign(b, DoubleFunctions.multSecond(1.0 / beta));
lbd = new DoubleSimpleLBD(A, U, reorth);
} else {
work = new DenseDoubleMatrix1D((int) b.size());
work = M.apply(b, work);
beta = alg.norm2(work);
U.viewRow(0).assign(work, DoubleFunctions.multSecond(1.0 / beta));
lbd = new DoublePLBD(M, A, U, reorth);
}
for (iter.setFirst(); !iter.converged(rnrm, x); iter.next()) {
lbd.apply();
U = lbd.getU();
C = lbd.getC();
V = lbd.getV();
v = new DenseDoubleMatrix1D(C.columns() + 1);
v.setQuick(0, beta);
int i = iter.iterations();
if (i >= 1) {
if (i >= begReg - 1) {
inSolver = innerSolver;
}
switch (inSolver) {
case TIKHONOV:
svd = alg.svd(C);
Ub = svd.getU();
sv = svd.getSingularValues();
Vb = svd.getV();
if (regMethod == HyBRRegularizationMethod.ADAPTWGCV) {
work = new DenseDoubleMatrix1D(Ub.rows());
Ub.zMult(v, work, 1, 0, true);
omegaList.add(Math.min(1, findOmega(work, sv)));
omega = DoubleDescriptive.mean(omegaList);
}
f = new DenseDoubleMatrix1D(Vb.rows());
alpha = tikhonovSolver(Ub, sv, Vb, v, f);
GCV.add(GCVstopfun(alpha, Ub.viewRow(0), sv, beta, rows, columns));
((HyBRDoubleIterationMonitor) iter).setRegularizationParameter(alpha);
if (i > 1) {
if (Math.abs((GCV.getQuick(i - 1) - GCV.getQuick(i - 2))) / GCV.get(begReg - 2) < flatTol) {
V.zMult(f, x);
((HyBRDoubleIterationMonitor) iter)
.setStoppingCondition(HyBRDoubleIterationMonitor.HyBRStoppingCondition.FLAT_GCV_CURVE);
if (computeRnrm) {
work = b.copy();
A.zMult(x, work, -1, 1, false);
((HyBRDoubleIterationMonitor) iter).residual = alg.norm2(work);
}
return x;
} else if ((warning == true) && (GCV.size() > iterationsSave + 3)) {
for (int j = iterationsSave; j < GCV.size(); j++) {
if (GCV.getQuick(iterationsSave - 1) > GCV.get(j)) {
bump = true;
}
}
if (bump == false) {
x.assign(xSave);
((HyBRDoubleIterationMonitor) iter)
.setStoppingCondition(HyBRDoubleIterationMonitor.HyBRStoppingCondition.MIN_OF_GCV_CURVE_WITHIN_WINDOW_OF_4_ITERATIONS);
((HyBRDoubleIterationMonitor) iter).iter = iterationsSave;
if (computeRnrm) {
work = b.copy();
A.zMult(x, work, -1, 1, false);
((HyBRDoubleIterationMonitor) iter).residual = alg.norm2(work);
}
((HyBRDoubleIterationMonitor) iter).setRegularizationParameter(alphaSave);
return x;
} else {
bump = false;
warning = false;
iterationsSave = iter.getMaxIterations();
}
} else if (warning == false) {
if (GCV.get(i - 2) < GCV.get(i - 1)) {
warning = true;
xSave = new DenseDoubleMatrix1D(V.rows());
alphaSave = alpha;
V.zMult(f, xSave);
iterationsSave = i;
}
}
}
break;
case NONE:
f = alg.solve(C, v);
break;
}
V.zMult(f, x);
if (computeRnrm) {
work = b.copy();
A.zMult(x, work, -1, 1, false);
rnrm = alg.norm2(work);
}
}
}
return x;
}
protected void checkSizes(DoubleMatrix2D A, DoubleMatrix1D b, DoubleMatrix1D x) {
if (b.size() != A.rows())
throw new IllegalArgumentException("b.size() != A.rows()");
if (x.size() != A.columns())
throw new IllegalArgumentException("x.size() != A.columns()");
}
private double findOmega(DoubleMatrix1D bhat, double[] s) {
int m = (int) bhat.size();
int n = s.length;
double alpha = s[n - 1];
double t0 = bhat.viewPart(n, m - n).aggregate(DoubleFunctions.plus, DoubleFunctions.square);
DoubleMatrix1D s2 = new DenseDoubleMatrix1D(s);
s2.assign(DoubleFunctions.square);
double alpha2 = alpha * alpha;
DoubleMatrix1D tt = s2.copy();
tt.assign(DoubleFunctions.plus(alpha2));
tt.assign(DoubleFunctions.inv);
double t1 = s2.aggregate(tt, DoubleFunctions.plus, DoubleFunctions.mult);
s2 = new DenseDoubleMatrix1D(s);
s2.assign(DoubleFunctions.mult(alpha));
s2.assign(bhat.viewPart(0, n), DoubleFunctions.mult);
s2.assign(DoubleFunctions.square);
DoubleMatrix1D work = tt.copy();
work.assign(DoubleFunctions.pow(3));
work.assign(DoubleFunctions.abs);
double t3 = work.aggregate(s2, DoubleFunctions.plus, DoubleFunctions.mult);
work = new DenseDoubleMatrix1D(s);
work.assign(tt, DoubleFunctions.mult);
double t4 = work.aggregate(DoubleFunctions.plus, DoubleFunctions.square);
work = tt.copy();
work.assign(bhat.viewPart(0, n), DoubleFunctions.mult);
work.assign(DoubleFunctions.mult(alpha2));
double t5 = work.aggregate(DoubleFunctions.plus, DoubleFunctions.square);
s2 = new DenseDoubleMatrix1D(s);
s2.assign(bhat.viewPart(0, n), DoubleFunctions.mult);
s2.assign(DoubleFunctions.square);
tt.assign(DoubleFunctions.pow(3));
tt.assign(DoubleFunctions.abs);
double v2 = tt.aggregate(s2, DoubleFunctions.plus, DoubleFunctions.mult);
return (m * alpha2 * v2) / (t1 * t3 + t4 * (t5 + t0));
}
private double tikhonovSolver(DoubleMatrix2D U, double[] s, DoubleMatrix2D V, DoubleMatrix1D b, DoubleMatrix1D x) {
TikFmin2D fmin;
DoubleMatrix1D bhat = new DenseDoubleMatrix1D(U.rows());
U.zMult(b, bhat, 1, 0, true);
double alpha = 0;
switch (regMethod) {
case GCV:
fmin = new TikFmin2D(bhat, s, 1);
alpha = DoubleFmin.fmin(0, s[0], fmin, FMIN_TOL);
break;
case WGCV:
fmin = new TikFmin2D(bhat, s, omega);
alpha = DoubleFmin.fmin(0, s[0], fmin, FMIN_TOL);
break;
case ADAPTWGCV:
fmin = new TikFmin2D(bhat, s, omega);
alpha = DoubleFmin.fmin(0, s[0], fmin, FMIN_TOL);
break;
case NONE: // regularization parameter is given
alpha = regPar;
break;
}
DoubleMatrix1D d = new DenseDoubleMatrix1D(s);
d.assign(DoubleFunctions.square);
d.assign(DoubleFunctions.plus(alpha * alpha));
bhat = bhat.viewPart(0, s.length);
DoubleMatrix1D S = new DenseDoubleMatrix1D(s);
bhat.assign(S, DoubleFunctions.mult);
bhat.assign(d, DoubleFunctions.div);
V.zMult(bhat, x);
return alpha;
}
private static class TikFmin2D implements DoubleFmin_methods {
DoubleMatrix1D bhat;
double[] s;
double omega;
public TikFmin2D(DoubleMatrix1D bhat, double[] s, double omega) {
this.bhat = bhat;
this.s = s;
this.omega = omega;
}
public double f_to_minimize(double alpha) {
int m = (int) bhat.size();
int n = s.length;
double t0 = bhat.viewPart(n, m - n).aggregate(DoubleFunctions.plus, DoubleFunctions.square);
DoubleMatrix1D s2 = new DenseDoubleMatrix1D(s);
s2.assign(DoubleFunctions.square);
double alpha2 = alpha * alpha;
DoubleMatrix1D work = s2.copy();
work.assign(DoubleFunctions.plus(alpha2));
work.assign(DoubleFunctions.inv);
DoubleMatrix1D t1 = work.copy();
t1.assign(DoubleFunctions.mult(alpha2));
DoubleMatrix1D t2 = t1.copy();
t2.assign(bhat.viewPart(0, n), DoubleFunctions.mult);
DoubleMatrix1D t3 = work.copy();
t3.assign(s2, DoubleFunctions.mult);
t3.assign(DoubleFunctions.mult(1 - omega));
double denom = t3.aggregate(t1, DoubleFunctions.plus, DoubleFunctions.plus) + m - n;
return n * (t2.aggregate(DoubleFunctions.plus, DoubleFunctions.square) + t0) / (denom * denom);
}
}
private double GCVstopfun(double alpha, DoubleMatrix1D u, double[] s, double beta, int rows, int columns) {
int k = s.length;
double beta2 = beta * beta;
DoubleMatrix1D s2 = new DenseDoubleMatrix1D(s);
s2.assign(DoubleFunctions.square);
double alpha2 = alpha * alpha;
DoubleMatrix1D t1 = s2.copy();
t1.assign(DoubleFunctions.plus(alpha2));
t1.assign(DoubleFunctions.inv);
DoubleMatrix1D t2 = t1.copy();
t2.assign(u.viewPart(0, k), DoubleFunctions.mult);
t2.assign(DoubleFunctions.mult(alpha2));
double num = beta2
* (t2.aggregate(DoubleFunctions.plus, DoubleFunctions.square) + Math.pow(Math.abs(u.getQuick(k)), 2))
/ columns;
double den = (rows - t1.aggregate(s2, DoubleFunctions.plus, DoubleFunctions.mult)) / columns;
den = den * den;
return num / den;
}
private interface DoubleLBD {
public void apply();
public DoubleMatrix2D getC();
public DoubleMatrix2D getU();
public DoubleMatrix2D getV();
}
private class DoubleSimpleLBD implements DoubleLBD {
private final DenseDoubleAlgebra alg = DenseDoubleAlgebra.DEFAULT;
private final DoubleFactory2D factory = DoubleFactory2D.dense;
private final DoubleMatrix2D alphaBeta = new DenseDoubleMatrix2D(2, 1);
private final DoubleMatrix2D A;
private DoubleMatrix2D C;
private DoubleMatrix2D U;
private DoubleMatrix2D V;
private boolean reorth;
private int counter = 1;
public DoubleSimpleLBD(DoubleMatrix2D A, DoubleMatrix2D U, boolean reorth) {
this.A = A;
this.reorth = reorth;
this.U = U;
this.V = null;
this.C = null;
}
public void apply() {
if (reorth) {
int k = U.rows();
DoubleMatrix1D u = null;
DoubleMatrix1D v = null;
DoubleMatrix1D column = null;
if (k == 1) {
v = A.zMult(U.viewRow(k - 1), v, 1, 0, true);
} else {
v = A.zMult(U.viewRow(k - 1), v, 1, 0, true);
column = V.viewColumn(k - 2);
v.assign(column, DoubleFunctions.plusMultSecond(-C.getQuick(k - 1, k - 2)));
for (int j = 0; j < k - 1; j++) {
column = V.viewColumn(j);
v.assign(column, DoubleFunctions.plusMultSecond(-column.zDotProduct(v)));
}
}
double alpha = alg.norm2(v);
v.assign(DoubleFunctions.div(alpha));
u = A.zMult(v, u);
column = U.viewRow(k - 1);
u.assign(column, DoubleFunctions.plusMultSecond(-alpha));
for (int j = 0; j < k; j++) {
column = U.viewRow(j);
u.assign(column, DoubleFunctions.plusMultSecond(-column.zDotProduct(u)));
}
double beta = alg.norm2(u);
alphaBeta.setQuick(0, 0, alpha);
alphaBeta.setQuick(1, 0, beta);
u.assign(DoubleFunctions.div(beta));
U = factory.appendRow(U, u);
if (V == null) {
V = new DenseColumnDoubleMatrix2D((int) v.size(), 1);
V.assign((double[]) v.elements());
} else {
V = factory.appendColumn(V, v);
}
if (C == null) {
C = new DenseDoubleMatrix2D(2, 1);
C.assign(alphaBeta);
} else {
C = factory.composeBidiagonal(C, alphaBeta);
}
} else {
DoubleMatrix1D u = null;
DoubleMatrix1D v = null;
DoubleMatrix1D column = null;
if (counter == 1) {
v = A.zMult(U.viewRow(0), v, 1, 0, true);
} else {
v = A.zMult(U.viewRow(0), v, 1, 0, true);
column = V.viewColumn(counter - 2);
v.assign(column, DoubleFunctions.plusMultSecond(-C.getQuick(counter - 1, counter - 2)));
}
double alpha = alg.norm2(v);
v.assign(DoubleFunctions.div(alpha));
u = A.zMult(v, u);
column = U.viewRow(0);
u.assign(column, DoubleFunctions.plusMultSecond(-alpha));
double beta = alg.norm2(u);
alphaBeta.setQuick(0, 0, alpha);
alphaBeta.setQuick(1, 0, beta);
u.assign(DoubleFunctions.div(beta));
U.viewRow(0).assign(u);
if (V == null) {
V = new DenseColumnDoubleMatrix2D((int) v.size(), 1);
V.assign((double[]) v.elements());
} else {
V = factory.appendColumn(V, v);
}
if (C == null) {
C = new DenseDoubleMatrix2D(2, 1);
C.assign(alphaBeta);
} else {
C = factory.composeBidiagonal(C, alphaBeta);
}
counter++;
}
}
public DoubleMatrix2D getC() {
return C;
}
public DoubleMatrix2D getU() {
return U;
}
public DoubleMatrix2D getV() {
return V;
}
}
private class DoublePLBD implements DoubleLBD {
private final DenseDoubleAlgebra alg = DenseDoubleAlgebra.DEFAULT;
private final DoubleFactory2D factory = DoubleFactory2D.dense;
private final DoubleMatrix2D alphaBeta = new DenseDoubleMatrix2D(2, 1);
private final DoublePreconditioner M;
private final DoubleMatrix2D A;
private DoubleMatrix2D C;
private DoubleMatrix2D U;
private DoubleMatrix2D V;
private boolean reorth;
private int counter = 1;
public DoublePLBD(DoublePreconditioner M, DoubleMatrix2D A, DoubleMatrix2D U, boolean reorth) {
this.M = M;
this.A = A;
this.reorth = reorth;
this.U = U;
this.V = null;
this.C = null;
}
public void apply() {
if (reorth) {
int k = U.rows();
DoubleMatrix1D u = null;
DoubleMatrix1D v = null;
DoubleMatrix1D row = null;
if (k == 1) {
row = U.viewRow(k - 1).copy();
row = M.transApply(row, row);
v = A.zMult(row, v, 1, 0, true);
} else {
row = U.viewRow(k - 1).copy();
row = M.transApply(row, row);
v = A.zMult(row, v, 1, 0, true);
row = V.viewColumn(k - 2);
v.assign(row, DoubleFunctions.plusMultSecond(-C.getQuick(k - 1, k - 2)));
for (int j = 0; j < k - 1; j++) {
row = V.viewColumn(j);
v.assign(row, DoubleFunctions.plusMultSecond(-row.zDotProduct(v)));
}
}
double alpha = alg.norm2(v);
v.assign(DoubleFunctions.div(alpha));
row = A.zMult(v, row);
u = M.apply(row, u);
row = U.viewRow(k - 1);
u.assign(row, DoubleFunctions.plusMultSecond(-alpha));
for (int j = 0; j < k; j++) {
row = U.viewRow(j);
u.assign(row, DoubleFunctions.plusMultSecond(-row.zDotProduct(u)));
}
double beta = alg.norm2(u);
alphaBeta.setQuick(0, 0, alpha);
alphaBeta.setQuick(1, 0, beta);
u.assign(DoubleFunctions.div(beta));
U = factory.appendRow(U, u);
if (V == null) {
V = new DenseColumnDoubleMatrix2D((int) v.size(), 1);
V.assign((double[]) v.elements());
} else {
V = factory.appendColumn(V, v);
}
if (C == null) {
C = new DenseDoubleMatrix2D(2, 1);
C.assign(alphaBeta);
} else {
C = factory.composeBidiagonal(C, alphaBeta);
}
} else {
DoubleMatrix1D u = null;
DoubleMatrix1D v = null;
DoubleMatrix1D row = null;
if (counter == 1) {
row = U.viewRow(0).copy();
row = M.transApply(row, row);
v = A.zMult(row, v, 1, 0, true);
} else {
row = U.viewRow(0).copy();
row = M.transApply(row, row);
v = A.zMult(row, v, 1, 0, true);
row = V.viewColumn(counter - 2);
v.assign(row, DoubleFunctions.plusMultSecond(-C.getQuick(counter - 1, counter - 2)));
}
double alpha = alg.norm2(v);
v.assign(DoubleFunctions.div(alpha));
row = A.zMult(v, row);
u = M.apply(row, u);
row = U.viewRow(0);
u.assign(row, DoubleFunctions.plusMultSecond(-alpha));
double beta = alg.norm2(u);
alphaBeta.setQuick(0, 0, alpha);
alphaBeta.setQuick(1, 0, beta);
u.assign(DoubleFunctions.div(beta));
U.viewRow(0).assign(u);
if (V == null) {
V = new DenseColumnDoubleMatrix2D((int) v.size(), 1);
V.assign((double[]) v.elements());
} else {
V = factory.appendColumn(V, v);
}
if (C == null) {
C = new DenseDoubleMatrix2D(2, 1);
C.assign(alphaBeta);
} else {
C = factory.composeBidiagonal(C, alphaBeta);
}
counter++;
}
}
public DoubleMatrix2D getC() {
return C;
}
public DoubleMatrix2D getU() {
return U;
}
public DoubleMatrix2D getV() {
return V;
}
}
}
© 2015 - 2025 Weber Informatics LLC | Privacy Policy