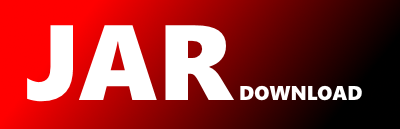
cern.colt.matrix.tdouble.algo.solver.DoubleMRNSD Maven / Gradle / Ivy
Go to download
Show more of this group Show more artifacts with this name
Show all versions of parallelcolt Show documentation
Show all versions of parallelcolt Show documentation
Parallel Colt is a multithreaded version of Colt - a library for high performance scientific computing in Java. It contains efficient algorithms for data analysis, linear algebra, multi-dimensional arrays, Fourier transforms, statistics and histogramming.
The newest version!
/*
* Copyright (C) 2009 Piotr Wendykier
*
* This program is free software; you can redistribute it and/or modify it
* under the terms of the GNU Lesser General Public License as published by the
* Free Software Foundation; either version 2.1 of the License, or (at your
* option) any later version.
*
* This library is distributed in the hope that it will be useful, but WITHOUT
* ANY WARRANTY; without even the implied warranty of MERCHANTABILITY or
* FITNESS FOR A PARTICULAR PURPOSE. See the GNU Lesser General Public License
* for more details.
*
* You should have received a copy of the GNU Lesser General Public License
* along with this library; if not, write to the Free Software Foundation,
* Inc., 59 Temple Place, Suite 330, Boston, MA 02111-1307 USA
*/
package cern.colt.matrix.tdouble.algo.solver;
import cern.colt.list.tint.IntArrayList;
import cern.colt.matrix.tdouble.DoubleMatrix1D;
import cern.colt.matrix.tdouble.DoubleMatrix2D;
import cern.colt.matrix.tdouble.algo.DenseDoubleAlgebra;
import cern.colt.matrix.tdouble.algo.solver.preconditioner.DoubleIdentity;
import cern.jet.math.tdouble.DoubleFunctions;
/**
* MRNSD is Modified Residual Norm Steepest Descent method used for solving
* large-scale, ill-posed inverse problems of the form: b = A*x + noise. This
* algorithm is nonnegatively constrained.
*
*
* References:
*
* [1] J. Nagy, Z. Strakos,
* "Enforcing nonnegativity in image reconstruction algorithms" in Mathematical
* Modeling, Estimation, and Imaging, David C. Wilson, et.al., Eds., 4121
* (2000), pg. 182--190.
*
*
* [2] L. Kaufman, "Maximum likelihood, least squares and penalized least
* squares for PET", IEEE Trans. Med. Imag. 12 (1993) pp. 200--214.
*
*
* @author Piotr Wendykier ([email protected])
*
*/
public class DoubleMRNSD extends AbstractDoubleIterativeSolver {
private static final DenseDoubleAlgebra alg = DenseDoubleAlgebra.DEFAULT;
public static final double sqrteps = Math.sqrt(Math.pow(2, -52));
public DoubleMRNSD() {
iter = new MRNSDDoubleIterationMonitor();
((MRNSDDoubleIterationMonitor) iter).setRelativeTolerance(-1);
}
public DoubleMatrix1D solve(DoubleMatrix2D A, DoubleMatrix1D b, DoubleMatrix1D x)
throws IterativeSolverDoubleNotConvergedException {
if (!(iter instanceof MRNSDDoubleIterationMonitor)) {
iter = new MRNSDDoubleIterationMonitor();
((MRNSDDoubleIterationMonitor) iter).setRelativeTolerance(-1);
}
double alpha;
double gamma;
double theta;
double rnrm;
DoubleMatrix1D r, s, u, w;
IntArrayList indexList;
double tau = sqrteps;
double sigsq = tau;
// x.assign(b); // x cannot be 0
double[] minAndLoc = x.getMinLocation();
double minX = minAndLoc[0];
if (minX < 0) {
x.assign(DoubleFunctions.plus(-minX + sigsq));
}
if (((MRNSDDoubleIterationMonitor) iter).getRelativeTolerance() == -1.0) {
((MRNSDDoubleIterationMonitor) iter)
.setRelativeTolerance(sqrteps * alg.norm2(A.zMult(b, null, 1, 0, true)));
}
r = A.zMult(x, null);
r.assign(b, DoubleFunctions.plusMultFirst(-1));
if (!(M instanceof DoubleIdentity)) {
r = M.apply(r, null);
r = M.transApply(r, null);
r = A.zMult(r, null, 1, 0, true);
r.assign(DoubleFunctions.neg);
gamma = x.aggregate(r, DoubleFunctions.plus, DoubleFunctions.multSquare);
rnrm = alg.norm2(r);
} else {
r = A.zMult(r, null, 1, 0, true);
r.assign(DoubleFunctions.neg);
gamma = x.aggregate(r, DoubleFunctions.plus, DoubleFunctions.multSquare);
rnrm = Math.sqrt(gamma);
}
indexList = new IntArrayList((int) b.size());
for (iter.setFirst(); !iter.converged(rnrm, x); iter.next()) {
s = x.copy();
s.assign(r, DoubleFunctions.multNeg);
u = A.zMult(s, null);
if (!(M instanceof DoubleIdentity)) {
u = M.apply(u, null);
}
theta = gamma / u.aggregate(DoubleFunctions.plus, DoubleFunctions.square);
s.getNegativeValues(indexList, null);
w = x.copy();
w.assign(s, DoubleFunctions.divNeg, indexList);
alpha = Math.min(theta, w.aggregate(DoubleFunctions.min, DoubleFunctions.identity, indexList));
x.assign(s, DoubleFunctions.plusMultSecond(alpha));
if (!(M instanceof DoubleIdentity)) {
w = M.transApply(u, null);
w = A.zMult(w, null, 1, 0, true);
r.assign(w, DoubleFunctions.plusMultSecond(alpha));
gamma = x.aggregate(r, DoubleFunctions.plus, DoubleFunctions.multSquare);
rnrm = alg.norm2(r);
} else {
w = A.zMult(u, null, 1, 0, true);
r.assign(w, DoubleFunctions.plusMultSecond(alpha));
gamma = x.aggregate(r, DoubleFunctions.plus, DoubleFunctions.multSquare);
rnrm = Math.sqrt(gamma);
}
}
return x;
}
}
© 2015 - 2025 Weber Informatics LLC | Privacy Policy