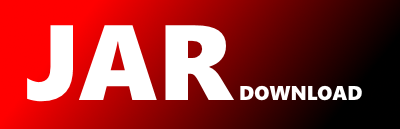
moa.classifiers.lazy.neighboursearch.kdtrees.KDTreeNodeSplitter Maven / Gradle / Ivy
Go to download
Massive On-line Analysis is an environment for massive data mining. MOA
provides a framework for data stream mining and includes tools for evaluation
and a collection of machine learning algorithms. Related to the WEKA project,
also written in Java, while scaling to more demanding problems.
Please wait ...
© 2015 - 2025 Weber Informatics LLC | Privacy Policy