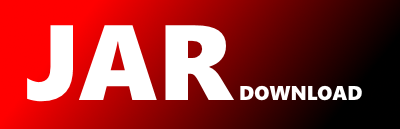
moa.classifiers.meta.ADACC Maven / Gradle / Ivy
Go to download
Show more of this group Show more artifacts with this name
Show all versions of moa Show documentation
Show all versions of moa Show documentation
Massive On-line Analysis is an environment for massive data mining. MOA
provides a framework for data stream mining and includes tools for evaluation
and a collection of machine learning algorithms. Related to the WEKA project,
also written in Java, while scaling to more demanding problems.
/*
* ADACC.java
*
* @author Ghazal Jaber ([email protected])
*
* Licensed under the Apache License, Version 2.0 (the "License");
* you may not use this file except in compliance with the License.
* You may obtain a copy of the License at
*
* http://www.apache.org/licenses/LICENSE-2.0
*
* Unless required by applicable law or agreed to in writing, software
* distributed under the License is distributed on an "AS IS" BASIS,
* WITHOUT WARRANTIES OR CONDITIONS OF ANY KIND, either express or implied.
* See the License for the specific language governing permissions and
* limitations under the License.
*
*
*/
package moa.classifiers.meta;
import moa.classifiers.Classifier;
import moa.core.Measurement;
import moa.options.FloatOption;
import moa.options.IntOption;
import weka.core.Instance;
import weka.core.Instances;
import weka.core.Utils;
import java.util.Arrays;
import java.util.Collections;
/**
* Anticipative and Dynamic Adaptation to Concept Changes.
* Ensemble method for data streams that adapts to concept changes
* and deals with concept recurrence.
*
* Reference: JABER, G., CORNUEJOLS, A., and TARROUX, P. A New On-Line Learning Method
* for Coping with Recurring Concepts: The ADACC System. In : Neural Information
* Processing. Springer Berlin Heidelberg, 2013. p. 595-604.
*
* @author Ghazal Jaber ([email protected])
*
*/
public class ADACC extends DACC {
private static final long serialVersionUID = 1L;
@Override
public String getPurposeString() {
return "Anticipative and Dynamic Adaptation to Concept Changes for data streams.";
}
/**
* Evaluation window for the stability index computation
*/
public IntOption tauSizeOption = new IntOption("tau", 't',
"The size of the evaluation window for the meta-learning.", 100, 1, 10000);
/**
* Threshold for the stability index
*/
public FloatOption stabIndexSizeOption = new FloatOption("StabThr", 'z',
"The threshold for stability", 0.8, 0, 1);
/**
* Threshold for concept equivalence
*/
public FloatOption equivIndexSizeOption = new FloatOption("CeThr", 'q',
"The threshold for concept equivalence", 0.7, 0, 1);
/**
* Size of the evaluation window to compute the stability index
*/
protected int tau_size = 0;
/**
* Last chunk of data of size (tau_size) to compute the stability index
*/
protected Instances recentChunk;
/**
* Threshold values for the stability index and concept equivalence
*/
protected double theta_stab, theta_diff;
/**
* Current stability index
*/
protected double index;
/**
* Maximum number of snapshots (copies of classifiers kept in case of recurrence)
*/
protected final static int MAXPERMANENT = 100;
/**
* Number of added snapshots
*/
protected int addedPermanent = 0;
@Override
protected void initVariables(){
this.tau_size = this.tauSizeOption.getValue();
this.theta_stab = this.stabIndexSizeOption.getValue();
this.theta_diff = this.equivIndexSizeOption.getValue();
this.recentChunk = null;
int ensembleSize = (int)this.memberCountOption.getValue() + MAXPERMANENT;
this.ensemble = new Classifier[ensembleSize];
this.ensembleAges = new double[ensembleSize];
this.ensembleWindows = new int[ensembleSize][(int)this.evaluationSizeOption.getValue()];
}
@Override
public void trainOnInstanceImpl(Instance inst) {
if (recentChunk == null)
recentChunk = new Instances(this.getModelContext());
if (recentChunk.size() < this.tau_size)
recentChunk.add(inst);
else
recentChunk.set(this.nbInstances % this.tau_size,inst);
trainAndClassify(inst);
if ((this.nbInstances % this.tau_size)==0)
takeSnapshot();
}
/**
* If the environment is stable enough, take a snapshot
* (a copy) of the best adaptive classifier and keep it
* for future use, in case of concept recurrence
*/
private void takeSnapshot(){
this.index = computeStabilityIndex();
if (this.index >= this.theta_stab)
if (addedPermanent == 0){
this.ensemble[this.ensemble.length-MAXPERMANENT+addedPermanent] = getBestAdaptiveClassifier().copy();
addedPermanent++;
}
else{
Classifier candidate = getBestAdaptiveClassifier().copy();
boolean duplicate = false;
for (int j=0;j=this.theta_diff){
duplicate = true; break;
}
}
if (!duplicate){
this.ensemble[this.ensemble.length-MAXPERMANENT+(addedPermanent%MAXPERMANENT)]=candidate;
addedPermanent++;
}
}
}
/**
* Returns the kappa statistics,
* a statistical measure of agreement in the predictions
* of 2 classifiers. Used as a measure of diversity of predictive
* models: the higher the kappa value, the smaller the diversity
* @param y1 the predictions of classifier A
* @param y2 the predictions of classifier B
* @return the kappa measure
*/
private double computeKappa(int[] y1,int[] y2){
int m=y1.length;
double theta1=0;
double counts[][]=new double[2][this.modelContext.numClasses()];
for (int i=0;i
© 2015 - 2025 Weber Informatics LLC | Privacy Policy