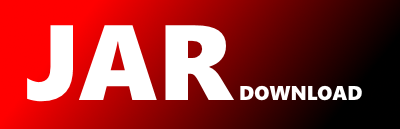
moa.classifiers.meta.OzaBagASHT Maven / Gradle / Ivy
Go to download
Show more of this group Show more artifacts with this name
Show all versions of moa Show documentation
Show all versions of moa Show documentation
Massive On-line Analysis is an environment for massive data mining. MOA
provides a framework for data stream mining and includes tools for evaluation
and a collection of machine learning algorithms. Related to the WEKA project,
also written in Java, while scaling to more demanding problems.
/*
* OzaBagASHT.java
* Copyright (C) 2008 University of Waikato, Hamilton, New Zealand
* @author Albert Bifet (abifet at cs dot waikato dot ac dot nz)
*
* This program is free software; you can redistribute it and/or modify
* it under the terms of the GNU General Public License as published by
* the Free Software Foundation; either version 3 of the License, or
* (at your option) any later version.
*
* This program is distributed in the hope that it will be useful,
* but WITHOUT ANY WARRANTY; without even the implied warranty of
* MERCHANTABILITY or FITNESS FOR A PARTICULAR PURPOSE. See the
* GNU General Public License for more details.
*
* You should have received a copy of the GNU General Public License
* along with this program. If not, see .
*
*/
package moa.classifiers.meta;
import moa.classifiers.Classifier;
import moa.classifiers.trees.ASHoeffdingTree;
import moa.core.DoubleVector;
import moa.core.MiscUtils;
import moa.options.IntOption;
import moa.options.FlagOption;
import weka.core.Instance;
import weka.core.Utils;
/**
* Bagging using trees of different size.
* The Adaptive-Size Hoeffding Tree (ASHT) is derived from the Hoeffding Tree
* algorithm with the following differences:
*
* - it has a maximum number of split nodes, or size
*
- after one node splits, if the number of split nodes of the ASHT tree
* is higher than the maximum value, then it deletes some nodes to reduce its size
*
* The intuition behind this method is as follows: smaller trees adapt
* more quickly to changes, and larger trees do better during periods with
* no or little change, simply because they were built on more data. Trees
* limited to size s will be reset about twice as often as trees with a size
* limit of 2s. This creates a set of different reset-speeds for an ensemble of such
* trees, and therefore a subset of trees that are a good approximation for the
* current rate of change. It is important to note that resets will happen all
* the time, even for stationary datasets, but this behaviour should not have
* a negative impact on the ensemble’s predictive performance.
* When the tree size exceeds the maximun size value, there are two different
* delete options:
* - delete the oldest node, the root, and all of its children except the one
* where the split has been made. After that, the root of the child not
* deleted becomes the new root
*
- delete all the nodes of the tree, i.e., restart from a new root.
*
* The maximum allowed size for the n-th ASHT tree is twice the maximum
* allowed size for the (n-1)-th tree. Moreover, each tree has a weight
* proportional to the inverse of the square of its error, and it monitors its
* error with an exponential weighted moving average (EWMA) with alpha = .01.
* The size of the first tree is 2.
*
* With this new method, it is attempted to improve bagging performance
* by increasing tree diversity. It has been observed that boosting tends to
* produce a more diverse set of classifiers than bagging, and this has been
* cited as a factor in increased performance.
* See more details in:
* Albert Bifet, Geoff Holmes, Bernhard Pfahringer, Richard Kirkby,
* and Ricard Gavaldà. New ensemble methods for evolving data
* streams. In 15th ACM SIGKDD International Conference on Knowledge
* Discovery and Data Mining, 2009.
* The learner must be ASHoeffdingTree, a Hoeffding Tree with a maximum
* size value.
* Example:
* OzaBagASHT -l ASHoeffdingTree -s 10 -u -r
* Parameters:
* - Same parameters as
OzaBag
* - -f : the size of first classifier in the bag.
*
- -u : Enable weight classifiers
*
- -e : Reset trees when size is higher than the max
*
*
* @author Albert Bifet (abifet at cs dot waikato dot ac dot nz)
* @version $Revision: 7 $
*/
public class OzaBagASHT extends OzaBag {
private static final long serialVersionUID = 1L;
@Override
public String getPurposeString() {
return "Bagging using trees of different size.";
}
public IntOption firstClassifierSizeOption = new IntOption("firstClassifierSize", 'f',
"The size of first classifier in the bag.", 1, 1, Integer.MAX_VALUE);
public FlagOption useWeightOption = new FlagOption("useWeight",
'u', "Enable weight classifiers.");
public FlagOption resetTreesOption = new FlagOption("resetTrees",
'e', "Reset trees when size is higher than the max.");
protected double[] error;
protected double alpha = 0.01;
@Override
public void resetLearningImpl() {
this.ensemble = new Classifier[this.ensembleSizeOption.getValue()];
this.error = new double[this.ensembleSizeOption.getValue()];
Classifier baseLearner = (Classifier) getPreparedClassOption(this.baseLearnerOption);
baseLearner.resetLearning();
int pow = this.firstClassifierSizeOption.getValue(); //EXTENSION TO ASHT
for (int i = 0; i < this.ensemble.length; i++) {
this.ensemble[i] = baseLearner.copy();
this.error[i] = 0.0;
((ASHoeffdingTree) this.ensemble[i]).setMaxSize(pow); //EXTENSION TO ASHT
if ((this.resetTreesOption != null)
&& this.resetTreesOption.isSet()) {
((ASHoeffdingTree) this.ensemble[i]).setResetTree();
}
pow *= 2; //EXTENSION TO ASHT
}
}
@Override
public void trainOnInstanceImpl(Instance inst) {
int trueClass = (int) inst.classValue();
for (int i = 0; i < this.ensemble.length; i++) {
int k = MiscUtils.poisson(1.0, this.classifierRandom);
if (k > 0) {
Instance weightedInst = (Instance) inst.copy();
weightedInst.setWeight(inst.weight() * k);
if (Utils.maxIndex(this.ensemble[i].getVotesForInstance(inst)) == trueClass) {
this.error[i] += alpha * (0.0 - this.error[i]); //EWMA
} else {
this.error[i] += alpha * (1.0 - this.error[i]); //EWMA
}
this.ensemble[i].trainOnInstance(weightedInst);
}
}
}
public double[] getVotesForInstance(Instance inst) {
DoubleVector combinedVote = new DoubleVector();
for (int i = 0; i < this.ensemble.length; i++) {
DoubleVector vote = new DoubleVector(this.ensemble[i].getVotesForInstance(inst));
if (vote.sumOfValues() > 0.0) {
vote.normalize();
if ((this.useWeightOption != null)
&& this.useWeightOption.isSet()) {
vote.scaleValues(1.0 / (this.error[i] * this.error[i]));
}
combinedVote.addValues(vote);
}
}
return combinedVote.getArrayRef();
}
@Override
public void getModelDescription(StringBuilder out, int indent) {
// TODO Auto-generated method stub
}
}
© 2015 - 2025 Weber Informatics LLC | Privacy Policy