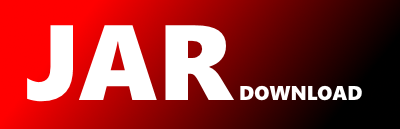
moa.clusterers.ClusterGenerator Maven / Gradle / Ivy
Go to download
Show more of this group Show more artifacts with this name
Show all versions of moa Show documentation
Show all versions of moa Show documentation
Massive On-line Analysis is an environment for massive data mining. MOA
provides a framework for data stream mining and includes tools for evaluation
and a collection of machine learning algorithms. Related to the WEKA project,
also written in Java, while scaling to more demanding problems.
/*
* ClusterGenerator.java
* Copyright (C) 2010 RWTH Aachen University, Germany
* @author Jansen ([email protected])
*
* Licensed under the Apache License, Version 2.0 (the "License");
* you may not use this file except in compliance with the License.
* You may obtain a copy of the License at
*
* http://www.apache.org/licenses/LICENSE-2.0
*
* Unless required by applicable law or agreed to in writing, software
* distributed under the License is distributed on an "AS IS" BASIS,
* WITHOUT WARRANTIES OR CONDITIONS OF ANY KIND, either express or implied.
* See the License for the specific language governing permissions and
* limitations under the License.
*
*
*/
package moa.clusterers;
import java.util.ArrayList;
import java.util.Arrays;
import java.util.Random;
import moa.cluster.Clustering;
import moa.cluster.SphereCluster;
import moa.core.Measurement;
import moa.gui.visualization.DataPoint;
import moa.options.FloatOption;
import moa.options.IntOption;
import weka.core.Instance;
public class ClusterGenerator extends AbstractClusterer{
private static final long serialVersionUID = 1L;
public IntOption timeWindowOption = new IntOption("timeWindow",
't', "Rang of the window.", 1000);
public FloatOption radiusDecreaseOption = new FloatOption("radiusDecrease", 'r',
"The average radii of the centroids in the model.", 0, 0, 1);
public FloatOption radiusIncreaseOption = new FloatOption("radiusIncrease", 'R',
"The average radii of the centroids in the model.", 0, 0, 1);
public FloatOption positionOffsetOption = new FloatOption("positionOffset", 'p',
"The average radii of the centroids in the model.", 0, 0, 1);
public FloatOption clusterRemoveOption = new FloatOption("clusterRemove", 'D',
"Deletes complete clusters from the clustering.", 0, 0, 1);
public FloatOption joinClustersOption = new FloatOption("joinClusters", 'j',
"Join two clusters if their hull distance is less minRadius times this factor.", 0, 0, 1);
public FloatOption clusterAddOption = new FloatOption("clusterAdd", 'A',
"Adds additional clusters.", 0, 0, 1);
private static double err_intervall_width = 0.0;
private ArrayList points;
private int instanceCounter;
private int windowCounter;
private Random random;
private Clustering sourceClustering = null;
@Override
public void resetLearningImpl() {
points = new ArrayList();
instanceCounter = 0;
windowCounter = 0;
random = new Random(227);
//joinClustersOption.set();
//evaluateMicroClusteringOption.set();
}
@Override
public void trainOnInstanceImpl(Instance inst) {
if(windowCounter >= timeWindowOption.getValue()){
points.clear();
windowCounter = 0;
}
windowCounter++;
instanceCounter++;
points.add( new DataPoint(inst,instanceCounter));
}
@Override
public boolean implementsMicroClusterer() {
return true;
}
public void setSourceClustering(Clustering source){
sourceClustering = source;
}
@Override
public Clustering getMicroClusteringResult() {
//System.out.println("Numcluster:"+clustering.size()+" / "+num);
//Clustering source_clustering = new Clustering(points, overlapThreshold, microInitMinPoints);
if(sourceClustering == null){
System.out.println("You need to set a source clustering for the ClusterGenerator to work");
return null;
}
return alterClustering(sourceClustering);
}
public Clustering getClusteringResult(){
sourceClustering = new Clustering(points);
// if(sourceClustering == null){
// System.out.println("You need to set a source clustering for the ClusterGenerator to work");
// return null;
// }
return alterClustering(sourceClustering);
}
private Clustering alterClustering(Clustering scclustering){
//percentage of the radius that will be cut off
//0: no changes to radius
//1: radius of 0
double errLevelRadiusDecrease = radiusDecreaseOption.getValue();
//0: no changes to radius
//1: radius 100% bigger
double errLevelRadiusIncrease = radiusIncreaseOption.getValue();
//0: no changes
//1: distance between centers is 2 * original radius
double errLevelPosition = positionOffsetOption.getValue();
int numRemoveCluster = (int)(clusterRemoveOption.getValue()*scclustering.size());
int numAddCluster = (int)(clusterAddOption.getValue()*scclustering.size());
for (int c = 0; c < numRemoveCluster; c++) {
int delId = random.nextInt(scclustering.size());
scclustering.remove(delId);
}
int numCluster = scclustering.size();
double[] err_seeds = new double[numCluster];
double err_seed_sum = 0.0;
double tmp_seed;
for (int i = 0; i < numCluster; i++) {
tmp_seed = random.nextDouble();
err_seeds[i] = err_seed_sum + tmp_seed;
err_seed_sum+= tmp_seed;
}
double sumWeight = 0;
for (int i = 0; i 0){
double errOffset = random.nextDouble()*err_intervall_width/2.0;
double errOffsetDirection = ((random.nextBoolean())? 1 : -1);
double level = errLevelPosition + errOffsetDirection * errOffset;
double[] vector = new double[center.length];
double vectorLength = 0;
for (int d = 0; d < center.length; d++) {
vector[d] = (random.nextBoolean()?1:-1)*random.nextDouble();
vectorLength += Math.pow(vector[d],2);
}
vectorLength = Math.sqrt(vectorLength);
//max is when clusters are next to each other
double length = 2 * radius * level;
for (int d = 0; d < center.length; d++) {
//normalize length and then strecht to reach error position
vector[d]=vector[d]/vectorLength*length;
}
// System.out.println("Center "+Arrays.toString(center));
// System.out.println("Vector "+Arrays.toString(vector));
//check if error position is within bounds
double [] newCenter = new double[center.length];
for (int d = 0; d < center.length; d++) {
//check bounds, otherwise flip vector
if(center[d] + vector[d] >= 0 && center[d] + vector[d] <= 1){
newCenter[d] = center[d] + vector[d];
}
else{
newCenter[d] = center[d] + (-1)*vector[d];
}
}
center = newCenter;
for (int d = 0; d < center.length; d++) {
if(newCenter[d] >= 0 && newCenter[d] <= 1){
}
else{
System.out.println("This shouldnt have happend, Cluster center out of bounds:"+Arrays.toString(newCenter));
}
}
//System.out.println("new Center "+Arrays.toString(newCenter));
}
//alter radius
if(errLevelRadiusDecrease > 0 || errLevelRadiusIncrease > 0){
double errOffset = random.nextDouble()*err_intervall_width/2.0;
int errOffsetDirection = ((random.nextBoolean())? 1 : -1);
if(errLevelRadiusDecrease > 0 && (errLevelRadiusIncrease == 0 || random.nextBoolean())){
double level = (errLevelRadiusDecrease + errOffsetDirection * errOffset);//*sourceCluster.getWeight()/sumWeight;
level = (level<0)?0:level;
level = (level>1)?1:level;
radius*=(1-level);
}
else{
double level = errLevelRadiusIncrease + errOffsetDirection * errOffset;
level = (level<0)?0:level;
level = (level>1)?1:level;
radius+=radius*level;
}
}
SphereCluster newCluster = new SphereCluster(center, radius, weight);
newCluster.setMeasureValue("Source Cluster", "C"+sourceCluster.getId());
clustering.add(newCluster);
}
if(joinClustersOption.getValue() > 0){
clustering = joinClusters(clustering);
}
//add new clusters by copying clusters and set a random center
for (int c = 0; c < numAddCluster; c++) {
int copyId = random.nextInt(clustering.size());
SphereCluster scorg = (SphereCluster)clustering.get(copyId);
int dim = scorg.getCenter().length;
double[] center = new double [dim];
double radius = scorg.getRadius();
boolean outofbounds = true;
int tryCounter = 0;
while(outofbounds && tryCounter < 20){
tryCounter++;
outofbounds = false;
for (int j = 0; j < center.length; j++) {
center[j] = random.nextDouble();
if(center[j]- radius < 0 || center[j] + radius > 1){
outofbounds = true;
break;
}
}
}
if(outofbounds){
System.out.println("Coludn't place additional cluster");
}
else{
SphereCluster scnew = new SphereCluster(center, radius, scorg.getWeight()/2);
scorg.setWeight(scorg.getWeight()-scnew.getWeight());
clustering.add(scnew);
}
}
return clustering;
}
private Clustering joinClusters(Clustering clustering){
double radiusFactor = joinClustersOption.getValue();
boolean[] merged = new boolean[clustering.size()];
Clustering mclustering = new Clustering();
if(radiusFactor >0){
for (int c1 = 0; c1 < clustering.size(); c1++) {
SphereCluster sc1 = (SphereCluster) clustering.get(c1);
double minDist = Double.MAX_VALUE;
double minOver = 1;
int maxindexCon = -1;
int maxindexOver = -1;
for (int c2 = 0; c2 < clustering.size(); c2++) {
SphereCluster sc2 = (SphereCluster) clustering.get(c2);
// double over = sc1.overlapRadiusDegree(sc2);
// if(over > 0 && over < minOver){
// minOver = over;
// maxindexOver = c2;
// }
double dist = sc1.getHullDistance(sc2);
double threshold = Math.min(sc1.getRadius(), sc2.getRadius())*radiusFactor;
if(dist > 0 && dist < minDist && dist < threshold){
minDist = dist;
maxindexCon = c2;
}
}
int maxindex = -1;
if(maxindexOver!=-1)
maxindex = maxindexOver;
else
maxindex = maxindexCon;
if(maxindex!=-1 && !merged[c1]){
merged[c1]=true;
merged[maxindex]=true;
SphereCluster scnew = new SphereCluster(sc1.getCenter(),sc1.getRadius(),sc1.getWeight());
SphereCluster sc2 = (SphereCluster) clustering.get(maxindex);
scnew.merge(sc2);
mclustering.add(scnew);
}
}
}
for (int i = 0; i < merged.length; i++) {
if(!merged[i])
mclustering.add(clustering.get(i));
}
return mclustering;
}
@Override
protected Measurement[] getModelMeasurementsImpl() {
throw new UnsupportedOperationException("Not supported yet.");
}
@Override
public void getModelDescription(StringBuilder out, int indent) {
throw new UnsupportedOperationException("Not supported yet.");
}
@Override
public boolean isRandomizable() {
return false;
}
@Override
public boolean keepClassLabel(){
return true;
}
public double[] getVotesForInstance(Instance inst) {
return null;
}
}
© 2015 - 2025 Weber Informatics LLC | Privacy Policy