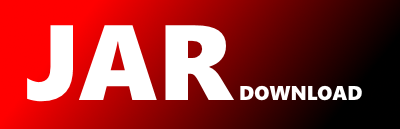
moa.clusterers.denstream.WithDBSCAN Maven / Gradle / Ivy
Go to download
Show more of this group Show more artifacts with this name
Show all versions of moa Show documentation
Show all versions of moa Show documentation
Massive On-line Analysis is an environment for massive data mining. MOA
provides a framework for data stream mining and includes tools for evaluation
and a collection of machine learning algorithms. Related to the WEKA project,
also written in Java, while scaling to more demanding problems.
/**
* Subspace MOA [DenStream_DBSCAN.java]
*
* DenStream with DBSCAN as the macro-clusterer.
*
* @author Stephan Wels ([email protected])
* @editor Yunsu Kim
* Data Management and Data Exploration Group, RWTH Aachen University
*
* Licensed under the Apache License, Version 2.0 (the "License");
* you may not use this file except in compliance with the License.
* You may obtain a copy of the License at
*
* http://www.apache.org/licenses/LICENSE-2.0
*
* Unless required by applicable law or agreed to in writing, software
* distributed under the License is distributed on an "AS IS" BASIS,
* WITHOUT WARRANTIES OR CONDITIONS OF ANY KIND, either express or implied.
* See the License for the specific language governing permissions and
* limitations under the License.
*
*
*/
package moa.clusterers.denstream;
import java.util.ArrayList;
import moa.cluster.Cluster;
import moa.cluster.Clustering;
import moa.clusterers.AbstractClusterer;
import moa.clusterers.macro.dbscan.DBScan;
import moa.core.Measurement;
import moa.options.FloatOption;
import moa.options.IntOption;
import weka.core.DenseInstance;
import weka.core.Instance;
public class WithDBSCAN extends AbstractClusterer {
private static final long serialVersionUID = 1L;
public IntOption horizonOption = new IntOption("horizon", 'h',
"Range of the window.", 1000);
public FloatOption epsilonOption = new FloatOption("epsilon", 'e',
"Defines the epsilon neighbourhood", 0.02, 0, 1);
// public IntOption minPointsOption = new IntOption("minPoints", 'p',
// "Minimal number of points cluster has to contain.", 10);
public FloatOption betaOption = new FloatOption("beta", 'b', "", 0.2, 0,
1);
public FloatOption muOption = new FloatOption("mu", 'm', "", 1, 0,
Double.MAX_VALUE);
public IntOption initPointsOption = new IntOption("initPoints", 'i',
"Number of points to use for initialization.", 1000);
public FloatOption offlineOption = new FloatOption("offline", 'o',
"offline multiplier for epsilion.", 2, 2, 20);
public FloatOption lambdaOption = new FloatOption("lambda", 'l', "",
0.25,
0, 1);
public IntOption speedOption = new IntOption("processingSpeed", 's',
"Number of incoming points per time unit.", 100, 1, 1000);
private double weightThreshold = 0.01;
double lambda;
double epsilon;
int minPoints;
double mu;
double beta;
Clustering p_micro_cluster;
Clustering o_micro_cluster;
ArrayList initBuffer;
boolean initialized;
private long timestamp = 0;
Timestamp currentTimestamp;
long tp;
/* #point variables */
protected int numInitPoints;
protected int numProcessedPerUnit;
protected int processingSpeed;
// TODO Some variables to prevent duplicated processes
private class DenPoint extends DenseInstance {
private static final long serialVersionUID = 1L;
protected boolean covered;
public DenPoint(Instance nextInstance, Long timestamp) {
super(nextInstance);
this.setDataset(nextInstance.dataset());
}
}
@Override
public void resetLearningImpl() {
// init DenStream
currentTimestamp = new Timestamp();
// lambda = -Math.log(weightThreshold) / Math.log(2)
// / (double) horizonOption.getValue();
lambda = lambdaOption.getValue();
epsilon = epsilonOption.getValue();
minPoints = (int) muOption.getValue();// minPointsOption.getValue();
mu = (int) muOption.getValue();
beta = betaOption.getValue();
initialized = false;
p_micro_cluster = new Clustering();
o_micro_cluster = new Clustering();
initBuffer = new ArrayList();
tp = Math.round(1 / lambda * Math.log((beta * mu) / (beta * mu - 1))) + 1;
numProcessedPerUnit = 0;
processingSpeed = speedOption.getValue();
}
public void initialDBScan() {
for (int p = 0; p < initBuffer.size(); p++) {
DenPoint point = initBuffer.get(p);
if (!point.covered) {
point.covered = true;
ArrayList neighbourhood = getNeighbourhoodIDs(point,
initBuffer, epsilon);
if (neighbourhood.size() > minPoints) {
MicroCluster mc = new MicroCluster(point,
point.numAttributes(), timestamp, lambda,
currentTimestamp);
expandCluster(mc, initBuffer, neighbourhood);
p_micro_cluster.add(mc);
} else {
point.covered = false;
}
}
}
}
@Override
public void trainOnInstanceImpl(Instance inst) {
DenPoint point = new DenPoint(inst, timestamp);
numProcessedPerUnit++;
/* Controlling the stream speed */
if (numProcessedPerUnit % processingSpeed == 0) {
timestamp++;
currentTimestamp.setTimestamp(timestamp);
}
// ////////////////
// Initialization//
// ////////////////
if (!initialized) {
initBuffer.add(point);
if (initBuffer.size() >= initPointsOption.getValue()) {
initialDBScan();
initialized = true;
}
} else {
// ////////////
// Merging(p)//
// ////////////
boolean merged = false;
if (p_micro_cluster.getClustering().size() != 0) {
MicroCluster x = nearestCluster(point, p_micro_cluster);
MicroCluster xCopy = x.copy();
xCopy.insert(point, timestamp);
if (xCopy.getRadius(timestamp) <= epsilon) {
x.insert(point, timestamp);
merged = true;
}
}
if (!merged && (o_micro_cluster.getClustering().size() != 0)) {
MicroCluster x = nearestCluster(point, o_micro_cluster);
MicroCluster xCopy = x.copy();
xCopy.insert(point, timestamp);
if (xCopy.getRadius(timestamp) <= epsilon) {
x.insert(point, timestamp);
merged = true;
if (x.getWeight() > beta * mu) {
o_micro_cluster.getClustering().remove(x);
p_micro_cluster.getClustering().add(x);
}
}
}
if (!merged) {
o_micro_cluster.getClustering().add(
new MicroCluster(point.toDoubleArray(), point
.toDoubleArray().length, timestamp, lambda,
currentTimestamp));
}
// //////////////////////////
// Periodic cluster removal//
// //////////////////////////
if (timestamp % tp == 0) {
ArrayList removalList = new ArrayList();
for (Cluster c : p_micro_cluster.getClustering()) {
if (((MicroCluster) c).getWeight() < beta * mu) {
removalList.add((MicroCluster) c);
}
}
for (Cluster c : removalList) {
p_micro_cluster.getClustering().remove(c);
}
for (Cluster c : o_micro_cluster.getClustering()) {
long t0 = ((MicroCluster) c).getCreationTime();
double xsi1 = Math
.pow(2, (-lambda * (timestamp - t0 + tp))) - 1;
double xsi2 = Math.pow(2, -lambda * tp) - 1;
double xsi = xsi1 / xsi2;
if (((MicroCluster) c).getWeight() < xsi) {
removalList.add((MicroCluster) c);
}
}
for (Cluster c : removalList) {
o_micro_cluster.getClustering().remove(c);
}
}
}
}
private void expandCluster(MicroCluster mc, ArrayList points,
ArrayList neighbourhood) {
for (int p : neighbourhood) {
DenPoint npoint = points.get(p);
if (!npoint.covered) {
npoint.covered = true;
mc.insert(npoint, timestamp);
ArrayList neighbourhood2 = getNeighbourhoodIDs(npoint,
initBuffer, epsilon);
if (neighbourhood.size() > minPoints) {
expandCluster(mc, points, neighbourhood2);
}
}
}
}
private ArrayList getNeighbourhoodIDs(DenPoint point,
ArrayList points, double eps) {
ArrayList neighbourIDs = new ArrayList();
for (int p = 0; p < points.size(); p++) {
DenPoint npoint = points.get(p);
if (!npoint.covered) {
double dist = distance(point.toDoubleArray(), points.get(p)
.toDoubleArray());
if (dist < eps) {
neighbourIDs.add(p);
}
}
}
return neighbourIDs;
}
private MicroCluster nearestCluster(DenPoint p, Clustering cl) {
MicroCluster min = null;
double minDist = 0;
for (int c = 0; c < cl.size(); c++) {
MicroCluster x = (MicroCluster) cl.get(c);
if (min == null) {
min = x;
}
double dist = distance(p.toDoubleArray(), x.getCenter());
dist -= x.getRadius(timestamp);
if (dist < minDist) {
minDist = dist;
min = x;
}
}
return min;
}
private double distance(double[] pointA, double[] pointB) {
double distance = 0.0;
for (int i = 0; i < pointA.length; i++) {
double d = pointA[i] - pointB[i];
distance += d * d;
}
return Math.sqrt(distance);
}
public Clustering getClusteringResult() {
DBScan dbscan = new DBScan(p_micro_cluster,offlineOption.getValue() * epsilon, minPoints);
return dbscan.getClustering(p_micro_cluster);
}
@Override
public boolean implementsMicroClusterer() {
return true;
}
@Override
public Clustering getMicroClusteringResult() {
return p_micro_cluster;
}
@Override
protected Measurement[] getModelMeasurementsImpl() {
throw new UnsupportedOperationException("Not supported yet.");
}
@Override
public void getModelDescription(StringBuilder out, int indent) {
}
public boolean isRandomizable() {
return true;
}
public double[] getVotesForInstance(Instance inst) {
return null;
}
public String getParameterString() {
StringBuffer sb = new StringBuffer();
sb.append(this.getClass().getSimpleName() + " ");
sb.append("-" + horizonOption.getCLIChar() + " ");
sb.append(horizonOption.getValueAsCLIString() + " ");
sb.append("-" + epsilonOption.getCLIChar() + " ");
sb.append(epsilonOption.getValueAsCLIString() + " ");
sb.append("-" + betaOption.getCLIChar() + " ");
sb.append(betaOption.getValueAsCLIString() + " ");
sb.append("-" + muOption.getCLIChar() + " ");
sb.append(muOption.getValueAsCLIString() + " ");
sb.append("-" + lambdaOption.getCLIChar() + " ");
sb.append(lambdaOption.getValueAsCLIString() + " ");
sb.append("-" + initPointsOption.getCLIChar() + " ");
// NO " " at the end! results in errors on windows systems
sb.append(initPointsOption.getValueAsCLIString());
return sb.toString();
}
}
© 2015 - 2025 Weber Informatics LLC | Privacy Policy