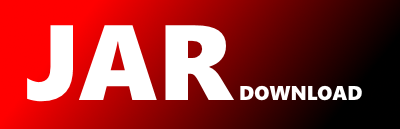
moa.clusterers.macro.AbstractMacroClusterer Maven / Gradle / Ivy
Go to download
Show more of this group Show more artifacts with this name
Show all versions of moa Show documentation
Show all versions of moa Show documentation
Massive On-line Analysis is an environment for massive data mining. MOA
provides a framework for data stream mining and includes tools for evaluation
and a collection of machine learning algorithms. Related to the WEKA project,
also written in Java, while scaling to more demanding problems.
/**
* [AbstractMacroClusterer.java] for Subspace MOA
*
* @author Stephen Wels
* Data Management and Data Exploration Group, RWTH Aachen University
*
* Licensed under the Apache License, Version 2.0 (the "License");
* you may not use this file except in compliance with the License.
* You may obtain a copy of the License at
*
* http://www.apache.org/licenses/LICENSE-2.0
*
* Unless required by applicable law or agreed to in writing, software
* distributed under the License is distributed on an "AS IS" BASIS,
* WITHOUT WARRANTIES OR CONDITIONS OF ANY KIND, either express or implied.
* See the License for the specific language governing permissions and
* limitations under the License.
*
*
*/
package moa.clusterers.macro;
import java.util.HashMap;
import java.util.List;
import java.util.Map;
import java.util.Vector;
import moa.cluster.Cluster;
import moa.cluster.Clustering;
public abstract class AbstractMacroClusterer {
public abstract Clustering getClustering(Clustering microClusters);
protected void setClusterIDs(Clustering clustering) {
// int numOfClusters = clustering.size();
// Set oldClusterIDs = new TreeSet();
//
// // Collect all the old IDs of the microclusters
// for (Cluster c : clustering.getClustering()) {
// NonConvexCluster ncc = (NonConvexCluster) c;
// for (Cluster mc : ncc.mMicroClusters) {
// if (!oldClusterIDs.contains(mc.getId()))
// oldClusterIDs.add(mc.getId());
// }
// }
HashMap countIDs = new HashMap();
for (Cluster c : clustering.getClustering()) {
HashMap ids = new HashMap();
NonConvexCluster ncc = (NonConvexCluster) c;
for (Cluster mc : ncc.getMicroClusters()) {
if (!ids.containsKey(mc.getId()))
ids.put(mc.getId(), new Integer(1));
else {
int i = ids.get(mc.getId());
i++;
ids.put(mc.getId(), i);
}
}
// find max
double maxID = -1d;
int max = -1;
for (Map.Entry entry : ids.entrySet()) {
if (entry.getValue() >= max) {
max = entry.getValue();
maxID = entry.getKey();
}
}
c.setId(maxID);
if (!countIDs.containsKey(maxID))
countIDs.put(maxID, new Integer(1));
else {
int i = countIDs.get(maxID);
i++;
countIDs.put(maxID, i);
}
}
// check if there are 2 clusters with the same color (same id, could
// appear after a split);
double freeID = 0;
List reservedIDs = new Vector();
reservedIDs.addAll(countIDs.keySet());
for (Map.Entry entry : countIDs.entrySet()) {
if (entry.getValue() > 1 || entry.getKey() == -1) {
// find first free id, search all the clusters which has the
// same id and replace the ids with free ids. One cluster can
// keep its id
int to = entry.getValue();
if (entry.getKey() != -1)
to--;
for (int i = 0; i < to; i++) {
while (reservedIDs.contains(freeID)
&& freeID < ColorArray.getNumColors())
freeID += 1.0;
for (int c = clustering.size() - 1; c >= 0; c--)
if (clustering.get(c).getId() == entry.getKey()) {
clustering.get(c).setId(freeID);
reservedIDs.add(freeID);
break;
}
}
}
}
for (Cluster c : clustering.getClustering()) {
NonConvexCluster ncc = (NonConvexCluster) c;
for (Cluster mc : ncc.getMicroClusters()) {
mc.setId(c.getId());
}
}
}
}
© 2015 - 2025 Weber Informatics LLC | Privacy Policy