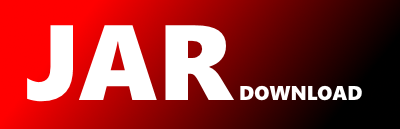
moa.recommender.rc.predictor.impl.BaselinePredictor Maven / Gradle / Ivy
Go to download
Show more of this group Show more artifacts with this name
Show all versions of moa Show documentation
Show all versions of moa Show documentation
Massive On-line Analysis is an environment for massive data mining. MOA
provides a framework for data stream mining and includes tools for evaluation
and a collection of machine learning algorithms. Related to the WEKA project,
also written in Java, while scaling to more demanding problems.
/*
* BaselinePredictor.java
* Copyright (C) 2012 Universitat Politecnica de Catalunya
* @author Alex Catarineu ([email protected])
*
* Licensed under the Apache License, Version 2.0 (the "License");
* you may not use this file except in compliance with the License.
* You may obtain a copy of the License at
*
* http://www.apache.org/licenses/LICENSE-2.0
*
* Unless required by applicable law or agreed to in writing, software
* distributed under the License is distributed on an "AS IS" BASIS,
* WITHOUT WARRANTIES OR CONDITIONS OF ANY KIND, either express or implied.
* See the License for the specific language governing permissions and
* limitations under the License.
*
*
*/
package moa.recommender.rc.predictor.impl;
import java.util.ArrayList;
import java.util.List;
import moa.recommender.rc.data.RecommenderData;
import moa.recommender.rc.predictor.RatingPredictor;
public class BaselinePredictor implements RatingPredictor {
/**
*
*/
private static final long serialVersionUID = 8444152568941483368L;
protected RecommenderData data;
public BaselinePredictor(RecommenderData data) {
this.data = data;
}
@Override
public double predictRating(int userID, int itemID) {
ArrayList itm = new ArrayList();
itm.add(itemID);
return predictRatings(userID, itm).get(0);
}
@Override
public List predictRatings(int userID, List itemIDS) {
ArrayList ret = new ArrayList(itemIDS.size());
double avg = data.getAvgRatingUser(userID) - data.getGlobalMean();
for (int i = 0; i < itemIDS.size(); ++i) {
int itemID = itemIDS.get(i);
double rat = avg + data.getAvgRatingItem(itemID);
rat = Math.min(Math.max(rat, data.getMinRating()),
data.getMaxRating());
ret.add(rat);
}
return ret;
}
@Override
public RecommenderData getData() {
return data;
}
@Override
public void train() {
}
}
© 2015 - 2025 Weber Informatics LLC | Privacy Policy