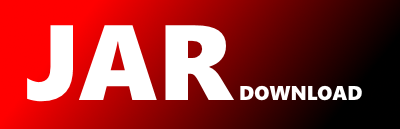
moa.tasks.EvaluateInterleavedTestThenTrain Maven / Gradle / Ivy
Go to download
Show more of this group Show more artifacts with this name
Show all versions of moa Show documentation
Show all versions of moa Show documentation
Massive On-line Analysis is an environment for massive data mining. MOA
provides a framework for data stream mining and includes tools for evaluation
and a collection of machine learning algorithms. Related to the WEKA project,
also written in Java, while scaling to more demanding problems.
/*
* EvaluateInterleavedTestThenTrain.java
* Copyright (C) 2007 University of Waikato, Hamilton, New Zealand
* @author Richard Kirkby ([email protected])
*
* This program is free software; you can redistribute it and/or modify
* it under the terms of the GNU General Public License as published by
* the Free Software Foundation; either version 3 of the License, or
* (at your option) any later version.
*
* This program is distributed in the hope that it will be useful,
* but WITHOUT ANY WARRANTY; without even the implied warranty of
* MERCHANTABILITY or FITNESS FOR A PARTICULAR PURPOSE. See the
* GNU General Public License for more details.
*
* You should have received a copy of the GNU General Public License
* along with this program. If not, see .
*
*/
package moa.tasks;
import java.io.File;
import java.io.FileOutputStream;
import java.io.PrintStream;
import moa.classifiers.Classifier;
import moa.core.Measurement;
import moa.core.ObjectRepository;
import moa.core.TimingUtils;
import moa.evaluation.ClassificationPerformanceEvaluator;
import moa.evaluation.LearningCurve;
import moa.evaluation.LearningEvaluation;
import moa.options.ClassOption;
import moa.options.FileOption;
import moa.options.IntOption;
import moa.streams.InstanceStream;
import weka.core.Instance;
/**
* Task for evaluating a classifier on a stream by testing then training with
* each example in sequence.
*
* @author Richard Kirkby ([email protected])
* @version $Revision: 7 $
*/
public class EvaluateInterleavedTestThenTrain extends MainTask {
@Override
public String getPurposeString() {
return "Evaluates a classifier on a stream by testing then training with each example in sequence.";
}
private static final long serialVersionUID = 1L;
public ClassOption learnerOption = new ClassOption("learner", 'l',
"Classifier to train.", Classifier.class, "bayes.NaiveBayes");
public ClassOption streamOption = new ClassOption("stream", 's',
"Stream to learn from.", InstanceStream.class,
"generators.RandomTreeGenerator");
public IntOption randomSeedOption = new IntOption(
"instanceRandomSeed", 'r',
"Seed for random generation of instances.", 1);
public ClassOption evaluatorOption = new ClassOption("evaluator", 'e',
"Classification performance evaluation method.",
ClassificationPerformanceEvaluator.class,
"BasicClassificationPerformanceEvaluator");
public IntOption instanceLimitOption = new IntOption("instanceLimit", 'i',
"Maximum number of instances to test/train on (-1 = no limit).",
100000000, -1, Integer.MAX_VALUE);
public IntOption timeLimitOption = new IntOption("timeLimit", 't',
"Maximum number of seconds to test/train for (-1 = no limit).", -1,
-1, Integer.MAX_VALUE);
public IntOption sampleFrequencyOption = new IntOption("sampleFrequency",
'f',
"How many instances between samples of the learning performance.",
100000, 0, Integer.MAX_VALUE);
public IntOption memCheckFrequencyOption = new IntOption(
"memCheckFrequency", 'q',
"How many instances between memory bound checks.", 100000, 0,
Integer.MAX_VALUE);
public FileOption dumpFileOption = new FileOption("dumpFile", 'd',
"File to append intermediate csv reslts to.", null, "csv", true);
@Override
public Class> getTaskResultType() {
return LearningCurve.class;
}
@Override
protected Object doMainTask(TaskMonitor monitor, ObjectRepository repository) {
String learnerString = this.learnerOption.getValueAsCLIString();
String streamString = this.streamOption.getValueAsCLIString();
//this.learnerOption.setValueViaCLIString(this.learnerOption.getValueAsCLIString() + " -r " +this.randomSeedOption);
this.streamOption.setValueViaCLIString(streamString + " -i " + this.randomSeedOption.getValueAsCLIString());
Classifier learner = (Classifier) getPreparedClassOption(this.learnerOption);
if (learner.isRandomizable()) {
learner.setRandomSeed(this.randomSeedOption.getValue());
learner.resetLearning();
}
InstanceStream stream = (InstanceStream) getPreparedClassOption(this.streamOption);
ClassificationPerformanceEvaluator evaluator = (ClassificationPerformanceEvaluator) getPreparedClassOption(this.evaluatorOption);
learner.setModelContext(stream.getHeader());
int maxInstances = this.instanceLimitOption.getValue();
long instancesProcessed = 0;
int maxSeconds = this.timeLimitOption.getValue();
int secondsElapsed = 0;
monitor.setCurrentActivity("Evaluating learner...", -1.0);
LearningCurve learningCurve = new LearningCurve(
"learning evaluation instances");
File dumpFile = this.dumpFileOption.getFile();
PrintStream immediateResultStream = null;
if (dumpFile != null) {
try {
if (dumpFile.exists()) {
immediateResultStream = new PrintStream(
new FileOutputStream(dumpFile, true), true);
} else {
immediateResultStream = new PrintStream(
new FileOutputStream(dumpFile), true);
}
} catch (Exception ex) {
throw new RuntimeException(
"Unable to open immediate result file: " + dumpFile, ex);
}
}
boolean firstDump = true;
boolean preciseCPUTiming = TimingUtils.enablePreciseTiming();
long evaluateStartTime = TimingUtils.getNanoCPUTimeOfCurrentThread();
long lastEvaluateStartTime = evaluateStartTime;
double RAMHours = 0.0;
while (stream.hasMoreInstances()
&& ((maxInstances < 0) || (instancesProcessed < maxInstances))
&& ((maxSeconds < 0) || (secondsElapsed < maxSeconds))) {
Instance trainInst = stream.nextInstance();
Instance testInst = (Instance) trainInst.copy();
int trueClass = (int) trainInst.classValue();
//testInst.setClassMissing();
double[] prediction = learner.getVotesForInstance(testInst);
//evaluator.addClassificationAttempt(trueClass, prediction, testInst
// .weight());
evaluator.addResult(testInst, prediction);
learner.trainOnInstance(trainInst);
instancesProcessed++;
if (instancesProcessed % this.sampleFrequencyOption.getValue() == 0
|| stream.hasMoreInstances() == false) {
long evaluateTime = TimingUtils.getNanoCPUTimeOfCurrentThread();
double time = TimingUtils.nanoTimeToSeconds(evaluateTime - evaluateStartTime);
double timeIncrement = TimingUtils.nanoTimeToSeconds(evaluateTime - lastEvaluateStartTime);
double RAMHoursIncrement = learner.measureByteSize() / (1024.0 * 1024.0 * 1024.0); //GBs
RAMHoursIncrement *= (timeIncrement / 3600.0); //Hours
RAMHours += RAMHoursIncrement;
lastEvaluateStartTime = evaluateTime;
learningCurve.insertEntry(new LearningEvaluation(
new Measurement[]{
new Measurement(
"learning evaluation instances",
instancesProcessed),
new Measurement(
"evaluation time ("
+ (preciseCPUTiming ? "cpu "
: "") + "seconds)",
time),
new Measurement(
"model cost (RAM-Hours)",
RAMHours)
},
evaluator, learner));
if (immediateResultStream != null) {
if (firstDump) {
immediateResultStream.print("Learner,stream,randomSeed,");
immediateResultStream.println(learningCurve.headerToString());
firstDump = false;
}
immediateResultStream.print(learnerString + "," + streamString + "," + this.randomSeedOption.getValueAsCLIString() + ",");
immediateResultStream.println(learningCurve.entryToString(learningCurve.numEntries() - 1));
immediateResultStream.flush();
}
}
if (instancesProcessed % INSTANCES_BETWEEN_MONITOR_UPDATES == 0) {
if (monitor.taskShouldAbort()) {
return null;
}
long estimatedRemainingInstances = stream.estimatedRemainingInstances();
if (maxInstances > 0) {
long maxRemaining = maxInstances - instancesProcessed;
if ((estimatedRemainingInstances < 0)
|| (maxRemaining < estimatedRemainingInstances)) {
estimatedRemainingInstances = maxRemaining;
}
}
monitor.setCurrentActivityFractionComplete(estimatedRemainingInstances < 0 ? -1.0
: (double) instancesProcessed
/ (double) (instancesProcessed + estimatedRemainingInstances));
if (monitor.resultPreviewRequested()) {
monitor.setLatestResultPreview(learningCurve.copy());
}
secondsElapsed = (int) TimingUtils.nanoTimeToSeconds(TimingUtils.getNanoCPUTimeOfCurrentThread()
- evaluateStartTime);
}
}
if (immediateResultStream != null) {
immediateResultStream.close();
}
return learningCurve;
}
}
© 2015 - 2025 Weber Informatics LLC | Privacy Policy