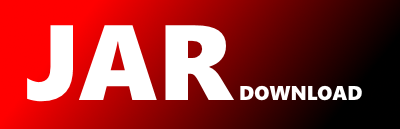
moa.classifiers.core.statisticaltests.KNN Maven / Gradle / Ivy
Go to download
Show more of this group Show more artifacts with this name
Show all versions of moa Show documentation
Show all versions of moa Show documentation
Massive On-line Analysis is an environment for massive data mining. MOA
provides a framework for data stream mining and includes tools for evaluation
and a collection of machine learning algorithms. Related to the WEKA project,
also written in Java, while scaling to more demanding problems.
/*
* KNN.java
* Copyright (C) 2017 Instituto Federal de Pernambuco
* @author Paulo Gonçalves ([email protected])
*
* This program is free software; you can redistribute it and/or modify
* it under the terms of the GNU General Public License as published by
* the Free Software Foundation; either version 3 of the License, or
* (at your option) any later version.
*
* This program is distributed in the hope that it will be useful,
* but WITHOUT ANY WARRANTY; without even the implied warranty of
* MERCHANTABILITY or FITNESS FOR A PARTICULAR PURPOSE. See the
* GNU General Public License for more details.
*
* You should have received a copy of the GNU General Public License
* along with this program. If not, see .
*
*/
package moa.classifiers.core.statisticaltests;
import com.github.javacliparser.IntOption;
import com.yahoo.labs.samoa.instances.Instance;
import java.util.Arrays;
import java.util.Comparator;
import java.util.List;
import java.util.PriorityQueue;
import moa.core.ObjectRepository;
import moa.options.AbstractOptionHandler;
import moa.tasks.TaskMonitor;
/**
* Implements the multivariate non-parametric KNN statistical test.
*
* @author Paulo Goncalves
*
*/
public class KNN extends AbstractOptionHandler implements StatisticalTest {
private List sample1i;
private List sample2i;
public IntOption kValueOption = new IntOption("kValue", 'k',
"K value of the K nearest neighbours algorithm.", 5, 1,
Integer.MAX_VALUE);
private double[] compute(double[][] set, int d, int n1, int n2) throws InterruptedException {
double n = n1 + n2;
Arrays.fill(set[d], 0, n1, 1.0);
Arrays.fill(set[d], n1, n1 + n2, 2.0);
int[] counts = this.knn(set, n1 + n2, d, this.kValueOption.getValue());
double Tk = 0;
for (int i = 0; i < counts.length; i++) {
Tk += counts[i];
}
Tk /= (n * this.kValueOption.getValue());
double V = (n1 - 1) * (n2 - 1) / ((n - 1) * (n - 1)) + 4
* ((n1 - 1) * (n1 - 2) / ((n - 1) * (n - 2)))
* ((n2 - 1) * (n2 - 2) / ((n - 1) * (n - 2)));
double Z = Math.sqrt(n * this.kValueOption.getValue())
* (Tk - (n1 - 1) * (n1 - 2) / ((n - 1) * (n - 2)) - (n2 - 1)
* (n2 - 2) / ((n - 1) * (n - 2))) / Math.sqrt(V);
double P = this.pnorm(Z, 0, 1, false, false);
return new double[]{Tk, Z, P};
}
private double[] attributeToDoubleArray(List list, int attIndex) {
double[] ret = new double[list.size()];
for (int i = 0; i < list.size(); i++) {
ret[i] = list.get(i).value(attIndex);
}
return ret;
}
public double[] mtsknn(List x, List y) throws InterruptedException {
if (x.get(0).numAttributes() != y.get(0).numAttributes()) {
System.out.println("The dimensions of two samples must match!!!");
return null;
}
int d = x.get(0).numAttributes() - 1;
int n1 = x.size();
int n2 = y.size();
double[][] set = new double[d + 1][n1 + n2];
for (int i = 0; i < d; i++) {
double[] t1 = this.attributeToDoubleArray(x, i);
double[] t2 = this.attributeToDoubleArray(y, i);
System.arraycopy(t1, 0, set[i], 0, t1.length);
System.arraycopy(t2, 0, set[i], t1.length, t2.length);
}
return this.compute(set, d, n1, n2);
}
private double pnorm(double x, double mu, double sigma, boolean lower_tail,
boolean log_p) {
double p;
if (Double.isNaN(x) || Double.isNaN(mu) || Double.isNaN(sigma)) {
return x + mu + sigma;
}
if (Double.isInfinite(x) && mu == x) {
return Double.NaN;/* x-mu is NaN */
}
if (sigma <= 0) {
// if(sigma < 0) ML_ERR_return_NAN;
if (x < mu) {
R_DT(lower_tail, log_p);
}
}
p = (x - mu) / sigma;
if (Double.isInfinite(p)) {
if (x < mu) {
R_DT(lower_tail, log_p);
}
}
x = p;
double[] ret = this.pnorm_both(x, p, (lower_tail ? 0 : 1), log_p);
return (lower_tail ? ret[0] : ret[1]);
}
private class DIPair {
double e = 0;
int i = 0;
public DIPair(double e0, int i0) {
this.e = e0;
this.i = i0;
}
public double getE() {
return e;
}
public int getI() {
return i;
}
}
private class HigherComparator implements Comparator {
@Override
public int compare(DIPair o1, DIPair o2) {
return (o1.e > o2.e) ? -1 : ((o1.e == o2.e) ? 0 : 1);
}
}
// Calculando as distancias entre dois pontos para todos os atributos
private double dist(double[][] points, int v1, int v2, int d) {
double sum = 0;
for (int i = 0; i != d; ++i) {
// Do not use Math.pow! It is 8x slower than computing directly
sum += (points[i][v1] - points[i][v2])
* (points[i][v1] - points[i][v2]);
}
return sum;
}
/**
* Computes, for each instance, the number of the k nearest neighbors that
* are from the same sample.
*
* @param points Instances of both samples put together, with the last
* column having 1 for the first sample and 2 for the second sample.
* @param n Number of instances.
* @param d Number of attributes + 1 column.
* @param k K nearest neighbors.
* @return the number of the closest neighbors that are from the same
* sample.
* @throws InterruptedException
*/
private int[] knn(double[][] points, int n, int d, int k) throws InterruptedException {
int[] counts = new int[n];
int[] closest = new int[n * k];
// Percorrendo todos os atributos
for (int i = 0; i != n; ++i) {
if (Thread.interrupted()) {
// We've been interrupted: no more crunching.
throw new InterruptedException();
}
PriorityQueue q = new PriorityQueue(k,
new HigherComparator());
// Percorrendo os valores do atributo
for (int j = 0; j != n; ++j) {
if (i != j) {
DIPair dis = new DIPair(this.dist(points, i, j, d), j);
if (q.size() == k) {
if (dis.getE() < q.peek().getE()) {
q.add(dis);
q.poll();
}
} else {
q.add(dis);
}
}
}
// Armazenando as posicoes das k menores distancias em ordem
// crescente para cada instancia
for (int j = 0; j != k; ++j) {
closest[i * k + j] = q.poll().getI();
}
}
// Percorrendo todas as instancias
for (int i = 0; i != n; ++i) {
if (Thread.interrupted()) {
// We've been interrupted: no more crunching.
throw new InterruptedException();
}
// Percorrendo os k valores mais proximos
for (int j = 0; j != k; ++j) {
// Verificando se as instancias mais proximas sao da mesma
// amostra
if (points[d][closest[i * k + j]] == points[d][i]) {
counts[i] += 1;
}
}
}
return counts;
}
private double R_DT(boolean lower_tail, boolean log_p) {
return (lower_tail) ? ((log_p) ? Double.NEGATIVE_INFINITY : 0)
: ((log_p) ? 0 : 1);
}
private double[] pnorm_both(double x, double cum, int i_tail,
boolean log_p) {
double ccum = 0;
final double a[] = {2.2352520354606839287, 161.02823106855587881,
1067.6894854603709582, 18154.981253343561249,
0.065682337918207449113};
final double b[] = {47.20258190468824187, 976.09855173777669322,
10260.932208618978205, 45507.789335026729956};
final double c[] = {0.39894151208813466764, 8.8831497943883759412,
93.506656132177855979, 597.27027639480026226,
2494.5375852903726711, 6848.1904505362823326,
11602.651437647350124, 9842.7148383839780218,
1.0765576773720192317e-8};
final double d[] = {22.266688044328115691, 235.38790178262499861,
1519.377599407554805, 6485.558298266760755,
18615.571640885098091, 34900.952721145977266,
38912.003286093271411, 19685.429676859990727};
final double p[] = {0.21589853405795699, 0.1274011611602473639,
0.022235277870649807, 0.001421619193227893466,
2.9112874951168792e-5, 0.02307344176494017303};
final double q[] = {1.28426009614491121, 0.468238212480865118,
0.0659881378689285515, 0.00378239633202758244,
7.29751555083966205e-5};
final double M_SQRT_32 = 5.656854249492380195206754896838;
final double M_1_SQRT_2PI = 0.398942280401432677939946059934;
double xden, xnum, temp, eps, xsq, y;
double min = Double.MIN_VALUE;
int i;
boolean lower, upper;
if (Double.isNaN(x)) {
cum = ccum = x;
return new double[]{cum, ccum};
}
eps = 1E-9 * 0.5;
lower = i_tail != 1;
upper = i_tail != 0;
y = Math.abs(x);
if (y <= 0.67448975) {
/*
* qnorm(3/4) = .6744.... -- earlier had
* 0.66291
*/
if (y > eps) {
xsq = x * x;
xnum = a[4] * xsq;
xden = xsq;
for (i = 0; i < 3; ++i) {
xnum = (xnum + a[i]) * xsq;
xden = (xden + b[i]) * xsq;
}
} else {
xnum = xden = 0.0;
}
temp = x * (xnum + a[3]) / (xden + b[3]);
if (lower) {
cum = 0.5 + temp;
}
if (upper) {
ccum = 0.5 - temp;
}
if (log_p) {
if (lower) {
cum = Math.log(cum);
}
if (upper) {
ccum = Math.log(ccum);
}
}
} else if (y <= M_SQRT_32) {
xnum = c[8] * y;
xden = y;
for (i = 0; i < 7; ++i) {
xnum = (xnum + c[i]) * y;
xden = (xden + d[i]) * y;
}
temp = (xnum + c[7]) / (xden + d[7]);
double[] retorno = do_del(y, log_p, cum, ccum, lower, x, temp,
upper);
retorno = swap_tail(x, temp, retorno[0], lower, retorno[1]);
cum = retorno[0];
ccum = retorno[1];
} else if (log_p || (lower && -37.5193 < x && x < 8.2924)
|| (upper && -8.2924 < x && x < 37.5193)) {
/* Evaluate pnorm for x in (-37.5, -5.657) union (5.657, 37.5) */
xsq = 1.0 / (x * x);
xnum = p[5] * xsq;
xden = xsq;
for (i = 0; i < 4; ++i) {
xnum = (xnum + p[i]) * xsq;
xden = (xden + q[i]) * xsq;
}
temp = xsq * (xnum + p[4]) / (xden + q[4]);
temp = (M_1_SQRT_2PI - temp) / y;
double[] retorno = do_del(x, log_p, cum, ccum, lower, x, temp,
upper);
retorno = swap_tail(x, temp, retorno[0], lower, retorno[1]);
cum = retorno[0];
ccum = retorno[1];
} else /* no log_p , large x such that probs are 0 or 1 */ if (x > 0) {
cum = 1.;
ccum = 0.;
} else {
cum = 0.;
ccum = 1.;
}
/* do not return "denormalized" -- we do in R */
if (log_p) {
if (cum > -min) {
cum = -0.;
}
if (ccum > -min) {
ccum = -0.;
}
} else {
if (cum < min) {
cum = 0.;
}
if (ccum < min) {
ccum = 0.;
}
}
return new double[]{cum, ccum};
}
private double[] do_del(double X, boolean log_p, double cum,
double ccum, boolean lower, double x, double temp, boolean upper) {
final int SIXTEN = 16;
double xsq = Math.ceil(X * SIXTEN) / SIXTEN;
double del = (X - xsq) * (X + xsq);
if (log_p) {
cum = (-xsq * xsq * 0.5) + (-del * 0.5) + Math.log(temp);
if ((lower && x > 0.) || (upper && x <= 0.)) {
ccum = Math.log1p(-Math.exp(-xsq * xsq * 0.5)
* Math.exp(-del * 0.5) * temp);
}
} else {
cum = Math.exp(-xsq * xsq * 0.5) * Math.exp(-del * 0.5) * temp;
ccum = 1.0 - cum;
}
return new double[]{cum, ccum};
}
private double[] swap_tail(double x, double temp, double cum,
boolean lower, double ccum) {
if (x > 0.) {/* swap ccum <--> cum */
temp = cum;
if (lower) {
cum = ccum;
}
ccum = temp;
}
return new double[]{cum, ccum};
}
@Override
public double test(List x, List y) {
try {
return this.mtsknn(x, y)[2];
} catch (InterruptedException ie) {
return 0.0;
}
}
@Override
public void getDescription(StringBuilder sb, int indent) {
// TODO Auto-generated method stub
}
@Override
protected void prepareForUseImpl(TaskMonitor monitor,
ObjectRepository repository) {
// TODO Auto-generated method stub
}
@Override
public Double call() throws Exception {
return this.test(sample1i, sample2i);
}
@Override
public void set(List x, List y) {
this.sample1i = x;
this.sample2i = y;
}
public static void main(String[] args) throws Exception {
List x = Cramer.fileToInstances("c:\\Users\\Paulo\\Documents\\test1-x.arff");
List y = Cramer.fileToInstances("c:\\Users\\Paulo\\Documents\\test1-y.arff");
KNN c = new KNN();
double[] ct = c.mtsknn(x, y);
System.out.println("p Value [Resultado esperado: 0.09866699171730517] [Resultado obtido..: " + ct[2] + "]");
System.out.println("Critical value [Resultado esperado: 0.521] [Resultado obtido: " + ct[0] + "]");
System.out.println("Statistic [Resultado esperado: 1.2891844104764096] [Resultado obtido: " + ct[1] + "]");
}
}
© 2015 - 2025 Weber Informatics LLC | Privacy Policy