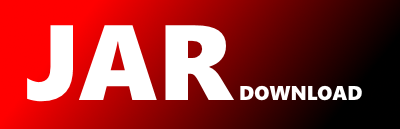
moa.classifiers.functions.SGD Maven / Gradle / Ivy
Go to download
Show more of this group Show more artifacts with this name
Show all versions of moa Show documentation
Show all versions of moa Show documentation
Massive On-line Analysis is an environment for massive data mining. MOA
provides a framework for data stream mining and includes tools for evaluation
and a collection of machine learning algorithms. Related to the WEKA project,
also written in Java, while scaling to more demanding problems.
/*
* SGD.java
* Copyright (C) 2009 University of Waikato, Hamilton, New Zealand
* @author Eibe Frank (eibe{[at]}cs{[dot]}waikato{[dot]}ac{[dot]}nz)
* @author Mark Hall (mhall{[at]}pentaho{[dot]}com)
*
* This program is free software; you can redistribute it and/or modify
* it under the terms of the GNU General Public License as published by
* the Free Software Foundation; either version 3 of the License, or
* (at your option) any later version.
*
* This program is distributed in the hope that it will be useful,
* but WITHOUT ANY WARRANTY; without even the implied warranty of
* MERCHANTABILITY or FITNESS FOR A PARTICULAR PURPOSE. See the
* GNU General Public License for more details.
*
* You should have received a copy of the GNU General Public License
* along with this program. If not, see .
*
*/
/*
* SGD.java
* Copyright (C) 2009 University of Waikato, Hamilton, New Zealand
*
*/
package moa.classifiers.functions;
import moa.classifiers.AbstractClassifier;
import moa.classifiers.MultiClassClassifier;
import moa.core.DoubleVector;
import moa.core.Measurement;
import moa.core.StringUtils;
import com.github.javacliparser.FloatOption;
import com.github.javacliparser.MultiChoiceOption;
import moa.classifiers.Regressor;
import com.yahoo.labs.samoa.instances.Instance;
import moa.core.Utils;
/**
* Implements stochastic gradient descent for learning various linear models (binary class SVM, binary class logistic regression and linear regression).
*
*
*/
public class SGD extends AbstractClassifier implements MultiClassClassifier, Regressor{
/** For serialization */
private static final long serialVersionUID = -3732968666673530290L;
@Override
public String getPurposeString() {
return "AStochastic gradient descent for learning various linear models (binary class SVM, binary class logistic regression and linear regression).";
}
/** The regularization parameter */
protected double m_lambda = 0.0001;
public FloatOption lambdaRegularizationOption = new FloatOption("lambdaRegularization",
'l', "Lambda regularization parameter .",
0.0001, 0.00, Integer.MAX_VALUE);
/** The learning rate */
protected double m_learningRate = 0.01;
public FloatOption learningRateOption = new FloatOption("learningRate",
'r', "Learning rate parameter.",
0.0001, 0.00, Integer.MAX_VALUE);
/** Stores the weights (+ bias in the last element) */
protected DoubleVector m_weights;
protected double m_bias;
/** Holds the current iteration number */
protected double m_t;
/** The number of training instances */
protected double m_numInstances;
protected static final int HINGE = 0;
protected static final int LOGLOSS = 1;
protected static final int SQUAREDLOSS = 2;
/** The current loss function to minimize */
protected int m_loss = HINGE;
public MultiChoiceOption lossFunctionOption = new MultiChoiceOption(
"lossFunction", 'o', "The loss function to use.", new String[]{
"HINGE", "LOGLOSS", "SQUAREDLOSS"}, new String[]{
"Hinge loss (SVM)",
"Log loss (logistic regression)",
"Squared loss (regression)"}, 0);
/**
* Set the value of lambda to use
*
* @param lambda the value of lambda to use
*/
public void setLambda(double lambda) {
m_lambda = lambda;
}
/**
* Get the current value of lambda
*
* @return the current value of lambda
*/
public double getLambda() {
return m_lambda;
}
/**
* Set the loss function to use.
*
* @param function the loss function to use.
*/
public void setLossFunction(int function) {
m_loss = function;
}
/**
* Get the current loss function.
*
* @return the current loss function.
*/
public int getLossFunction() {
return m_loss;
}
/**
* Set the learning rate.
*
* @param lr the learning rate to use.
*/
public void setLearningRate(double lr) {
m_learningRate = lr;
}
/**
* Get the learning rate.
*
* @return the learning rate
*/
public double getLearningRate() {
return m_learningRate;
}
/**
* Reset the classifier.
*/
public void reset() {
m_t = 1;
m_weights = null;
m_bias = 0.0;
}
protected double dloss(double z) {
if (m_loss == HINGE) {
return (z < 1) ? 1 : 0;
}
if (m_loss == LOGLOSS) {
// log loss
if (z < 0) {
return 1.0 / (Math.exp(z) + 1.0);
} else {
double t = Math.exp(-z);
return t / (t + 1);
}
}
// squared loss
return z;
}
protected static double dotProd(Instance inst1, DoubleVector weights, int classIndex) {
double result = 0;
int n1 = inst1.numValues();
int n2 = weights.numValues();
for (int p1 = 0, p2 = 0; p1 < n1 && p2 < n2;) {
int ind1 = inst1.index(p1);
int ind2 = p2;
if (ind1 == ind2) {
if (ind1 != classIndex && !inst1.isMissingSparse(p1)) {
result += inst1.valueSparse(p1) * weights.getValue(p2);
}
p1++;
p2++;
} else if (ind1 > ind2) {
p2++;
} else {
p1++;
}
}
return (result);
}
@Override
public void resetLearningImpl() {
reset();
setLambda(this.lambdaRegularizationOption.getValue());
setLearningRate(this.learningRateOption.getValue());
setLossFunction(this.lossFunctionOption.getChosenIndex());
}
/**
* Trains the classifier with the given instance.
*
* @param instance the new training instance to include in the model
*/
@Override
public void trainOnInstanceImpl(Instance instance) {
if (m_weights == null) {
m_weights = new DoubleVector();
m_bias = 0.0;
}
if (!instance.classIsMissing()) {
double wx = dotProd(instance, m_weights, instance.classIndex());
double y;
double z;
if (instance.classAttribute().isNominal()) {
y = (instance.classValue() == 0) ? -1 : 1;
z = y * (wx + m_bias);
} else {
y = instance.classValue();
z = y - (wx + m_bias);
y = 1;
}
// Compute multiplier for weight decay
double multiplier = 1.0;
if (m_numInstances == 0) {
multiplier = 1.0 - (m_learningRate * m_lambda) / m_t;
} else {
multiplier = 1.0 - (m_learningRate * m_lambda) / m_numInstances;
}
for (int i = 0; i < m_weights.numValues(); i++) {
m_weights.setValue(i,m_weights.getValue (i) * multiplier);
}
// Only need to do the following if the loss is non-zero
if (m_loss != HINGE || (z < 1)) {
// Compute Factor for updates
double factor = m_learningRate * y * dloss(z);
// Update coefficients for attributes
int n1 = instance.numValues();
for (int p1 = 0; p1 < n1; p1++) {
int indS = instance.index(p1);
if (indS != instance.classIndex() && !instance.isMissingSparse(p1)) {
m_weights.addToValue(indS, factor * instance.valueSparse(p1));
}
}
// update the bias
m_bias += factor;
}
m_t++;
}
}
/**
* Calculates the class membership probabilities for the given test
* instance.
*
* @param instance the instance to be classified
* @return predicted class probability distribution
*/
@Override
public double[] getVotesForInstance(Instance inst) {
if (m_weights == null) {
return new double[inst.numClasses()];
}
double[] result = (inst.classAttribute().isNominal())
? new double[2]
: new double[1];
double wx = dotProd(inst, m_weights, inst.classIndex());// * m_wScale;
double z = (wx + m_bias);
if (inst.classAttribute().isNumeric()) {
result[0] = z;
return result;
}
if (z <= 0) {
// z = 0;
if (m_loss == LOGLOSS) {
result[0] = 1.0 / (1.0 + Math.exp(z));
result[1] = 1.0 - result[0];
} else {
result[0] = 1;
}
} else {
if (m_loss == LOGLOSS) {
result[1] = 1.0 / (1.0 + Math.exp(-z));
result[0] = 1.0 - result[1];
} else {
result[1] = 1;
}
}
return result;
}
@Override
public void getModelDescription(StringBuilder result, int indent) {
StringUtils.appendIndented(result, indent, toString());
StringUtils.appendNewline(result);
}
/**
* Prints out the classifier.
*
* @return a description of the classifier as a string
*/
public String toString() {
if (m_weights == null) {
return "SGD: No model built yet.\n";
}
StringBuffer buff = new StringBuffer();
buff.append("Loss function: ");
if (m_loss == HINGE) {
buff.append("Hinge loss (SVM)\n\n");
} else if (m_loss == LOGLOSS) {
buff.append("Log loss (logistic regression)\n\n");
} else {
buff.append("Squared loss (linear regression)\n\n");
}
// buff.append(m_data.classAttribute().name() + " = \n\n");
int printed = 0;
for (int i = 0; i < m_weights.numValues(); i++) {
// if (i != m_data.classIndex()) {
if (printed > 0) {
buff.append(" + ");
} else {
buff.append(" ");
}
buff.append(Utils.doubleToString(m_weights.getValue(i), 12, 4) + " "
// + m_data.attribute(i).name()
+ "\n");
printed++;
//}
}
if (m_bias > 0) {
buff.append(" + " + Utils.doubleToString(m_bias, 12, 4));
} else {
buff.append(" - " + Utils.doubleToString(-m_bias, 12, 4));
}
return buff.toString();
}
@Override
protected Measurement[] getModelMeasurementsImpl() {
return null;
}
@Override
public boolean isRandomizable() {
return false;
}
}
© 2015 - 2025 Weber Informatics LLC | Privacy Policy