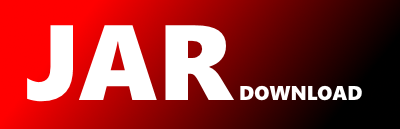
moa.classifiers.lazy.neighboursearch.NormalizableDistance Maven / Gradle / Ivy
Go to download
Massive On-line Analysis is an environment for massive data mining. MOA
provides a framework for data stream mining and includes tools for evaluation
and a collection of machine learning algorithms. Related to the WEKA project,
also written in Java, while scaling to more demanding problems.
/*
* This program is free software: you can redistribute it and/or modify
* it under the terms of the GNU General Public License as published by
* the Free Software Foundation, either version 3 of the License, or
* (at your option) any later version.
*
* This program is distributed in the hope that it will be useful,
* but WITHOUT ANY WARRANTY; without even the implied warranty of
* MERCHANTABILITY or FITNESS FOR A PARTICULAR PURPOSE. See the
* GNU General Public License for more details.
*
* You should have received a copy of the GNU General Public License
* along with this program. If not, see .
*/
/*
* NormalizableDistance.java
* Copyright (C) 2007-2012 University of Waikato, Hamilton, New Zealand
*
*/
package moa.classifiers.lazy.neighboursearch;
import com.yahoo.labs.samoa.instances.Attribute;
import com.yahoo.labs.samoa.instances.Instance;
import com.yahoo.labs.samoa.instances.Instances;
/**
* Represents the abstract ancestor for normalizable distance functions, like
* Euclidean or Manhattan distance.
*
* @author Fracpete (fracpete at waikato dot ac dot nz)
* @author Gabi Schmidberger ([email protected]) -- original code from weka.core.EuclideanDistance
* @author Ashraf M. Kibriya ([email protected]) -- original code from weka.core.EuclideanDistance
* @version $Revision: 8034 $
*/
public abstract class NormalizableDistance
implements DistanceFunction {
/** Index in ranges for MIN. */
public static final int R_MIN = 0;
/** Index in ranges for MAX. */
public static final int R_MAX = 1;
/** Index in ranges for WIDTH. */
public static final int R_WIDTH = 2;
/** the instances used internally. */
protected Instances m_Data = null;
/** True if normalization is turned off (default false).*/
protected boolean m_DontNormalize = false;
/** The range of the attributes. */
protected double[][] m_Ranges;
/** The range of attributes to use for calculating the distance. */
// protected Range m_AttributeIndices = new Range("first-last");
/** The boolean flags, whether an attribute will be used or not. */
protected boolean[] m_ActiveIndices;
/** Whether all the necessary preparations have been done. */
protected boolean m_Validated;
/**
* Invalidates the distance function, Instances must be still set.
*/
public NormalizableDistance() {
invalidate();
}
/**
* Initializes the distance function and automatically initializes the
* ranges.
*
* @param data the instances the distance function should work on
*/
public NormalizableDistance(Instances data) {
setInstances(data);
}
/**
* Returns a string describing this object.
*
* @return a description of the evaluator suitable for
* displaying in the explorer/experimenter gui
*/
public abstract String globalInfo();
/**
* Returns the tip text for this property.
*
* @return tip text for this property suitable for
* displaying in the explorer/experimenter gui
*/
public String dontNormalizeTipText() {
return "Whether if the normalization of attributes should be turned off " +
"for distance calculation (Default: false i.e. attribute values " +
"are normalized). ";
}
/**
* Sets whether if the attribute values are to be normalized in distance
* calculation.
*
* @param dontNormalize if true the values are not normalized
*/
public void setDontNormalize(boolean dontNormalize) {
m_DontNormalize = dontNormalize;
invalidate();
}
/**
* Gets whether if the attribute values are to be normazlied in distance
* calculation. (default false i.e. attribute values are normalized.)
*
* @return false if values get normalized
*/
public boolean getDontNormalize() {
return m_DontNormalize;
}
/**
* Returns the tip text for this property.
*
* @return tip text for this property suitable for
* displaying in the explorer/experimenter gui
*/
public String attributeIndicesTipText() {
return
"Specify range of attributes to act on. "
+ "This is a comma separated list of attribute indices, with "
+ "\"first\" and \"last\" valid values. Specify an inclusive "
+ "range with \"-\". E.g: \"first-3,5,6-10,last\".";
}
/**
* Sets the range of attributes to use in the calculation of the distance.
* The indices start from 1, 'first' and 'last' are valid as well.
* E.g.: first-3,5,6-last
*
* @param value the new attribute index range
*/
public void setAttributeIndices(String value) {
//m_AttributeIndices.setRanges(value);
invalidate();
}
/**
* Gets the range of attributes used in the calculation of the distance.
*
* @return the attribute index range
*/
public String getAttributeIndices() {
return null; //m_AttributeIndices.getRanges();
}
/**
* Returns the tip text for this property.
*
* @return tip text for this property suitable for
* displaying in the explorer/experimenter gui
*/
public String invertSelectionTipText() {
return
"Set attribute selection mode. If false, only selected "
+ "attributes in the range will be used in the distance calculation; if "
+ "true, only non-selected attributes will be used for the calculation.";
}
/**
* Sets whether the matching sense of attribute indices is inverted or not.
*
* @param value if true the matching sense is inverted
*/
public void setInvertSelection(boolean value) {
//m_AttributeIndices.setInvert(value);
invalidate();
}
/**
* Gets whether the matching sense of attribute indices is inverted or not.
*
* @return true if the matching sense is inverted
*/
public boolean getInvertSelection() {
return false; //m_AttributeIndices.getInvert();
}
/**
* invalidates all initializations.
*/
protected void invalidate() {
m_Validated = false;
}
/**
* performs the initializations if necessary.
*/
protected void validate() {
if (!m_Validated) {
initialize();
m_Validated = true;
}
}
/**
* initializes the ranges and the attributes being used.
*/
protected void initialize() {
initializeAttributeIndices();
initializeRanges();
}
/**
* initializes the attribute indices.
*/
protected void initializeAttributeIndices() {
//m_AttributeIndices.setUpper(m_Data.numAttributes() - 1);
m_ActiveIndices = new boolean[m_Data.numAttributes()];
for (int i = 0; i < m_ActiveIndices.length; i++)
m_ActiveIndices[i] = true; //m_AttributeIndices.isInRange(i);
}
/**
* Sets the instances.
*
* @param insts the instances to use
*/
public void setInstances(Instances insts) {
m_Data = insts;
invalidate();
}
/**
* returns the instances currently set.
*
* @return the current instances
*/
public Instances getInstances() {
return m_Data;
}
/**
* Does nothing, derived classes may override it though.
*
* @param distances the distances to post-process
*/
public void postProcessDistances(double[] distances) {
}
/**
* Update the distance function (if necessary) for the newly added instance.
*
* @param ins the instance to add
*/
public void update(Instance ins) {
validate();
m_Ranges = updateRanges(ins, m_Ranges);
}
/**
* Calculates the distance between two instances.
*
* @param first the first instance
* @param second the second instance
* @return the distance between the two given instances
*/
public double distance(Instance first, Instance second) {
return distance(first, second, Double.POSITIVE_INFINITY);
}
/**
* Calculates the distance between two instances. Offers speed up (if the
* distance function class in use supports it) in nearest neighbour search by
* taking into account the cutOff or maximum distance. Depending on the
* distance function class, post processing of the distances by
* postProcessDistances(double []) may be required if this function is used.
*
* @param first the first instance
* @param second the second instance
* @param cutOffValue If the distance being calculated becomes larger than
* cutOffValue then the rest of the calculation is
* discarded.
* @return the distance between the two given instances or
* Double.POSITIVE_INFINITY if the distance being
* calculated becomes larger than cutOffValue.
*/
public double distance(Instance first, Instance second, double cutOffValue) {
double distance = 0;
int firstI, secondI;
int firstNumValues = first.numValues();
int secondNumValues = second.numValues();
int numAttributes = m_Data.numAttributes();
int classIndex = m_Data.classIndex();
validate();
for (int p1 = 0, p2 = 0; p1 < firstNumValues || p2 < secondNumValues; ) {
if (p1 >= firstNumValues)
firstI = numAttributes;
else
firstI = first.index(p1);
if (p2 >= secondNumValues)
secondI = numAttributes;
else
secondI = second.index(p2);
if (firstI == classIndex) {
p1++;
continue;
}
if ((firstI < numAttributes) && !m_ActiveIndices[firstI]) {
p1++;
continue;
}
if (secondI == classIndex) {
p2++;
continue;
}
if ((secondI < numAttributes) && !m_ActiveIndices[secondI]) {
p2++;
continue;
}
double diff;
if (firstI == secondI) {
diff = difference(firstI,
first.valueSparse(p1),
second.valueSparse(p2));
p1++;
p2++;
}
else if (firstI > secondI) {
diff = difference(secondI,
0, second.valueSparse(p2));
p2++;
}
else {
diff = difference(firstI,
first.valueSparse(p1), 0);
p1++;
}
distance = updateDistance(distance, diff);
if (distance > cutOffValue)
return Double.POSITIVE_INFINITY;
}
return distance;
}
/**
* Updates the current distance calculated so far with the new difference
* between two attributes. The difference between the attributes was
* calculated with the difference(int,double,double) method.
*
* @param currDist the current distance calculated so far
* @param diff the difference between two new attributes
* @return the update distance
* @see #difference(int, double, double)
*/
protected abstract double updateDistance(double currDist, double diff);
/**
* Normalizes a given value of a numeric attribute.
*
* @param x the value to be normalized
* @param i the attribute's index
* @return the normalized value
*/
protected double norm(double x, int i) {
if (Double.isNaN(m_Ranges[i][R_MIN]) || (m_Ranges[i][R_MAX] == m_Ranges[i][R_MIN]))
return 0;
else
return (x - m_Ranges[i][R_MIN]) / (m_Ranges[i][R_WIDTH]);
}
/**
* Computes the difference between two given attribute
* values.
*
* @param index the attribute index
* @param val1 the first value
* @param val2 the second value
* @return the difference
*/
protected double difference(int index, double val1, double val2) {
//switch (m_Data.attribute(index).type()) {
//case Attribute.NOMINAL:
if (m_Data.attribute(index).isNominal() == true){
if (isMissingValue(val1) ||
isMissingValue(val2) ||
((int) val1 != (int) val2)) {
return 1;
}
else {
return 0;
}
} else {
//case Attribute.NUMERIC:
if (isMissingValue(val1) ||
isMissingValue(val2)) {
if (isMissingValue(val1) &&
isMissingValue(val2)) {
if (!m_DontNormalize) //We are doing normalization
return 1;
else
return (m_Ranges[index][R_MAX] - m_Ranges[index][R_MIN]);
}
else {
double diff;
if (isMissingValue(val2)) {
diff = (!m_DontNormalize) ? norm(val1, index) : val1;
}
else {
diff = (!m_DontNormalize) ? norm(val2, index) : val2;
}
if (!m_DontNormalize && diff < 0.5) {
diff = 1.0 - diff;
}
else if (m_DontNormalize) {
if ((m_Ranges[index][R_MAX]-diff) > (diff-m_Ranges[index][R_MIN]))
return m_Ranges[index][R_MAX]-diff;
else
return diff-m_Ranges[index][R_MIN];
}
return diff;
}
}
else {
return (!m_DontNormalize) ?
(norm(val1, index) - norm(val2, index)) :
(val1 - val2);
}
//default:
// return 0;
}
}
/**
* Initializes the ranges using all instances of the dataset.
* Sets m_Ranges.
*
* @return the ranges
*/
public double[][] initializeRanges() {
if (m_Data == null) {
m_Ranges = null;
return m_Ranges;
}
int numAtt = m_Data.numAttributes();
double[][] ranges = new double [numAtt][3];
if (m_Data.numInstances() <= 0) {
initializeRangesEmpty(numAtt, ranges);
m_Ranges = ranges;
return m_Ranges;
}
else {
// initialize ranges using the first instance
updateRangesFirst(m_Data.instance(0), numAtt, ranges);
}
// update ranges, starting from the second
for (int i = 1; i < m_Data.numInstances(); i++)
updateRanges(m_Data.instance(i), numAtt, ranges);
m_Ranges = ranges;
return m_Ranges;
}
/**
* Used to initialize the ranges. For this the values of the first
* instance is used to save time.
* Sets low and high to the values of the first instance and
* width to zero.
*
* @param instance the new instance
* @param numAtt number of attributes in the model
* @param ranges low, high and width values for all attributes
*/
public void updateRangesFirst(Instance instance, int numAtt, double[][] ranges) {
for (int j = 0; j < numAtt; j++) {
if (!instance.isMissing(j)) {
ranges[j][R_MIN] = instance.value(j);
ranges[j][R_MAX] = instance.value(j);
ranges[j][R_WIDTH] = 0.0;
}
else { // if value was missing
ranges[j][R_MIN] = Double.POSITIVE_INFINITY;
ranges[j][R_MAX] = -Double.POSITIVE_INFINITY;
ranges[j][R_WIDTH] = Double.POSITIVE_INFINITY;
}
}
}
/**
* Updates the minimum and maximum and width values for all the attributes
* based on a new instance.
*
* @param instance the new instance
* @param numAtt number of attributes in the model
* @param ranges low, high and width values for all attributes
*/
public void updateRanges(Instance instance, int numAtt, double[][] ranges) {
// updateRangesFirst must have been called on ranges
for (int j = 0; j < numAtt; j++) {
double value = instance.value(j);
if (!instance.isMissing(j)) {
if (value < ranges[j][R_MIN]) {
ranges[j][R_MIN] = value;
ranges[j][R_WIDTH] = ranges[j][R_MAX] - ranges[j][R_MIN];
if (value > ranges[j][R_MAX]) { //if this is the first value that is
ranges[j][R_MAX] = value; //not missing. The,0
ranges[j][R_WIDTH] = ranges[j][R_MAX] - ranges[j][R_MIN];
}
}
else {
if (value > ranges[j][R_MAX]) {
ranges[j][R_MAX] = value;
ranges[j][R_WIDTH] = ranges[j][R_MAX] - ranges[j][R_MIN];
}
}
}
}
}
/**
* Used to initialize the ranges.
*
* @param numAtt number of attributes in the model
* @param ranges low, high and width values for all attributes
*/
public void initializeRangesEmpty(int numAtt, double[][] ranges) {
for (int j = 0; j < numAtt; j++) {
ranges[j][R_MIN] = Double.POSITIVE_INFINITY;
ranges[j][R_MAX] = -Double.POSITIVE_INFINITY;
ranges[j][R_WIDTH] = Double.POSITIVE_INFINITY;
}
}
/**
* Updates the ranges given a new instance.
*
* @param instance the new instance
* @param ranges low, high and width values for all attributes
* @return the updated ranges
*/
public double[][] updateRanges(Instance instance, double[][] ranges) {
// updateRangesFirst must have been called on ranges
for (int j = 0; j < ranges.length; j++) {
double value = instance.value(j);
if (!instance.isMissing(j)) {
if (value < ranges[j][R_MIN]) {
ranges[j][R_MIN] = value;
ranges[j][R_WIDTH] = ranges[j][R_MAX] - ranges[j][R_MIN];
} else {
if (instance.value(j) > ranges[j][R_MAX]) {
ranges[j][R_MAX] = value;
ranges[j][R_WIDTH] = ranges[j][R_MAX] - ranges[j][R_MIN];
}
}
}
}
return ranges;
}
/**
* Initializes the ranges of a subset of the instances of this dataset.
* Therefore m_Ranges is not set.
*
* @param instList list of indexes of the subset
* @return the ranges
* @throws Exception if something goes wrong
*/
public double[][] initializeRanges(int[] instList) throws Exception {
if (m_Data == null)
throw new Exception("No instances supplied.");
int numAtt = m_Data.numAttributes();
double[][] ranges = new double [numAtt][3];
if (m_Data.numInstances() <= 0) {
initializeRangesEmpty(numAtt, ranges);
return ranges;
}
else {
// initialize ranges using the first instance
updateRangesFirst(m_Data.instance(instList[0]), numAtt, ranges);
// update ranges, starting from the second
for (int i = 1; i < instList.length; i++) {
updateRanges(m_Data.instance(instList[i]), numAtt, ranges);
}
}
return ranges;
}
/**
* Initializes the ranges of a subset of the instances of this dataset.
* Therefore m_Ranges is not set.
* The caller of this method should ensure that the supplied start and end
* indices are valid (start <= end, end<instList.length etc) and
* correct.
*
* @param instList list of indexes of the instances
* @param startIdx start index of the subset of instances in the indices array
* @param endIdx end index of the subset of instances in the indices array
* @return the ranges
* @throws Exception if something goes wrong
*/
public double[][] initializeRanges(int[] instList, int startIdx, int endIdx) throws Exception {
if (m_Data == null)
throw new Exception("No instances supplied.");
int numAtt = m_Data.numAttributes();
double[][] ranges = new double [numAtt][3];
if (m_Data.numInstances() <= 0) {
initializeRangesEmpty(numAtt, ranges);
return ranges;
}
else {
// initialize ranges using the first instance
updateRangesFirst(m_Data.instance(instList[startIdx]), numAtt, ranges);
// update ranges, starting from the second
for (int i = startIdx+1; i <= endIdx; i++) {
updateRanges(m_Data.instance(instList[i]), numAtt, ranges);
}
}
return ranges;
}
/**
* Update the ranges if a new instance comes.
*
* @param instance the new instance
*/
public void updateRanges(Instance instance) {
validate();
m_Ranges = updateRanges(instance, m_Ranges);
}
/**
* Test if an instance is within the given ranges.
*
* @param instance the instance
* @param ranges the ranges the instance is tested to be in
* @return true if instance is within the ranges
*/
public boolean inRanges(Instance instance, double[][] ranges) {
boolean isIn = true;
// updateRangesFirst must have been called on ranges
for (int j = 0; isIn && (j < ranges.length); j++) {
if (!instance.isMissing(j)) {
double value = instance.value(j);
isIn = value <= ranges[j][R_MAX];
if (isIn) isIn = value >= ranges[j][R_MIN];
}
}
return isIn;
}
/**
* Check if ranges are set.
*
* @return true if ranges are set
*/
public boolean rangesSet() {
return (m_Ranges != null);
}
/**
* Method to get the ranges.
*
* @return the ranges
* @throws Exception if no randes are set yet
*/
public double[][] getRanges() throws Exception {
validate();
if (m_Ranges == null)
throw new Exception("Ranges not yet set.");
return m_Ranges;
}
/**
* Returns an empty string.
*
* @return an empty string
*/
public String toString() {
return "";
}
/**
* Tests if the given value codes "missing".
*
* @param val the value to be tested
* @return true if val codes "missing"
*/
public static boolean isMissingValue(double val) {
return Double.isNaN(val);
}
}
© 2015 - 2025 Weber Informatics LLC | Privacy Policy